Pre-existing chromosomal polymorphisms in pathogenic E. coli potentiate the evolution of resistance to a last-resort antibiotic
Abstract
Bacterial pathogens show high levels of chromosomal genetic diversity, but the influence of this diversity on the evolution of antibiotic resistance by plasmid acquisition remains unclear. Here, we address this problem in the context of colistin, a ‘last line of defence’ antibiotic. Using experimental evolution, we show that a plasmid carrying the MCR-1 colistin resistance gene dramatically increases the ability of Escherichia coli to evolve high-level colistin resistance by acquiring mutations in lpxC, an essential chromosomal gene involved in lipopolysaccharide biosynthesis. Crucially, lpxC mutations increase colistin resistance in the presence of the MCR-1 gene, but decrease the resistance of wild-type cells, revealing positive sign epistasis for antibiotic resistance between the chromosomal mutations and a mobile resistance gene. Analysis of public genomic datasets shows that lpxC polymorphisms are common in pathogenic E. coli, including those carrying MCR-1, highlighting the clinical relevance of this interaction. Importantly, lpxC diversity is high in pathogenic E. coli from regions with no history of MCR-1 acquisition, suggesting that pre-existing lpxC polymorphisms potentiated the evolution of high-level colistin resistance by MCR-1 acquisition. More broadly, these findings highlight the importance of standing genetic variation and plasmid/chromosomal interactions in the evolutionary dynamics of antibiotic resistance.
Editor's evaluation
This paper combines evolution experiments with genomic analysis of environmental samples to study the evolution of colistin resistance in Escherichia coli. It highlights the importance of pre-existing genomic variations on the chromosomes of clinical strains in driving the evolution of antibiotic resistance. The results are relevant for clinical and non-clinical microbiologists studying antibiotic resistance to last-resort drugs like colistin. The design of the research is simple and elegant, and the genomic data analysis connects the in vitro findings to the real world.
https://doi.org/10.7554/eLife.78834.sa0Introduction
Decades of research on the genetics of antibiotic resistance have shown that pathogenic bacteria evolve resistance to clinically relevant doses of antibiotics by acquiring a small number of mutations or by acquiring mobile elements carrying dedicated antibiotic resistance gene (MacLean and San Millan, 2019; Partridge et al., 2018). An open and unresolved question in evolutionary biology and genetics is the extent to which the other genes in bacterial genomes contribute to the evolution of resistance by modifying the rate of acquisition and effects of resistance determinants (Lukačišinová et al., 2020; Vogwill et al., 2014; Bethke et al., 2020; Card et al., 2019; Vogwill et al., 2016; Bottery et al., 2017; MacLean et al., 2010; Torres Ortiz et al., 2021).
Many of the bacterial pathogens where resistance poses a high risk to public health are opportunistic pathogens with high levels of chromosomal genetic diversity (Baker et al., 2018; Didelot et al., 2012; Smith et al., 2000). Although these pathogens acquire resistance determinants against a backdrop of extensive chromosomal diversity, the importance of this diversity in shaping the evolution of resistance remains poorly understood (Torres Ortiz et al., 2021; Baker et al., 2018; Didelot et al., 2012; Smith et al., 2000; Papkou et al., 2020). One approach to addressing this problem has been to challenge diverse collections of clinical/environmental strains with evolving resistance to a controlled antibiotic pressure using laboratory experimental evolution (Vogwill et al., 2014; Bethke et al., 2020; Papkou et al., 2020; Gifford et al., 2018). These studies have shown that strains evolve resistance at different rates, and it has been possible to identify ‘potentiator’ genes that accelerate the evolution of resistance by epistatically increasing the effects of canonical chromosomal resistance mutations (Papkou et al., 2020; Gifford et al., 2018). For example, strains of Staphylococcus aureus with high levels of expression of the norA efflux pump rapidly evolve resistance to fluoroquinolone antibiotics because high levels of norA expression increase the effect of classical fluoroquinolone resistance mutations in DNA topoisomerase (Papkou et al., 2020). Although these studies have focussed on resistance evolution by chromosomal mutations, most resistance in important pathogenic bacteria is caused by the acquisition of plasmids carrying mobile resistance genes (MacLean and San Millan, 2019; Partridge et al., 2018). It is equally conceivable that chromosomal polymorphisms shape the evolution of resistance by altering the acquisition and fitness effects of horizontally acquired resistance genes (Bottery et al., 2017).
One important challenge of using experimental evolution to identify potentiator genes is that pathogen isolates often differ from each other by thousands of single-nucleotide polymorphisms (SNPs) and variation in the presence/absence of accessory genes. Previous studies have used transcriptomic methods and genome-wide association study to identify genes that cause variation in evolvability between strains, but identifying these causal variants is challenging using this approach (Torres Ortiz et al., 2021; Baker et al., 2018; Didelot et al., 2012; Smith et al., 2000; Papkou et al., 2020). Given that potentiator genes interact epistatically with resistance genes, it follows that the presence of a resistance gene should also generate selection on potentiator genes under antibiotic pressure. According to this logic, comparing genomic changes between populations that carry established resistance determinants and sensitive control populations that arise during selection for elevated antibiotic resistance should provide a simple way to search for potentiator genes that interact epistatically with classical ‘resistance’ genes.
To test this idea, we challenged populations of Escherichia coli carrying an MCR-1 (mobile colistin resistance) plasmid and wild-type control populations with adapting to colistin, a last line of defence antibiotic that is used in the treatment of infections caused by multidrug-resistant pathogens (Li et al., 2006). E. coli has adapted to the widespread use of colistin in agriculture by acquiring a range of conjugative plasmids carrying the MCR-1 gene (Liu et al., 2016; Sun et al., 2018; Wang et al., 2018). MCR-1 is selectively advantageous in the presence of colistin (Liu et al., 2016; Sun et al., 2018), but it generates a colistin resistance phenotype that is of borderline clinical significance (Liu et al., 2016; Bardet et al., 2017; Doumith et al., 2016; Yang et al., 2017). In our experiments, MCR-1 populations consistently evolved high-level colistin resistance by acquiring mutations in lpxC, an essential gene involved in lipopolysaccharide (LPS) biosynthesis, that interacts epistatically with MCR-1 to increase resistance. Wild-type populations, on the other hand, were unable to evolve increased colistin resistance under the strong selective regime used in our experiments. We then tested for associations between MCR-1 and lpxC polymorphisms in pathogenic and commensal E. coli using large genomic datasets to better understand the consequences of this interaction in vivo.
Results
Accelerated evolution of colistin resistance in MCR-1 E. coli
To search for genes that interact with MCR-1 to increase colistin resistance, we evolved populations of E. coli carrying MCR-1 encoded on an IncX4 plasmid (MCREC) and wild-type control populations under strong selection for increased colistin resistance. Our selection experiment used an ‘evolutionary ramp’ in which the concentration of colistin was doubled daily from a very low dose (1/8 minimum inhibitory concentration [MIC]) to a very high dose (16× MIC) (see Methods). Antibiotic doses were standardized relative to the MICs of the two parental strains to ensure that the strength of selection was equivalent for the two strains. The rapid increase in antibiotic concentration in the ‘evolutionary ramp’ treatment ensures that populations must either evolve increased resistance or face extinction (once concentrations exceed the MIC of the parental strains) (Gifford et al., 2018; San Millan et al., 2016). Thus, the rate of populations extinction defines the measure of the evolvability of a strain.
