Cortical Development: Do progenitors play dice?
Understanding how the many different cell types that make up an adult organism emerge from the successive divisions of a single cell is a central question in biology. In particular, given the numerous random interactions that happen between and within cells (Vogt, 2015; Symmons and Raj, 2016; Hiesinger and Hassan, 2018), how do these multiple cell types reproducibly organize themselves into a robust body structure?
Consider the neocortex, a region of the brain that is involved in higher-order functions such as cognition and language. The neocortex contains a large number of different types of nerve cells called neurons, which are organized into six distinct layers. Neurons within each layer express specific genes and have distinct patterns of connections, which are key features for establishing proper brain circuitry (Jabaudon, 2017).
As the embryo develops, neurons are generated from mother cells called progenitors located below the neocortex: deep-layer neurons are born first, followed by superficial-layer neurons, in an 'inside-out' pattern during neocortical development. Although molecularly distinct mature neocortical neurons have been identified within each of the six layers (Tasic et al., 2016), a corresponding set of molecularly diverse neocortical progenitors has not been found (Telley et al., 2019). Thus, it remains unclear how exactly distinct neuron types emerge from a seemingly more uniform pool of progenitor cells.
Now, in eLife, Oscar Marín from King’s College London and co-workers – including Alfredo Llorca as first author – report that stochastic, random processes may explain how single progenitors are able to generate a wide range of neuron types (Llorca et al., 2019). The team – which includes researchers at King's College, the IST in Austria, the University of Freiburg and the University of Sussex – used three different techniques to genetically label progenitor cells, and then mapped the lineages (i.e. the neocortical clone) produced by each progenitor, focusing on progenitors that started generating neurons early during neocortical development. Of the clones that Llorca et al. were able to characterize, ~80% had daughter neurons in both the deep and superficial layers of the neocortex, while only ~20% had daughter neurons restricted to one of these two tiers. The neurons generated from individual progenitors also showed various axonal projection patterns and molecular identities, which corresponded to their layer position.
While this type of analyses has been done previously (Gao et al., 2014), these results provide important and thorough cross-validations of experimentally challenging approaches. In addition, Llorca et al. went one step further and developed mathematical models that could emulate their biological observations. They found that a model containing two types of progenitor cell which could randomly generate diverse ranges of neuron types was the best fit for their experimental results. These computer simulations suggest that a limited number of progenitors with a stochastic neuronal output can in principle account for the diverse clone types observed in the adult neocortex (Figure 1).
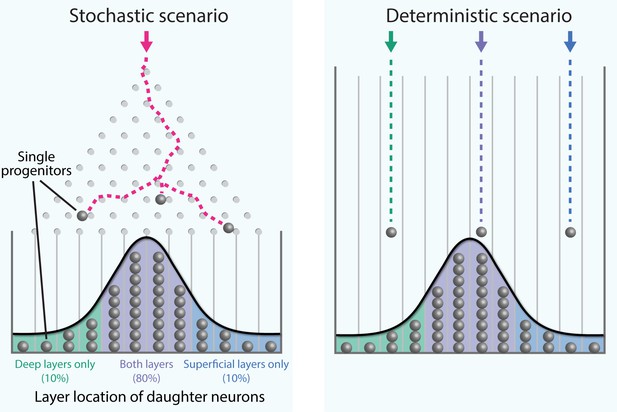
Stochastic generation of wide ranges of neuron types by single cortical progenitors.
The path taken by a single marble as it rolls down an inclined plane through an array of pegs and into a row of bins (a device called a Galton board; see video for a live-action example) cannot be predicted (left). However, over time a distribution of marbles in the bins builds up, and when the number of marbles is large enough this distribution can be predicted. Llorca et al. propose that the diversity in the output of single progenitors in the mouse neocortex is best explained by a small number of progenitors undergoing such stochastic (i.e. random) fate choices, rather than each type of adult neuron developing in a deterministic manner from a corresponding fate-restricted progenitor (right). The distribution of progenitors within the bins reflects the experimentally observed laminar position of their daughter neurons.
Stochastic events are critical for other developmental processes (Vogt, 2015; Symmons and Raj, 2016; Hiesinger and Hassan, 2018), including the fate choices of blood cell progenitors (Chang et al., 2008). However, distinguishing genuinely stochastic processes from unidentified deterministic processes is difficult. While modern technologies are becoming more sensitive, their signal-to-noise ratio is not infinite, which means that complex deterministic processes involving multiple factors can appear to be stochastic.
