Natural variation in stochastic photoreceptor specification and color preference in Drosophila
Abstract
Each individual perceives the world in a unique way, but little is known about the genetic basis of variation in sensory perception. In the fly eye, the random mosaic of color-detecting R7 photoreceptor subtypes is determined by stochastic on/off expression of the transcription factor Spineless (Ss). In a genome-wide association study, we identified a naturally occurring insertion in a regulatory DNA element in ss that lowers the ratio of SsON to SsOFF cells. This change in photoreceptor fates shifts the innate color preference of flies from green to blue. The genetic variant increases the binding affinity for Klumpfuss (Klu), a zinc finger transcriptional repressor that regulates ss expression. Klu is expressed at intermediate levels to determine the normal ratio of SsON to SsOFF cells. Thus, binding site affinity and transcription factor levels are finely tuned to regulate stochastic expression, setting the ratio of alternative fates and ultimately determining color preference.
https://doi.org/10.7554/eLife.29593.001Introduction
Organisms require a diverse repertoire of sensory receptor neurons to perceive a range of stimuli in their environments. Differentiation of sensory neurons often requires stochastic mechanisms whereby individual neurons randomly choose between different fates. Stochastic fate specification diversifies sensory neuron subtypes in a wide array of species including worms, flies, mice, and humans (Ressler et al., 1993; Roorda and Williams, 1999; Troemel et al., 1999; Hofer et al., 2005; Johnston and Desplan, 2010; Magklara and Lomvardas, 2013; Alqadah et al., 2016; Viets et al., 2016). How naturally occurring changes in the genome affect stochastic mechanisms to alter sensory system development and perception is poorly understood. To address this question, we investigated natural variation in stochastic color photoreceptor specification in the Drosophila retina.
The fly eye, like the human eye, contains a random mosaic of photoreceptors defined by expression of light-detecting Rhodopsin proteins (Montell et al., 1987; Bell et al., 2007; Johnston and Desplan, 2010; Viets et al., 2016). In flies, the stochastic on/off expression of Spineless (Ss), a PAS-bHLH transcription factor, determines R7 photoreceptor subtypes. Ss expression in a random subset of R7s induces ‘yellow’ (yR7) fate and expression of Rhodopsin4 (Rh4), whereas the absence of Ss in the complementary subset of R7s allows for ‘pale’ (pR7) fate and Rhodopsin3 (Rh3) expression (Figure 1A) (Wernet et al., 2006; Johnston et al., 2011; Thanawala et al., 2013; Johnston and Desplan, 2014). The on/off state of Ss in a given R7 also indirectly determines the subtype fate of the neighboring R8 photoreceptor. pR7s lacking Ss signal to pR8s to activate expression of blue-detecting Rhodopsin5 (Rh5). yR7s expressing Ss do not send this signal, resulting in expression of green-detecting Rhodopsin6 (Rh6) in yR8s (Figure 1A) (Franceschini et al., 1981; Montell et al., 1987; Zuker et al., 1987; Chou et al., 1996; Huber et al., 1997; Chou et al., 1999a; Mikeladze-Dvali et al., 2005; Mazzoni et al., 2008; Vasiliauskas et al., 2009; Jukam and Desplan, 2011; Hsiao et al., 2013; Johnston, 2013; Jukam et al., 2013; Jukam et al., 2016; Yan et al., 2017).
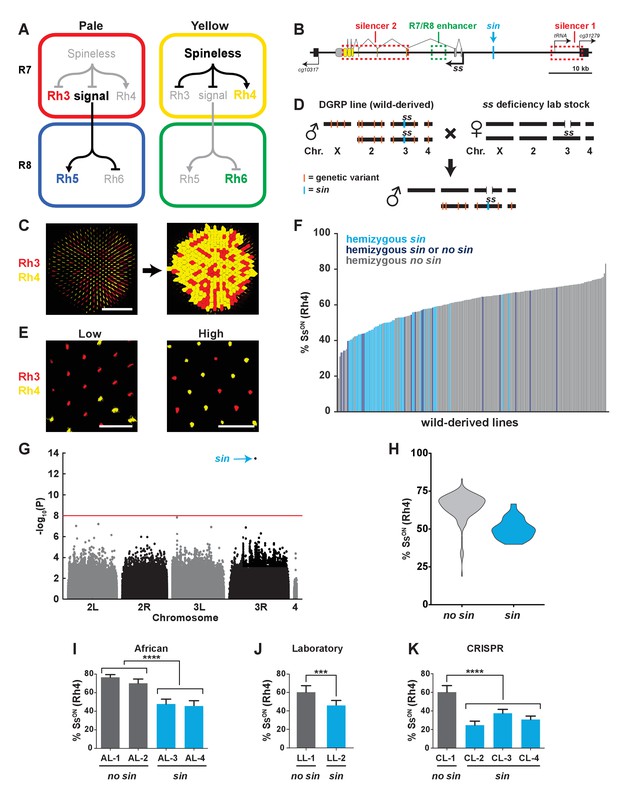
A naturally-occurring single base insertion (sin) in the ss locus lowered the ratio of SsON to SsOFF R7s.