Previous selection experiments have shown that mutants with low resistance tend to have high resistance evolvability, generating characteristic patterns of diminishing returns adaptation (MacLean et al., 2010) that has been reported for other microbial phenotypes (Kryazhimskiy et al., 2014; Wiser et al., 2013). All else being equal, we would therefore expect that control populations (initial MIC = 0.5mg/l) would have higher evolvability than the MCREC populations (initial MIC = 4mg/l). In contrast to this expectation, MCREC populations showed consistent evidence of adaptation to colistin, whereas only a very small minority of control populations were able to evolve increased resistance (Figure 1; proportional hazard likelihood ratio = 116, p = <0.0001).
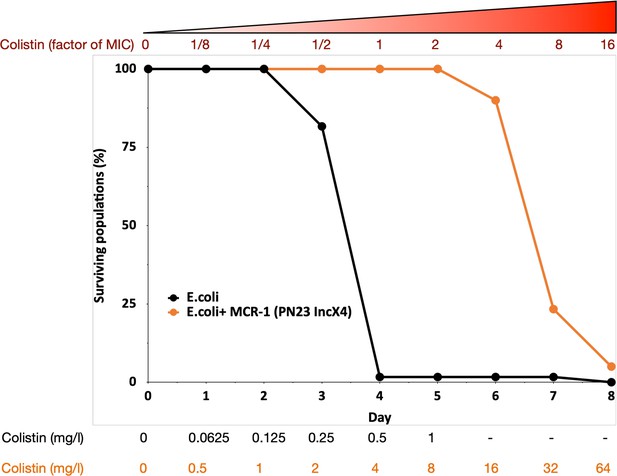
MCR-1 carrying bacterial populations (MCREC) show accelerated evolution of high-level colistin resistance.
The plot shows the survival of wild-type and MCR-1 carrying populations of E. coli (n = 120) populations for E. coli and n = 60 populations for E. coli + MCR-1 (PN23 IncX4) over time under increasing selection for colistin resistance. MCR-1 carrying populations showed increased survival, which is indicative of higher colistin evolvability. The numbers shown at the bottom represent the colistin concentration for wild-type E. coli (black) and for E. coli with MCR-1 (orange).
Mechanistic basis of the evolution of high-level colistin resistance
The increased evolvability of MCREC populations suggests that the presence of MCR-1 opened up new pathways to evolving elevated colistin resistance that were not accessible to wild-type populations. To test this idea, we sequenced the genomes of n = 21 independently evolved MCREC clones from day 6 of the experiment (4× MIC). All of the evolved clones had increased colistin resistance (mean MIC = 19.2mg/l, range = 8–32mg/l) compared to the MCREC parental strain (MIC = 4mg/l) (Supplementary file 1).
Strikingly, the majority of MCREC clones (n = 11/21) carried mutations in LpxC (Figure 2a), an UDP-3-O-acyl-N-acetylglucosamine deacetylase that catalyses the first step in lipid A biosynthesis and is important for membrane composition and asymmetry (Guest et al., 2021; Fivenson and Bernhardt, 2020). lpxC is an essential gene (Beall and Lutkenhaus, 1987), suggesting that its evolution should be constrained. Consistent with this idea, lpxC mutations were clustered in the coding sequence and both of the observed deletions kept the coding sequence in frame. Interestingly, several clones had mutations in the metal- (zinc) binding domain site which is very important for lpxC activity (Jackman et al., 2001; Figure 2b). For example, mutations at positions 79, 238, 242, and 246 lead to a hundred to thousand-fold decrease in lpxC activity (Jackman et al., 2001; Clayton et al., 2013; Lee et al., 2014), and many of our mutations map closely to these important sites (Figure 2b). Mutations in other genes involved in LPS biosynthesis were found in evolved clones that lacked lpxC mutations, including lpxA (n = 2) and rfaY (n = 1) (Figure 2a). Interestingly, a minority of clones lacked mutations and/or structural variants (n = 4). The colistin resistance of these clones did not differ suggesting that adaptive plasticity in gene expression and/or unstable genetic changes (i.e., heteroresistance; Andersson et al., 2019) can also contribute to increased colistin resistance (Figure 2—figure supplement 1).
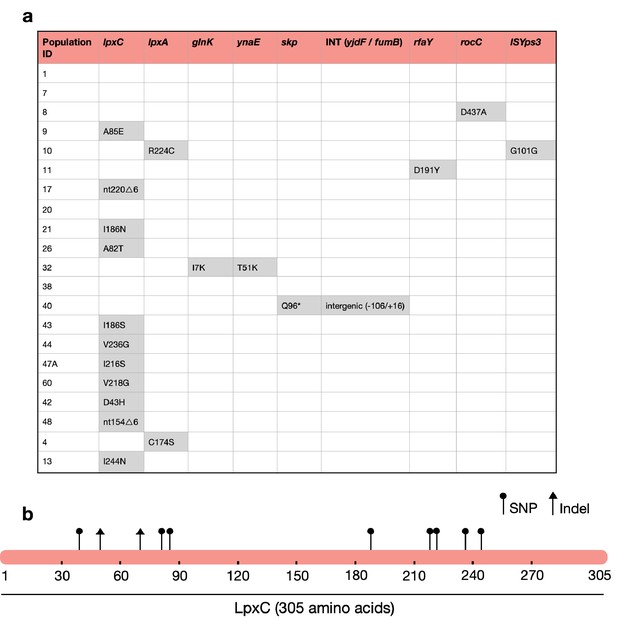
The majority of MCREC clones show mutations in LpxC.
(a) List of mutations identified in colistin-evolved MCR-1-positive clones. (b) Figure shows the mutations identified in LpxC. LpxC positions 79, 238, 242, and 246 are important for Zn binding and LpxC activity (Jackman et al., 2001). nt – nucleotide; △ – deletion; SNP – single-nucleotide polymorphism; Indel – insertions/deletions of nucleotides.
Testing for interactions between LpxC and MCR-1
The presence of lpxC mutations in MCREC populations suggests that the presence of MCR-1 increases the selective advantage provided by lpxC mutations in the presence of colistin. To test this hypothesis, we focussed on measuring the interaction between MCR-1 and a lpxC mutation from a domain that is critical for LpxC function (i.e., I244N). The MCR-1 natural plasmid used in our experiments (i.e., IncX4:MCR-1) is an intermediate size plasmid (approx. 34kb) that also carries genes for housekeeping and conjugation, as well as cargo genes of unknown function (Yang et al., 2017). To examine the interaction between LpxC and MCR-1 more directly, we cloned MCR-1 into pSEVA121, a low copy number (approx. 5 copies/cell) synthetic plasmid that confers a similar level of colistin resistance (MIC = 5mg/l) to the IncX4:MCR-1 plasmid used the selection experiment (MIC = 4mg/l). The key advantage of the pSEVA system is that this makes it possible to measure the impact of realistic levels of MCR-1 expression while controlling for any background plasmid effects using an empty vector control.