Although the stochastic hypothesis of Llorca et al. is based on simulations, it is provocative and raises exciting questions that can be experimentally tested. For example, can the two progenitor subtypes proposed by the model be molecularly identified? Do stochastic events occur within progenitors themselves or in their neuronal progenies? And when it comes to shaping the fate of a neuronal cell, what are the relative contributions of stochastic and deterministic processes?
Most importantly perhaps, this study highlights the limits of a reductionist, cell-by-cell description of neocortical and embryo development. If dynamic cellular features are predictable at a population rather than single-cell level, developmental processes may be most efficiently addressed by understanding the emergent properties of populations of cells rather than by a detailed account of their individual components.
References
-
The evolution of variability and robustness in neural developmentTrends in Neurosciences 41:577–586.https://doi.org/10.1016/j.tins.2018.05.007
-
Fate and freedom in developing neocortical circuitsNature Communications 8:16042.https://doi.org/10.1038/ncomms16042
-
Adult mouse cortical cell taxonomy revealed by single cell transcriptomicsNature Neuroscience 19:335–346.https://doi.org/10.1038/nn.4216
Article and author information
Author details
Publication history
Copyright
© 2020, Klingler and Jabaudon
This article is distributed under the terms of the Creative Commons Attribution License, which permits unrestricted use and redistribution provided that the original author and source are credited.
Metrics
-
- 1,906
- views
-
- 295
- downloads
-
- 12
- citations
Views, downloads and citations are aggregated across all versions of this paper published by eLife.
Download links
Downloads (link to download the article as PDF)
Open citations (links to open the citations from this article in various online reference manager services)
Cite this article (links to download the citations from this article in formats compatible with various reference manager tools)
Further reading
-
- Developmental Biology
Hair follicle development is initiated by reciprocal molecular interactions between the placode-forming epithelium and the underlying mesenchyme. Cell fate transformation in dermal fibroblasts generates a cell niche for placode induction by activation of signaling pathways WNT, EDA, and FGF in the epithelium. These successive paracrine epithelial signals initiate dermal condensation in the underlying mesenchyme. Although epithelial signaling from the placode to mesenchyme is better described, little is known about primary mesenchymal signals resulting in placode induction. Using genetic approach in mice, we show that Meis2 expression in cells derived from the neural crest is critical for whisker formation and also for branching of trigeminal nerves. While whisker formation is independent of the trigeminal sensory innervation, MEIS2 in mesenchymal dermal cells orchestrates the initial steps of epithelial placode formation and subsequent dermal condensation. MEIS2 regulates the expression of transcription factor Foxd1, which is typical of pre-dermal condensation. However, deletion of Foxd1 does not affect whisker development. Overall, our data suggest an early role of mesenchymal MEIS2 during whisker formation and provide evidence that whiskers can normally develop in the absence of sensory innervation or Foxd1 expression.
-
- Developmental Biology
Wing dimorphism is a common phenomenon that plays key roles in the environmental adaptation of aphid; however, the signal transduction in response to environmental cues and the regulation mechanism related to this event remain unknown. Adenosine (A) to inosine (I) RNA editing is a post-transcriptional modification that extends transcriptome variety without altering the genome, playing essential roles in numerous biological and physiological processes. Here, we present a chromosome-level genome assembly of the rose-grain aphid Metopolophium dirhodum by using PacBio long HiFi reads and Hi-C technology. The final genome assembly for M. dirhodum is 447.8 Mb, with 98.50% of the assembled sequences anchored to nine chromosomes. The contig and scaffold N50 values are 7.82 and 37.54 Mb, respectively. A total of 18,003 protein-coding genes were predicted, of which 92.05% were functionally annotated. In addition, 11,678 A-to-I RNA-editing sites were systematically identified based on this assembled M. dirhodum genome, and two synonymous A-to-I RNA-editing sites on CYP18A1 were closely associated with transgenerational wing dimorphism induced by crowding. One of these A-to-I RNA-editing sites may prevent the binding of miR-3036-5p to CYP18A1, thus elevating CYP18A1 expression, decreasing 20E titer, and finally regulating the wing dimorphism of offspring. Meanwhile, crowding can also inhibit miR-3036-5p expression and further increase CYP18A1 abundance, resulting in winged offspring. These findings support that A-to-I RNA editing is a dynamic mechanism in the regulation of transgenerational wing dimorphism in aphids and would advance our understanding of the roles of RNA editing in environmental adaptability and phenotypic plasticity.