(A) R7 and R8 subtypes are determined by the on/off expression of Spineless (Ss). (Left) The absence of Ss allows Rh3 expression in pale R7s and Rh5 expression in pale R8s. (Right) Expression of Ss induces Rh4 expression in yellow R7s and Rh6 expression in yellow R8s. The signal by which Spineless mediates Rh5 vs. Rh6 expression in R8s is currently unknown. (B) Schematic of the ss locus. Green dashed rectangle indicates R7/R8 enhancer; red dashed rectangles indicate silencer 1 and silencer 2; blue line indicates Klu binding site; blue arrow indicates ss insertion/sin; gray ovals represent untranslated exons; yellow ovals represent translated exons; black boxes indicate neighboring genes; arrows indicate transcriptional starts. See also Figure 1—figure supplement 1. (C) Image of a whole mount fly retina. (Left) Stochastic distribution of R7s expressing Rh3 (SsOFF) or Rh4 (SsON). Scale bar indicates 100 µm. (Right) An automated counting system identified and counted Rh3- and Rh4-expressing R7s. (D) Crossing scheme: Wild-derived DGRP flies were crossed with ss deficiency flies, yielding progeny that were hemizygous at the ss locus. Orange lines indicate hypothetical genetic variants; blue line indicates sin in ss. (E) Representative images from progeny in (D) with low (left; DGRP-397) and high (right; DGRP-229) proportions of SsON (Rh4) R7s. Scale bar indicates 20 µm. (F) SsON proportion varied across DGRP fly lines. sin was enriched in lines with a low proportion of SsON R7s. Each bar represents progeny from a single DGRP line, and bars are arranged in rank order. Light blue bars indicate hemizygous sin. Dark blue bars indicate hemizygous sin or hemizygous no sin (original DGRP line was heterozygous sin/no sin). Gray bars indicate hemizygous no sin. See also Figure 1—source data 1. (G) GWAS identified sin as a genetic variant associated with Ss expression. Manhattan plot of the genetic variant p-values. Genetic variants above the red line (Bonferroni correction) are considered significant. Arrow indicates sin. (H) sin was enriched in lines with a low proportion of SsON R7s. Violin plot of DGRP lines with and without sin. (I–K) Flies with sin displayed a lower proportion of SsON R7s compared to flies without sin. AL indicates African lines; LL indicates laboratory lines; CL indicates lines in which sin was inserted with CRISPR. **** indicates p<0.0001; *** indicates p<0.001. Error bars indicate standard deviation (SD). See also Figure 1—figure supplements 2–4.
-
Figure 1—source data 1
DGRP % SsON phenotypes.
- https://doi.org/10.7554/eLife.29593.007
The stochastic decision to express Ss is made cell-autonomously at the level of the ss gene locus via a random repression mechanism. The R7/R8 enhancer induces ss expression in all R7s, whereas two silencer regions (silencer 1 and 2) repress expression in a random subset of R7s (Figure 1B) (Johnston and Desplan, 2014).
Though the stochastic expression of Ss is binary (i.e. on or off) in individual R7s, it does not result in a simple 50:50 on/off ratio across the population of R7s in a given retina. In most lab stocks, Ss is on in ~65% of R7s and off in ~35% (Figure 1C) (Wernet et al., 2006; Johnston and Desplan, 2014). Here, we find that the proportion of SsON to SsOFF R7s varies greatly among fly lines derived from the wild. We performed a genome-wide association study (GWAS) and identified a single base pair insertion that increases the affinity of a DNA binding site for a transcriptional repressor, significantly reducing the SsON/SsOFF ratio. This genetic variant changes the proportion of photoreceptor subtypes and alters the innate color preference of flies.
Results
sin decreases the ratio of SsON to SsOFF R7s
To determine the mechanism controlling the ratio of stochastic on/off Ss expression, we analyzed the variation in 203 naturally-derived lines collected from Raleigh, North Carolina (Drosophila Genetic Reference Panel (DGRP)) (Mackay et al., 2012). We evaluated Rh4 and Rh3 expression, as they faithfully report Ss expression in R7s (i.e. SsON = Rh4; SsOFF = Rh3) (Figure 1A) (Thanawala et al., 2013; Johnston and Desplan, 2014). To facilitate scoring, we generated a semi-automated counting system to determine the Rh4:Rh3 ratio for each genotype (Figure 1C).
To assess the variation in the DGRP lines attributable to the ss locus and limit the phenotypic contribution of recessive variants at other loci, we crossed each DGRP line to a line containing a ~200 kb deficiency covering the ss locus and analyzed Rh3 and Rh4 expression in the F1 male progeny (Figure 1D). This genetic strategy generated flies hemizygous (i.e. single copy) for the wild-derived ss gene locus, heterozygous wild-derived/lab stock for the second, third, and fourth chromosomes, and hemizygous lab stock for the X chromosome (Figure 1D). While the lab stock expressed Ss (Rh4) in 62% of R7s under these conditions, expression among the DGRP lines varied significantly, ranging from 19% to 83% SsON (Rh4) (Figure 1E–F; Figure 1—source data 1).