Our approach to measure the interaction between LpxC and MCR-1 was to test for epistasis between these genes in quantitative traits that were key targets of selection in our experiment. Epistasis occurs when different genes make a non-additive contribution to phenotypes (Durão et al., 2018). For any quantitative trait, epistasis (e) can be calculated as e = wAB*wab − wAbwaB where wAB is the value of the trait in the wild type, ab is the double mutants, and the single mutants are represented as Ab and aB (Trindade et al., 2009). Negative epistasis (e < 0) occurs when the value of the trait is less in the double mutant than would be expected from the single mutant, and vice versa for positive epistasis (e > 0). Although evolutionary biologists typically focus on epistatic interactions for fitness, the same methods can be applied to calculate epistasis for any quantitative trait.
The rapid increase in colistin concentration in our experiment implies that colistin resistance is likely to have been a key target of selection. MCR-1 increased the colistin resistance of the E. coli parental strain from 0.5 to 4mg/l, which is the clinical breakpoint MIC for colistin resistance (The European Committee on Antimicrobial Susceptibility Testing, 2022). The lpxC mutation, on the other hand, marginally decreased colistin resistance in the wild-type strain (~35% decrease in MIC) (Figure 3a). However, the combination of lpxC and MCR-1 increased colistin resistance to about 2× higher than the levels observed in the MCR-1 strain, revealing positive sign epistasis between lpxC and MCR-1 for colistin resistance (Figure 3a).
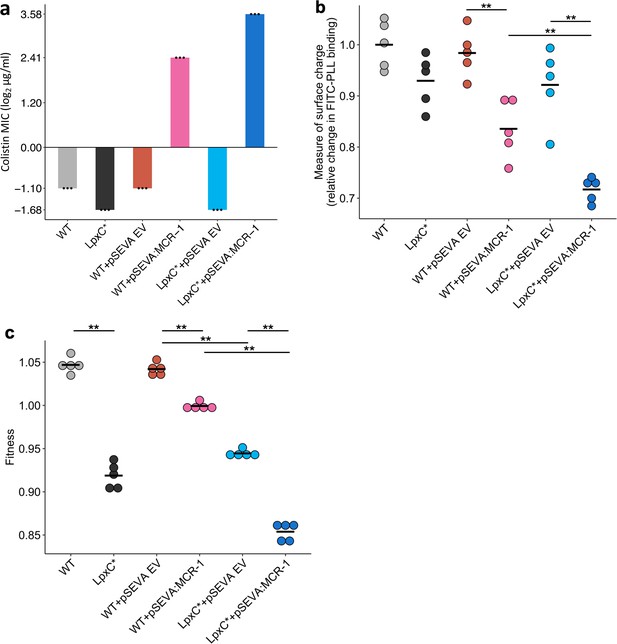
Effect of the lpxC mutation (lpxC*), with and without MCR-1, on bacterial susceptibility to colistin resistance (a), membrane surface charge (b), and fitness (c).
(a) Bacterial susceptibility to colistin was tested by measuring minimum inhibitory concentration (MIC) (n = 3 biological replicates per strain). (b) Relative change in membrane surface charge was measured by using fluorescein isothiocyanate-labelled poly-L-lysine (FITC-PLL)-binding assay (n = 5 biological replicates/strain) (see Methods). The changes were compared to wild-type (WT) control strain. (c) Bacterial fitness was measured using in vitro competition assay where strains were competed against a tester E. coli strain carrying a chromosomally integrated GFP (n = 5 biological replicates per competition). Statistical significance was determined by pairwise comparisons using the Wilcoxon rank-sum test, and double asterisks show differences with a p value <0.01. Central horizontal lines represent mean values of biological replicates. WT – wild type; EV – empty vector.
Electrostatic attractions occur between colistin, which is positively charged, and the bacterial outer membrane, which is negatively charged. MCR-1 increases resistance to colistin in part by decreasing the net negative charge on the bacterial outer membrane by modifying LPS (Sabnis et al., 2021). Given that lpxC is involved in LPS biosynthesis, we reasoned that lpxC and MCR-1 may interact epistatically to reduce membrane surface charge. As expected, the MCR-1 gene led to a substantial reduction in cell surface charge (Figure 3b). The lpxC mutation led to a small reduction in net cell surface charge that was not statistically significant (Wilcoxon rank-sum test p = 0.150). However, the combination of the lpxC mutation and MCR-1 reduced the surface charge to a greater extent than the MCR-1 alone (Figure 3b). The surface charge of the lpxC-MCR-1 double mutant was marginally less than the additive expectation, providing evidence of weak negative epistasis for cell surface charge (e = −0.059, error = 0.0355).
Antibiotic resistance is associated with costs that modulate the selective benefit of resistance (Andersson and Hughes, 2010). Previous work has shown that MCR-1 expression is associated with fitness costs (Yang et al., 2017), and it is possible that lpxC mutations provide a fitness advantage by compensating for the costs of MCR expression. To address this possibility, we measured the fitness of mutants in antibiotic-free culture medium. As expected, MCR-1 was associated with a fitness cost (Figure 3c). lpxC is an essential gene and extensive pleiotropic effects of lpxC mutations have been reported, including defective cell division, altered membrane composition, and leakage of periplasmic enzymes (Young and Silver, 1991; Kloser et al., 1996), suggesting that mutations in lpxC should be deleterious. Consistent with this idea, the lpxC mutation was associated with a high fitness cost in both the presence and absence of MCR-1, allowing us to rule out the possibility that lpxC mutations represent a compensatory adaptation for MCR-1 (Figure 3c). Interestingly, the fitness of double mutant was marginally less than we would expect under an additive model, revealing weak negative epistasis for fitness (ε = −0.0244, error = 0.0089).
Association between MCR-1 and LpxC in pathogenic E. coli
The large-scale use of colistin as a growth promoter in agriculture drove the spread of MCR-1 across agricultural, environmental, and human-associated populations of E. coli in South East Asia (Sun et al., 2018; Wang et al., 2018). To test the importance of lpxC in colistin resistance, we searched for lpxC polymorphisms in a recently published dataset of MCREC isolates from China collected from a diversity of agricultural, environmental, and human-associated sources (Shen et al., 2020). Notably, the frequency of lpxC polymorphisms in pathogenic E. coli isolates collected from infections was higher than the level found in patients colonized with E. coli and in the environment (Figure 4—figure supplement 1). Colistin is used as a last line of defence antibiotic for the treatment of infections caused by multidrug-resistant pathogens (Li et al., 2006) and this association between lpxC and MCR-1 in pathogenic E. coli is worrying, as it suggests that high-level colistin resistance may be common in pathogenic MCR-1-positive isolates.
