Statistics: Sex difference analyses under scrutiny
Scientific research requires the use of appropriate methods and statistical analyses, otherwise results and interpretations can be flawed. How research outcomes differ by sex, for example, has historically been understudied, and only recently have policies been implemented to require such consideration in the design of a study (e.g., NIH, 2015).
Over two decades ago, the renowned biomedical statistician Doug Altman labeled methodological weaknesses a “scandal”, raising awareness of shortcomings related to the representativeness of research as well as inappropriate research designs and statistical analysis (Altman, 1994). These methodological weaknesses extend to research on sex differences: simply adding female cells, animals, or participants to experiments does not guarantee an improved understanding of this field of research. Rather, the experiments must also be correctly designed and analyzed appropriately to examine such differences. While guidance exists for proper analysis of sex differences, the frequency of errors in published research articles related to this topic has not been well understood (e.g., Beltz et al., 2019).
Now, in eLife, Yesenia Garcia-Sifuentes and Donna Maney of Emory University fill this gap by surveying the literature to examine whether the statistical analyses used in different research articles are appropriate to support conclusions of sex differences (Garcia-Sifuentes and Maney, 2021). Drawing from a previous study that surveyed articles studying mammals from nine biological disciplines, Garcia-Sifuentes and Maney sampled 147 articles that included both males and females and performed an analysis by sex (Woitowich et al., 2020).
Over half of the articles surveyed (83, or 56%) reported a sex difference. Garcia-Sifuentes and Maney examined the statistical methods used to analyze sex differences and found that over a quarter (24 out of 83) of these articles did not perform or report a statistical analysis supporting the claim of a sex difference. A factorial design with sex as a factor is an appropriate way to examine sex differences in response to treatment, by giving each sex each treatment option (such as a treatment or control diet; see Figure 1A). A slight majority of all articles (92, or 63%) used a factorial design. Within the articles using a factorial design, however, less than one third (27) applied and reported a method appropriate to test for sex differences (e.g., testing for an interaction between sex and the exposure, such as different diets; Figure 1B). Similarly, within articles that used a factorial design and concluded a sex-specific effect, less than one third (16 out of 53) used an appropriate analysis.
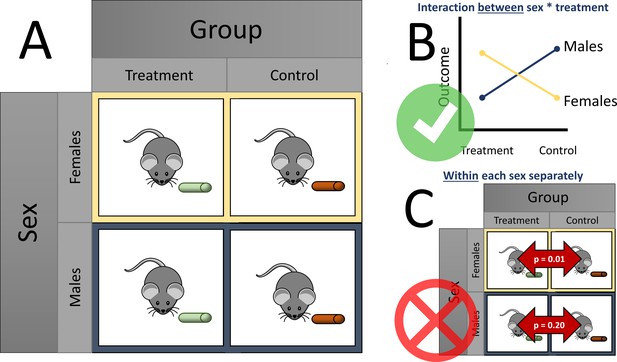
Considering sex differences in experimental design.
(A) A so-called factorial design permits testing of sex differences. For example, both female (yellow boxes) and male mice (blue boxes) are fed either a treatment diet (green pellets) or control diet (orange pellets). Garcia-Sifuentes and Maney found that 63 % of articles employed a factorial design in at least one experiment with sex as a factor. (B) An appropriate way to statistically test for sex differences is with a two-way analysis of variance (ANOVA). If a statistically significant interaction is observed between sex and treatment, as shown in the figure, evidence for a sex difference is supported. Garcia-Sifuentes and Maney found that in studies using a factorial design, less than one third tested for an interaction between sex and treatment. (C) Performing a statistical test between the treatment and control groups within each sex, and comparing the nominal statistical significance, is not a valid method to look for sex differences. Yet, this method was used in nearly half of articles that used a factorial design and concluded a sex-specific effect.
Notably, nearly half of the articles (24 out of 53) that concluded a sex-specific effect statistically tested the effect of treatment within each sex and compared the resulting statistical significance. In other words, when one sex had a statistically significant change and the other did not, the authors of the original studies concluded that a sex difference existed. This approach, which is sometimes called ‘differences in nominal significance’, or ‘DINS’ error (George et al., 2016), is invalid and has been found to occur for decades among several disciplines, including neuroscience (Nieuwenhuis et al., 2011), obesity and nutrition (Bland and Altman, 2015; George et al., 2016; Vorland et al., 2021), and more general areas (Gelman and Stern, 2006; Makin, 2019; Matthews and Altman, 1996; Sainani, 2010; Figure 1C).
This approach is invalid because testing within each sex separately inflates the probability of falsely concluding that a sex-specific effect is present compared to testing between them directly. Other inappropriate analyses that were identified in the survey included testing sex within treatment and ignoring control animals; not reporting results after claiming to do an appropriate analysis; or claiming an effect when the appropriate analysis was not statistically significant despite subscribing to ‘null hypothesis significance’ testing. Finally, when articles pooled the data of males and females together in their analysis, about half of them did not first test for a sex difference, potentially masking important differences.
The results of Garcia-Sifuentes and Maney highlight the need for thoughtful planning of study design, analysis, and communication to maximize our understanding and use of biological sex differences in practice. Although the survey does not quantify what proportion of this research comes to incorrect conclusions from using inappropriate statistical methods, which would require estimation procedures or reanalyzing the data, many of these studies’ conclusions may change if they were analyzed correctly. Misleading results divert our attention and resources, contributing to the larger problem of ‘waste’ in biomedical research, that is, the avoidable costs of research that does not contribute to our understanding of what is true because it is flawed, methodologically weak, or not clearly communicated (Glasziou and Chalmers, 2018).