Although this association between MCR and lpxC mutations in pathogenic E. coli appears compelling, the population structure of E. coli differs between isolate sources. This is an important issue to address, as it is not clear a priori if the high diversity of lpxC in pathogenic E. coli reflects the high diversity of this niche as opposed to the high diversity of particular phylogroups. In particular, extraintestinal pathogenic E. coli usually belong to phylogroup B2 and D (Picard et al., 1999; Johnson et al., 2001; Moulin-Schouleur et al., 2007), with B2 the main lineage for the majority of bloodstream E. coli infections in the UK (Kallonen et al., 2017). Similarly, the majority of B2 isolates in the Shen dataset were from patients infected with MRCEC (n = 10/18). It is not clear whether these lpxC mutations in pathogenic MCREC were acquired after MCR-1 (as in our experiments) or if these are pre-existing lpxC polymorphisms that were present in the B2 phylogroup prior to the acquisition of MCR-1. To address this issue, we analysed lpxC diversity in genomic datasets of commensal, pathogenic, and animal-associated E. coli from the UK, where MCR-1 is effectively absent (Davies et al., 2020; Shaw et al., 2021). The diversity of lpxC was consistently highest in isolates from the B2 phylogroup in this MCR-1 naive population of E. coli (Figure 5), suggesting that high levels of lpxC in B2 pre-date the acquisition of MCR-1 plasmids by HGT, and that this phylogroup is effectively primed to evolve high-level colistin resistance by MCR-1 plasmid acquisition.
The vast majority of SNPs seen in lpxC were synonymous (n = 6746/6783), suggesting that lpxC is under strong purifying selection. Only 37/1429 isolates had non-synonymous alleles. Strikingly, the majority were within B2 from the Davies infection dataset (33/37, 89%), despite B2 making up only around half of the filtered dataset (n = 659, 46%). This greater proportion of isolates with non-synonymous SNPs in B2 compared to other phylogroups was significant (chi-squared test p < 0.001, X-squared = 26.7; 33/659 B2 vs. 44/771 non-B2). We verified this higher diversity of lpxC in phylogroup B2 using complete RefSeq assemblies (Figure 5—figure supplement 1). However, RefSeq isolates did not show a greater proportion of non-synonymous SNPs in B2, with both having ~2% of isolates with non-synonymous SNPs (chi-squared test p = 1, X-squared <0.001; 6/278 B2 vs. 35/1611 non-B2). It may be relevant that the Davies dataset was from bloodstream infections whereas RefSeq includes a diverse range of isolates.
The greater diversity of lpxC in the pathogenic B2 phylogroup across studies is intriguing. Levels of overall genetic diversity are known to differ between E. coli phylogroups, with phylogroup D having the highest nucleotide diversity across 112 core E. coli gene families in a recent study (Touchon et al., 2020). However, phylogroup B2 was found to have similar overall nucleotide diversity to major phylogroups such as A or B1, suggesting that our observation here of higher diversity in lpxC from phylogroup B2 is not simply an artefact of higher overall genetic diversity. Altogether, this analysis suggests that pathogenic populations of E. coli already contain higher levels of standing genetic variation in lpxC, which may pre-dispose them to evolving high-level colistin resistance by acquiring conjugative plasmids carrying MCR-1.
As a final test for the importance of interactions between lpxC and MCR-1 in clinical settings, we compared the mutations observed in our evolved clones with those found in B2 isolates. We found no shared mutations between the clinical isolates and the lab-evolved isolates, either at the level of individual nucleotides or codons (Figure 5—figure supplement 2). However, it is unclear if we should expect to see an overlap for two reasons.
First, the mutations found in clinical isolates likely reflect a combination of beneficial mutations, neutral mutations, and weakly deleterious mutations across multiple strains, whereas the mutations found in our isolates reflect mutations that were beneficial under colistin treatment in a single MCR-1 carrying strain. Given this, it is unclear to what extent we should expect to see a strong overlap of mutated sites, but this is nonetheless an interesting comparison to make.
Second, our data suggest that a large number of mutations in lpxC can interact with MCR-1 to increase colistin resistance. Experimental evolution studies have shown that while parallel evolution at the level of the gene is quite common, parallelism at the level of individual nucleotides is rare (Stern, 2013; Tenaillon et al., 2012; Schenk et al., 2022). The notable exception to this is cases where strong selective constraints limit the potential number of adaptive mutations, as has been observed for hotspot mutations in DNA gyrase and topoisomerase that are associated with fluoroquinolone resistance (Papkou et al., 2020; Wong et al., 2012). In our evolution experiment, we found no cases of parallel evolution at the nucleotide level and only a single example of codon-level parallelism (I86 S/N), giving an estimate of the probability of codon-level parallelism of (1/92 = 1/81). This low probability of parallel evolution implies that a very large number of mutations in lpxC are likely to interact epistatically with MCR-1 to increase resistance. Given this, it is perhaps not surprising that we did not observe any overlap between lab-evolved mutations and naturally occurring polymorphisms in lpxC. Furthermore, lpxC mutations will have many other impacts beyond colistin resistance. It seems plausible that pathogenic E. coli may have higher lpxC diversity due to the different host environment they may have recently inhabited compared to non-pathogenic E. coli.
Discussion
The acquisition of specialized antibiotic resistance genes through horizontal gene transfer has played a key role in the evolution of antibiotic resistance in pathogenic bacteria. One of the key insights from genomics studies has been that human pathogens are highly polymorphic (Baker et al., 2018; Didelot et al., 2012), but the role of chromosomal genetic variation in shaping the acquisition and fitness effects of mobile resistance genes remains poorly understood (Bottery et al., 2017; McNally et al., 2016; San Millan et al., 2015). Here, we show that the evolution of high-level colistin resistance is driven by epistatic interactions between the MCR-1 mobile colistin resistance and lpxC, a chromosomal gene involved in LPS biosynthesis (Figures 1—3). lpxC mutations are more common in pathogenic MCR-positive E. coli (MCREC) than commensal MCREC, highlighting the clinical relevance of this interaction (Figure 4). Crucially, lpxC polymorphisms are over-represented in pathogenic lineages for E. coli from populations with no history of MCR-1 acquisition, suggesting that pre-existing polymorphisms in lpxC potentiated the evolution of high-level colistin resistance through the acquisition of conjugative plasmids carrying MCR-1 (Figure 5). The underlying causes of high lpxC diversity in pathogenic E. coli are unclear, and we hope that future work will shed light on the impact of lpxC polymorphisms on E. coli virulence and infection.
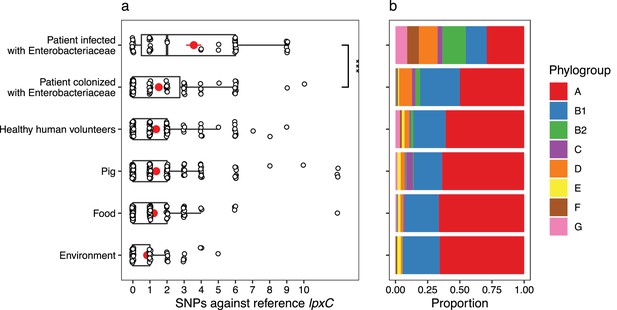
mcr-1-positive isolates from patients infected with Enterobacteriaceae have more mutations in lpxC compared to isolates from other sources.
Data shown are from n = 673 unfiltered MCR-1 plasmid carrying isolates collected in 2016 by Shen et al., 2020. (a) Single-nucleotide polymorphisms (SNPs) in lpxC determined by the alignment of the lpxC sequence for each assembled isolate against the reference lpxC sequence from E. coli MG1655 (NC_000913.3:106557–107474). Mean values (± standard error) are shown by red points (lines). Isolates from patients infected with Enterobacteriaceae have significantly more SNPs than other sources, for example, those from patients only colonized with Enterobacteriaceae (Wilcoxon rank-sum test, W = 1150, p < 0.001). ***p < 0.001. However, this difference is associated with (b) the population structure of E. coli, with a greater proportion of phylogroups B2 and D in isolates from infected patients. The same plot for filtered isolates (Figure 4—figure supplement 1) showed a similar pattern although the comparison between patients infected vs. colonized was not significant.
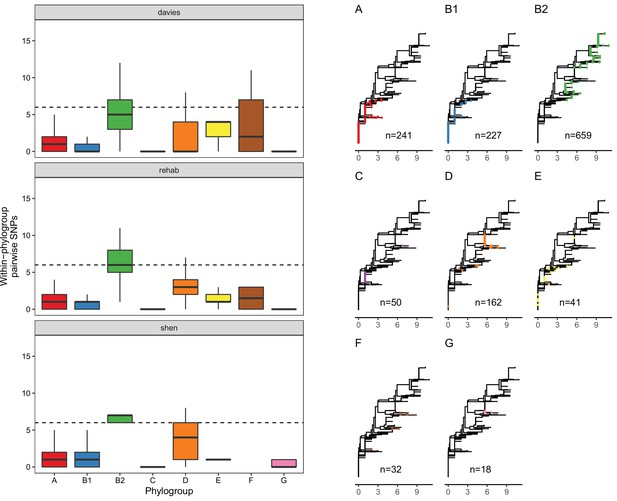
LpxC diversity in main E. coli phylogroups.
The diversity of lpxC within phylogroup B2 is greater across three different datasets (called Davies et al., 2020, Shaw et al., 2021, Shen et al., 2020) (see Methods for details). Left: boxplots of the pairwise single-nucleotide polymorphisms (SNPs) between lpxC sequences of the same phylogroup within different studies. Dashed line shows the median pairwise distance (six SNPs) between isolates of different phylogroups. Right: a midpoint-rooted neighbour-joining tree of lpxC diversity, with coloured tips in each panel showing the location of isolates from each main phylogroup (A-G). Assembled isolates were filtered to only include high-quality assemblies (see Methods).
One of the most surprising features of our results is that approximately 20% of clones that were recovered from MCR-1-positive populations growing at 4× MIC (16mg/l) did not have any detectable mutations or structural variants, a phenomenon that we have also reported in Pseudomonas aeruginosa (Kapel et al., 2022). There are a number of examples of adaptation to colistin through the acquisition of unstable resistance mutations that rapidly revert when selection for resistance is relaxed (i.e. heteroresistance Andersson et al., 2019). Importantly, we cultured clones for DNA extraction on a medium containing a low dose of colistin (1mg/l, ¼ MIC) that may not have generated effective selection for the maintenance of unstable resistance determinants. Alternatively, it is possible that these clones were sampled from populations that adapted to colistin phenotypically, for example, due to the growth of a subset of cells with altered expression of genes involved in LPS biosynthesis. Given the importance of colistin as a ‘last line of defence’ antibiotic, an important goal for future work will be to investigate the mechanisms that underpin revertible colistin resistance and how these interact with MCR-1.
There is growing evidence that standing genetic variation (i.e., pre-existing variation) in pathogen species has played an important role in the evolution of antibiotic resistance. For example, at least two of the major lineages of methicillin-resistant S. aureus originated well before the clinical introduction of methicillin (Harkins et al., 2017; Gill et al., 2021). Our study builds on previous work (Vogwill et al., 2014; Bethke et al., 2020; Papkou et al., 2020; Gifford et al., 2018) by demonstrating that epistatic interactions between mobile resistance genes and chromosomal variants shape the evolution of resistance. One limitation of our study is that we only focussed on interactions between the chromosome and the MCR-1 resistance gene. We suggest that an important goal for future work will be to study the importance of genetic interactions between bacterial chromosomes and mobile elements that transfer AMR genes (Partridge et al., 2018) more broadly. We speculate that chromosome–mobile element interactions likely to be particularly important for highly mobile resistance genes, such as MCR-1 (Liu et al., 2016; Wang et al., 2018; Wang et al., 2017) and carbapenemases (León-Sampedro et al., 2021) that are rapidly transferred between divergent bacterial strains and species. Our findings suggest that understanding interactions between mobile resistance genes and background genetic variation could help to develop methods for predicting the spread of resistance in pathogen populations, which is an important goal for evolutionary microbiology.
Methods
Bacterial strains and growth condition
All the experiments were carried out in E. coli J53. Experiments were conducted in Luria-Bertani (LB) medium (Sigma-Aldrich).
pSEVA:MCR-1 vector construction
A synthetic MCR-1 plasmid was constructed by cloning mcr-1 gene into pSEVA121 plasmid (Wong et al., 2012). The mcr-1 gene along with its natural promoter was amplified from the natural PN16 (IncI2) plasmid using Q5 High-Fidelity DNA Polymerase (New England BioLabs). The amplified and purified mcr-1 fragment was assembled together with PCR-amplified pSEVA121 backbone using NEBuilder HiFi DNA Assembly Master Mix according to the manufacturer’s instructions. The assembled plasmid was then transformed into E. coli J53 using the standard electroporation method. Cells were recovered in 1 ml SOC (super optimal broth with catabolite repression) medium, followed by 1-hr incubation at 37°C. Different dilutions of transformant mixture were made and were plated onto Petri dishes containing LB agar supplemented with 100 µg/ml ampicillin. The culture plates were incubated at 37°C for overnight. PCR and sequence verification by Sanger sequencing were performed to ensure the presence of the correctly assembled recombinant plasmid. A full list of the primers used is given in Supplementary file 2.