What can the scientific enterprise do about this problem? The survey suggests that there may be a large variability in discipline-specific practices in the design, reporting, and analysis strategies to examine sex differences. Although larger surveys are needed to assess these more comprehensively, they may imply that education and support efforts could be targeted where they are most needed. Compelling scientists to publicly share their data can facilitate reanalysis when statistical errors are discovered – though the burden on researchers performing the reanalysis is not trivial. Partnering with statisticians in the design, analysis, and interpretation of research is perhaps the most effective means of prevention.
Scientific research often does not reflect the diversity of those who benefit from it. Even when it does, using methods that are inappropriate fails to support the progress toward equity. Surely this is nothing less than a scandal.
References
-
Analysis of sex differences in pre-clinical and clinical data setsNeuropsychopharmacology 44:2155–2158.https://doi.org/10.1038/s41386-019-0524-3
-
Best (but oft forgotten) practices: testing for treatment effects in randomized trials by separate analyses of changes from baseline in each group is a misleading approachThe American Journal of Clinical Nutrition 102:991–994.https://doi.org/10.3945/ajcn.115.119768
-
The difference between “significant” and “not significant” is not itself statistically significantThe American Statistician 60:328–331.https://doi.org/10.1198/000313006X152649
-
Erroneous analyses of interactions in neuroscience: a problem of significanceNature Neuroscience 14:1105–1107.https://doi.org/10.1038/nn.2886
-
WebsiteConsideration of Sex as a Biological Variable in NIH-funded ResearchAccessed October 13, 2021.
Article and author information
Author details
Publication history
Copyright
© 2021, Vorland
This article is distributed under the terms of the Creative Commons Attribution License, which permits unrestricted use and redistribution provided that the original author and source are credited.
Metrics
-
- 3,646
- views
-
- 256
- downloads
-
- 12
- citations
Views, downloads and citations are aggregated across all versions of this paper published by eLife.
Download links
Downloads (link to download the article as PDF)
Open citations (links to open the citations from this article in various online reference manager services)
Cite this article (links to download the citations from this article in formats compatible with various reference manager tools)
Further reading
-
- Medicine
- Microbiology and Infectious Disease
Persisting HIV reservoir viruses in resting CD4 T cells and other cellular subsets are a barrier to cure efforts. Early antiretroviral therapy (ART) enables post-treatment viral control in some cases, but mechanisms remain unclear. We hypothesised that ART initiated before peak viremia impacts HIV-1 subtype C reservoirs. We studied 35 women at high risk of infection from Durban, South Africa, identified with hyperacute HIV by twice-weekly HIV-RNA testing. Participants included 11 starting ART at a median of 456 (297–1203) days post-onset of viremia (DPOV) and 24 at 1 (1–3) DPOV. Peripheral blood mononuclear cells (PBMCs) were used to measured total HIV-1 DNA by droplet digital PCR (ddPCR) and sequence viral reservoir genomes by full-length proviral sequencing (FLIP-seq). ART during hyperacute infection blunted peak viremia (p<0.0001), but contemporaneous total HIV-1 DNA did not differ (p=0.104). Over 1 year, a decline of total HIV-1 DNA was observed in early treated persons (p=0.0004), but not late treated. Among 697 viral genome sequences, the proviral genetic landscape differed between untreated, late treated, and early treated groups. Intact genomes after 1 year were higher in untreated (31%) versus late treated (14%) and early treated (0%). Treatment in both late and early infection caused more rapid decay of intact (13% and 51% per month) versus defective (2% and 35%) viral genomes. However, intact genomes persisted 1 year post chronic treatment but were undetectable with early ART. Early ART also reduced phylogenetic diversity of intact genomes and limited cytotoxic T lymphocyte immune escape variants in the reservoir. Overall, ART initiated in hyperacute HIV-1 subtype C infection did not impact reservoir seeding but was associated with rapid intact viral genome decay, reduced genetic complexity, and limited immune escape, which may accelerate reservoir clearance in combination with other interventional strategies.
-
- Cancer Biology
- Medicine
A doctoral-level internship program was developed at the University of North Carolina at Chapel Hill with the intent to create customizable experiential learning opportunities for biomedical trainees to support career exploration, preparation, and transition into their postgraduate professional roles. We report the outcomes of this program over a 5-year period. During that 5-year period, 123 internships took place at over 70 partner sites, representing at least 20 academic, for-profit, and non-profit career paths in the life sciences. A major goal of the program was to enhance trainees’ skill development and expertise in careers of interest. The benefits of the internship program for interns, host/employer, and supervisor/principal investigator were assessed using a mixed-methods approach, including surveys with closed- and open-ended responses as well as focus group interviews. Balancing stakeholder interests is key to creating a sustainable program with widespread support; hence, the level of support from internship hosts and faculty members were the key metrics analyzed throughout. We hypothesized that once a successful internship program was implemented, faculty culture might shift to be more accepting of internships; indeed, the data quantifying faculty attitudes support this. Furthermore, host motivation and performance expectations of interns were compared with results achieved, and this data revealed both expected and surprising benefits to hosts. Data suggests a myriad of benefits for each stakeholder group, and themes are cataloged and discussed. Program outcomes, evaluation data, policies, resources, and best practices developed through the implementation of this program are shared to provide resources that facilitate the creation of similar internship programs at other institutions. Program development was initially spurred by National Institutes of Health pilot funding, thereafter, successfully transitioning from a grant-supported model, to an institutionally supported funding model to achieve long-term programmatic sustainability.