Experimental evolution
The experimental evolution experiment was carried out using our previously established ‘evolutionary ramp’ method (Gifford et al., 2018; San Millan et al., 2016). Briefly, wild-type E. coli (control) and E. coli with PN23 IncX4:MCR-1 plasmid were grown on LB agar medium supplemented with appropriate antibiotic at 37°C for overnight. To ensure reproducibility, we had 120 and 60 independent replicates for E. coli control and E. coli-MCR-1 strain (MCREC), respectively. Parallel cultures were propagated in 96-well microtitre plates where the inner 60 wells were used for bacterial cultures and the outer wells for blank measurement and contamination controls. We used checkerboard layout on the plate to control for potential cross-contaminations. The plates were incubated for 22 hr and then overnight grown cultures were diluted 1:400-fold into fresh LB medium plates with increasing concentration of colistin. The concentration of colistin was doubled daily from the very low dose (1/8 MIC) to a very high dose (16× MIC). At the end of each transfer, the optical density was measured, and the experiment was carried out until more than >90% of cultures have gone extinct (i.e. OD595 less than 0.05). In total, eight transfers were performed in LB medium with colistin. The evolved E. coli-MCR-1 and control populations were preserved at −80°C in 15% glycerol solution. We tested for a difference between the survival of control populations (n = 120) and plasmid carrying populations (n = 60) using Cox’s proportional hazard model. Each population was considered extinct on the day when OD fell below our 0.05 threshold.
DNA extraction, whole-genome sequencing, and data analysis
To identify the polymorphisms associated with colistin resistance evolution, we selected 21 evolved populations from day 6 (4× MIC). Bacterial DNA was extracted using DNeasy Blood & Tissue Kit (Qiagen) on a QIAcube automated system (Qiagen). The concentration of each genomic DNA sample was determined using a QuantiFluor dsDNA System (Promega) following the manufacturer’s protocol. The purified genomic DNA samples were subjected to Illumina HiSeq 4000 platform at the Oxford Genomics Centre (Wellcome Centre for Human Genetics, University of Oxford, UK). The ancestral isolate was sequenced on an Oxford nanopore MinION platform using a FLO-MIN106 flow-cell and the SQK-LSK109 Ligation Sequencing kit.
Raw Illumina reads were quality controlled with Trimmomatic v. 0.39 (Bolger et al., 2014) using the ILLUMINACLIP (2:30:10) and SLIDING WINDOW (4:15) parameters. Raw nanopore reads were basecalled using guppy v. 0.0.0+7,969d57 and reads were demultiplexed using qcat v. 1.1.0 (https://github.com/nanoporetech/qcat; Rescheneder, 2019). Resulting sequencing reads were assembled using unicycler v. 0.4.8 (Wick et al., 2017), which used SAMtools v. 1.9 (Li et al., 2009), pilon v. 1.23 (Walker et al., 2014), and bowtie2 v. 2.3.5.1 (Langmead and Salzberg, 2012), in hybrid mode with respective Illumina reads. The resulting assembly was annotated using Prokka v. 1.14.0 (Seemann, 2014).
Small variants were identified using breseq v. 0.34.0 (Deatherage and Barrick, 2014) in the polymorphism prediction mode, which implemented bowtie2 v. 2.3.5.1. Copy number variants were estimated with the default parameters of the package CNOGpro v. 1.1 (Brynildsrud et al., 2015) in R (R Development Core Team, 2021). The plasmid copy number was calculated by dividing the average sequencing coverage of each plasmid by the average sequencing coverage of the chromosome using the SAMtools depth algorithm (Figure 2—figure supplements 2–4).
Construction of I244N lpxC mutant (lpxC*)
A previously described pORTMAGE method (Nyerges et al., 2016) was applied to introduce I244N lpxC mutation into the wild-type E. coli J53. The mutant was constructed via synthetic ssDNA-mediated recombineering using the pORTMAGE3 plasmid. The MODEST tool was used to design 90 nucleotides long ssDNA oligos that had complementary sequences to the replicating lagging strand with a minimized secondary structure (≥−12 kcal mol−1) (Bonde et al., 2014). The oligos were ordered from Integrated DNA Technologies (Coralville, IA, USA). For the recombineering, pORTMAGE3 vector was transformed into E. coli J53 electrocompetent cells using standard electroporation method. E. coli carrying pORTMAGE3 vector was further used for recombineering. pORTMAGE3 was induced at 42°C for 15 min to allow for efficient mutation incorporation and to avoid off-target mutagenesis. 1 µl of the 100 µM mutation carrying oligo was transformed into induced electrocompetent cells. Following the electroporation, cells were recovered in 5 ml Terrific-Broth (TB) media (24 g yeast extract, 12 g tryptone, 9.4 g K2HPO4, and 2 g KH2PO4 per 1 l of water) and incubated at 30°C for 60 min. After the incubation period, 5 ml of LB medium was added, and cells were further incubated at 30°C overnight. Appropriate dilutions of the cultures were then plated onto LB agar plates to form individual colonies and incubated at 30°C overnight. Individual colonies were screened for the mutation using Sanger capillary-sequencing. Finally, the pORTMAGE3 plasmid was cured from the sequence-verified colony by growing the cells at 42°C for overnight in antibiotic-free LB medium.
A list of oligos used for the mutation construction is given in Supplementary file 2.
Determination of MIC
MICs were determined using a standard serial broth dilution method with a minor modification that we previously optimized to capture small differences in MIC (Kintses et al., 2019). Specifically, smaller colistin concentration steps were used (typically 1.5-fold). Ten-step serial dilution of colistin was prepared in fresh LB medium in 96-well microtitre plates. Three wells contained only LB medium to monitor the bacterial growth in the absence of colistin. Bacterial strains were grown in LB medium supplemented with appropriate antibiotic (100 μg/ml ampicillin for E. coli pSEVA:MCR-1) at 30°C for overnight. Following overnight incubation, approximately 5 × 105 cells were inoculated into the each well of the 96-well microtitre plate. Three independent replicates for each strain and the corresponding control were used. The top and bottom row in the 96-well plate were filled with LB medium to obtain the background OD value of the growth medium. Plates were incubated at 30°C with continuous shaking at 250 rpm. After 20–24 hr of incubation, OD600 values were measured in a microplate reader (Biotek Synergy 2). After background subtraction, MIC was defined as the lowest concentration of colistin where the OD600 <0.05.
Membrane surface charge measurement
To measure bacterial membrane surface charge, we carried out a fluorescein isothiocyanate-labelled poly-L-lysine- (FITC-PLL) (Sigma) binding assay (Kintses et al., 2019; Rossetti et al., 2004). FITC-PLL is a polycationic molecule that binds to an anionic lipid membrane in a charge-dependent manner and is used to investigate the interaction between cationic peptides and charged lipid bilayer membranes. The assay was performed as previously described (Kintses et al., 2019). Briefly, bacterial cells were grown overnight in LB medium, and then centrifuged, and washed twice with 1× phosphate-buffered saline (PBS) buffer (pH 7.4). The washed bacterial cells were re-suspended in 1× PBS buffer to a final OD600 of 0.1. A freshly prepared FITC-PLL solution was added to the bacterial suspension at a final concentration of 6.5 µg/ml. The suspension was incubated at room temperature for 10 min, and pelleted by centrifugation. The remaining amount of FITC-PLL in the supernatant was determined fluorometrically (excitation at 500 nm and emission at 530 nm) with or without bacterial exposure. The quantity of bound molecules was calculated from the difference between these values. A lower binding of FITC-PLL indicates a less net negative surface charge of the outer bacterial membrane.
In vitro competition assay
We carried out in vitro competition experiment using a flow cytometry-based sensitive and reproducible method developed in our laboratory (Gifford et al., 2018; San Millan et al., 2016; Jangir et al., 2022). Flow cytometry was performed on an Accuri C6 (Becton Dickenson, Biosciences, UK). The test strains were competed against a GFP-labelled E. coli strain J53 to measure the relative fitness. All competitions were carried out in LB medium with five biological replicates per strain, as previously described (San Millan et al., 2016; Jangir et al., 2022). Briefly, the bacterial cells were grown in LB medium for overnight. The overnight grown cultures were washed with filtered PBS buffer. The washed cells were diluted into a fresh LB medium and mixed approximately at 1:1 ratio with GFP-labelled E. coli J53. Before starting the competition, the total cell density in the competition mix was around half million cells, as we also used for MIC assay. The initial density of fluorescent and non-fluorescent cells was estimated in the mix using medium flow rate, recoding 10,000 events and discarding events with forward scatter <10,000 and side scatter <8000. After confirming the actual ratio close to 1:1, the competition plates were incubated at 30°C with shaking at 250 rpm. After overnight incubation, the competition mix was diluted in PBS buffer and cell densities were adjusted around 1000 per microlitre. The final density of fluorescent and non-fluorescent cells was estimated in the competition mix. Using the initial and final density of fluorescent and non-fluorescent cells, the relative fitness was calculated as described below:
where d0 and d1 represent cell density before and after the competition, respectively.
Analysis of E. coli genomes datasets
We downloaded three short-read datasets of E. coli genomes to investigate lpxC diversity: mcr-1-positive E. coli from China from a range of sources including human infection isolates, collected 2016–2018 (n = 688; Shen dataset) ( Shen et al., 2020); mcr-1-negative E. coli bloodstream infection isolates from Oxfordshire, UK, collected 2013–2015 (n = 981 short-read datasets from n = 976 isolates; Davies dataset) (Davies et al., 2020); and mcr-1-negative E. coli from UK, mainly from livestock, collected 2017 (n = 502; REHAB dataset) (Shaw et al., 2021).
Scripts and data for analysis, including assemblies, are available on figshare (doi:10.6084/m9.figshare.18591347). In brief, genomes for the Shen and Davies datasets were assembled from short reads downloaded from the NCBI SRA. These reads were filtered with Trimmomatic v0.39 with default parameters (Bolger et al., 2014) and assembled with Spades v3.15.3 (Bankevich et al., 2012). Genomes from the REHAB dataset were downloaded from previously assembled genomes from hybrid assembly. We screened genomes for a full-length lpxC using blastn against lpxC from E. coli MG1655 (NZ_LR881938.1_cds_WP_000595482.1_97; identical to lpxC in strain J53; both phylogroup A). We discarded genomes without a full-length lpxC match (n = 5), those where lpxC had >12 SNPs compared to the reference suggesting poor assembly (n = 20, all from Shen dataset) leaving n = 2146 isolates to analyse. Details of these isolates including accessions, phylogroup, and SNPs in lpxC are given in Supplementary file 3. We assigned phylogroup with ClermonTyper and analysed the n = 2,127 isolates which were in the main phylogroups (A, B1, B2, C, D, E, F, and G). To visualize lpxC diversity we plotted a midpoint-rooted neighbour-joining tree including the reference. After obtaining initial results similar to Figure 5 (not shown), we subsequently filtered isolates to include only higher-quality assemblies (L90 <100, <999 contigs) to ensure that assembly quality was not driving the patterns, resulting in n = 1429 isolates. Numbers given in Results refer to this filtered dataset.
To reproduce our finding of higher lpxC diversity in phylogroup B2 on another dataset, we downloaded all complete E. coli assemblies on NCBI RefSeq (as of 13 January 2022, n = 1903). Of these, n = 1887 (>99%) were from the main phylogroups and had a full-length lpxC with <13 SNPs against the reference lpxC.
Data availability
All data generated or analysed during this study are included in this article and its Supplementary Information. All sequencing data are available in the NCBI Sequence Read Archive, with accession numbers SRR19785165–SRR19785186.
-
NCBI BioProjectID PRJNA851773. Pre-existing chromosomal polymorphisms in pathogenic E. coli potentiate the evolution of resistance to a last-resort antibiotic.
References
-
Antibiotic resistance and its cost: is it possible to reverse resistance?Nature Reviews. Microbiology 8:260–271.https://doi.org/10.1038/nrmicro2319
-
Mechanisms and clinical relevance of bacterial heteroresistanceNature Reviews. Microbiology 17:479–496.https://doi.org/10.1038/s41579-019-0218-1
-
SPAdes: A new genome assembly algorithm and its applications to single-cell sequencingJournal of Computational Biology 19:455–477.https://doi.org/10.1089/cmb.2012.0021
-
Trimmomatic: a flexible trimmer for Illumina sequence dataBioinformatics 30:2114–2120.https://doi.org/10.1093/bioinformatics/btu170
-
MODEST: a web-based design tool for oligonucleotide-mediated genome engineering and recombineeringNucleic Acids Research 42:W408–W415.https://doi.org/10.1093/nar/gku428
-
Adaptive modulation of antibiotic resistance through intragenomic coevolutionNature Ecology & Evolution 1:1364–1369.https://doi.org/10.1038/s41559-017-0242-3
-
Structure of the bacterial deacetylase LpxC bound to the nucleotide reaction product reveals mechanisms of oxyanion stabilization and proton transferThe Journal of Biological Chemistry 288:34073–34080.https://doi.org/10.1074/jbc.M113.513028
-
Reconciling the potentially irreconcilable? Genotypic and phenotypic amoxicillin-clavulanate resistance in Escherichia coliAntimicrobial Agents and Chemotherapy 64:e02026-19.https://doi.org/10.1128/AAC.02026-19
-
Identification of mutations in laboratory-evolved microbes from next-generation sequencing data using breseqMethods in Molecular Biology 1151:165–188.https://doi.org/10.1007/978-1-4939-0554-6_12
-
Transforming clinical microbiology with bacterial genome sequencingNature Reviews. Genetics 13:601–612.https://doi.org/10.1038/nrg3226
-
Detection of the plasmid-mediated mcr-1 gene conferring colistin resistance in human and food isolates of Salmonella enterica and Escherichia coli in England and WalesThe Journal of Antimicrobial Chemotherapy 71:2300–2305.https://doi.org/10.1093/jac/dkw093
-
Evolutionary mechanisms shaping the maintenance of antibiotic resistanceTrends in Microbiology 26:677–691.https://doi.org/10.1016/j.tim.2018.01.005
-
Identifying and exploiting genes that potentiate the evolution of antibiotic resistanceNature Ecology & Evolution 2:1033–1039.https://doi.org/10.1038/s41559-018-0547-x
-
Border control: regulating LPS biogenesisTrends in Microbiology 29:334–345.https://doi.org/10.1016/j.tim.2020.09.008
-
Phylogenetic distribution of extraintestinal virulence-associated traits in Escherichia coliThe Journal of Infectious Diseases 183:78–88.https://doi.org/10.1086/317656
-
Fast gapped-read alignment with Bowtie 2Nature Methods 9:357–359.https://doi.org/10.1038/nmeth.1923
-
Structural basis of the promiscuous inhibitor susceptibility of Escherichia coli LpxCACS Chemical Biology 9:237–246.https://doi.org/10.1021/cb400067g
-
Colistin: the re-emerging antibiotic for multidrug-resistant Gram-negative bacterial infectionsThe Lancet. Infectious Diseases 6:589–601.https://doi.org/10.1016/S1473-3099(06)70580-1
-
The sequence alignment/map format and SAMtoolsBioinformatics 25:2078–2079.https://doi.org/10.1093/bioinformatics/btp352
-
The evolution of antibiotic resistanceScience 365:1082–1083.https://doi.org/10.1126/science.aax3879
-
Mobile genetic elements associated with antimicrobial resistanceClinical Microbiology Reviews 31:e00088-17.https://doi.org/10.1128/CMR.00088-17
-
The link between phylogeny and virulence in Escherichia coli extraintestinal infectionInfection and Immunity 67:546–553.https://doi.org/10.1128/IAI.67.2.546-553.1999
-
SoftwareR: A Language and Environment for Statistical ComputingR Foundation for Statistical Computing, Vienna, Austria.
-
Multicopy plasmids potentiate the evolution of antibiotic resistance in bacteriaNature Ecology & Evolution 1:10.https://doi.org/10.1038/s41559-016-0010
-
Prokka: rapid prokaryotic genome annotationBioinformatics 30:2068–2069.https://doi.org/10.1093/bioinformatics/btu153
-
Dynamics of mcr-1 prevalence and mcr-1-positive Escherichia coli after the cessation of colistin use as a feed additive for animals in China: a prospective cross-sectional and whole genome sequencing-based molecular epidemiological studyThe Lancet. Microbe 1:e34–e43.https://doi.org/10.1016/S2666-5247(20)30005-7
-
The genetic causes of convergent evolutionNature Reviews. Genetics 14:751–764.https://doi.org/10.1038/nrg3483
-
Towards understanding mcr-like colistin resistanceTrends in Microbiology 26:794–808.https://doi.org/10.1016/j.tim.2018.02.006
-
ReportBreakpoint tables for interpretation of MICs and zone diameters Version 12EUCAST.
-
Genomic signatures of pre-resistance in Mycobacterium tuberculosisNature Communications 12:7312.https://doi.org/10.1038/s41467-021-27616-7
-
Testing the role of genetic background in parallel evolution using the comparative experimental evolution of antibiotic resistanceMolecular Biology and Evolution 31:3314–3323.https://doi.org/10.1093/molbev/msu262
-
Epistasis between antibiotic resistance mutations and genetic background shape the fitness effect of resistance across species of PseudomonasProceedings. Biological Sciences 283:20160151.https://doi.org/10.1098/rspb.2016.0151
-
Unicycler: Resolving bacterial genome assemblies from short and long sequencing readsPLOS Computational Biology 13:e1005595.https://doi.org/10.1371/journal.pcbi.1005595
-
Leakage of periplasmic enzymes from envA1 strains of Escherichia coliJournal of Bacteriology 173:3609–3614.https://doi.org/10.1128/jb.173.12.3609-3614.1991
Article and author information
Author details
Funding
Wellcome Trust (106918/Z/15Z)
- R Craig MacLean
Medical Research Council (MR/S013768/1)
- R Craig MacLean
- Tim Walsh
Biotechnology and Biological Sciences Research Council (BB/M011224/1)
- Lois Ogunlana
National Natural Science Foundation of China (31200150)
- Qiue Yang
The funders had no role in study design, data collection, and interpretation, or the decision to submit the work for publication. For the purpose of Open Access, the authors have applied a CC BY public copyright license to any Author Accepted Manuscript version arising from this submission.
Acknowledgements
This work was supported by grants from the Wellcome Trust (106918/Z/15Z, CM) and the Medical Research Council (MR/S013768/1, TW and CM). LO was supported by the Biotechnology and Biological Sciences Research Council (BBSRC) doctoral training partnership (BB/M011224/1). YQE is supported by Laboratory of Lingnan Modern Agriculture Project NT2021010 and the National Natural Science Foundation of China (31200150). LPS is a Sir Henry Wellcome Postdoctoral Fellow funded by Wellcome (grant 220422/Z/20/Z). We thank Csaba Pal lab (Biological Research Centre, Szeged, Hungary) for providing the pORTMAGE plasmid. We also thank Ákos Nyerges (Harvard University) for the helpful discussion on pORTMAGE.
Copyright
© 2022, Jangir, Yang et al.
This article is distributed under the terms of the Creative Commons Attribution License, which permits unrestricted use and redistribution provided that the original author and source are credited.
Metrics
-
- 2,238
- views
-
- 336
- downloads
-
- 13
- citations
Views, downloads and citations are aggregated across all versions of this paper published by eLife.
Download links
Downloads (link to download the article as PDF)
Open citations (links to open the citations from this article in various online reference manager services)
Cite this article (links to download the citations from this article in formats compatible with various reference manager tools)
Further reading
-
- Evolutionary Biology
The majority of highly polymorphic genes are related to immune functions and with over 100 alleles within a population, genes of the major histocompatibility complex (MHC) are the most polymorphic loci in vertebrates. How such extraordinary polymorphism arose and is maintained is controversial. One possibility is heterozygote advantage (HA), which can in principle maintain any number of alleles, but biologically explicit models based on this mechanism have so far failed to reliably predict the coexistence of significantly more than ten alleles. We here present an eco-evolutionary model showing that evolution can result in the emergence and maintenance of more than 100 alleles under HA if the following two assumptions are fulfilled: first, pathogens are lethal in the absence of an appropriate immune defence; second, the effect of pathogens depends on host condition, with hosts in poorer condition being affected more strongly. Thus, our results show that HA can be a more potent force in explaining the extraordinary polymorphism found at MHC loci than currently recognized.
-
- Evolutionary Biology
Spatial patterns in genetic diversity are shaped by individuals dispersing from their parents and larger-scale population movements. It has long been appreciated that these patterns of movement shape the underlying genealogies along the genome leading to geographic patterns of isolation by distance in contemporary population genetic data. However, extracting the enormous amount of information contained in genealogies along recombining sequences has, until recently, not been computationally feasible. Here we capitalize on important recent advances in genome-wide gene-genealogy reconstruction and develop methods to use thousands of trees to estimate per-generation dispersal rates and to locate the genetic ancestors of a sample back through time. We take a likelihood approach in continuous space using a simple approximate model (branching Brownian motion) as our prior distribution of spatial genealogies. After testing our method with simulations we apply it to Arabidopsis thaliana. We estimate a dispersal rate of roughly 60km2 per generation, slightly higher across latitude than across longitude, potentially reflecting a northward post-glacial expansion. Locating ancestors allows us to visualize major geographic movements, alternative geographic histories, and admixture. Our method highlights the huge amount of information about past dispersal events and population movements contained in genome-wide genealogies.