To identify the genetic basis of this variation, we performed a genome-wide association study (GWAS) using the SsON (Rh4) phenotype data and inferred full genome sequences of the progeny of each DGRP line crossed with the ss deficiency line. We performed an association analysis and identified a single base pair insertion within the ss locus (‘ss insertion’ or ‘sin’) that was significant (p<10−13) after Bonferroni correction (Figure 1G). sin was enriched in DGRP lines with a low ratio of SsON to SsOFF R7s (Figure 1F and H).
We next confirmed the regulatory role of sin. Naturally derived lines from Africa that are homozygous for sin displayed a decrease in the proportion of SsON (Rh4) R7s compared to lines from Africa lacking sin (Figure 1I) (Lack et al., 2015). We identified sin on a balancer chromosome (TM6B) in a lab stock that similarly displayed a decrease in the proportion of SsON (Rh4) R7s when ss was hemizygous (Figure 1J). To definitively test the role of sin, we used CRISPR to insert sin into a lab stock. Flies hemizygous for CRISPR sin alleles displayed a significant decrease in the proportion of SsON (Rh4) R7s (Figure 1K). Using a Ss antibody, we examined Ss expression directly and found that flies homozygous for CRISPR sin alleles displayed a significant decrease in the proportion of SsON R7s (Figure 1—figure supplement 2A–B and E). Thus, sin causes a decrease in the ratio of SsON to SsOFF R7s.
sin shifts innate color preference from green to blue
As sin alters the proportion of color-detecting photoreceptors, we hypothesized that it would also change color detection and preference. When presented with two light stimuli in a T-maze (Tully and Quinn, 1985), flies will phototax toward the light source that they perceive as more intense (Figure 2A) (McEwen, 1918; Heisenberg and Wolf, 1984; Choe and Clandinin, 2005). The absorption spectra of Rh3 and Rh4 significantly overlap in the UV range (Feiler et al., 1992), complicating behavioral assessment of color preference caused by differences in R7 photoreceptor ratios. Instead, we focused on the perception of blue light by Rh5 and green light by Rh6 in the R8 photoreceptors, as these Rhodopsins have more distinct absorption spectra (Salcedo et al., 1999). Because R8 fate is coupled to R7 fate (Chou et al., 1996) (Figure 1A), we predicted that flies with sin would have a low ratio of Rh6- to Rh5-expressing R8s and would consequently prefer blue light, while flies without sin would have a higher ratio of Rh6- to Rh5-expressing R8s and would instead prefer green light. Indeed, DGRP lines containing sin preferred blue light, while DGRP lines lacking sin preferred green light (Figure 2A–C; Figure 2—source data 1), showing that sin changes innate color preference in flies. Natural variation in the gene regulatory pathway controlling Rh5 and Rh6 expression downstream of Ss (Mikeladze-Dvali et al., 2005; Jukam and Desplan, 2011; Jukam et al., 2013; Viets et al., 2016) or in the neural circuit downstream of R8 signaling likely caused the green light preference of some lines with sin.
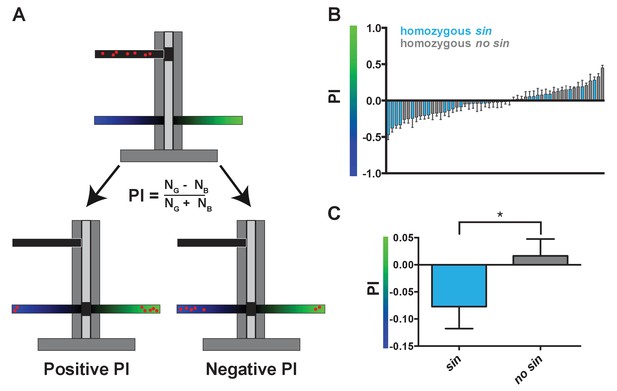
Wild-derived flies with sin display a shift in innate color preference from green to blue.
(A) Schematic of T-maze apparatus. Red dots represent flies. PI = Preference Index. Positive PI indicates preference for green light; negative PI indicates preference for blue light. NG: number of flies on green side; NB: number of flies on blue side. (B–C) Flies with sin preferred blue light, while flies without sin preferred green light. Color of bar indicates genotype of DGRP line. Light blue bars indicate homozygous sin. Gray bars indicate homozygous no sin. Error bars indicate standard error of the mean (SEM). See also Figure 2—source data 1. (B) PIs for individual DGRP lines with and without sin. (C) Averages of PIs for DGRP lines with (n = 23) and without sin (n = 31). * indicates p<0.05.
-
Figure 2—source data 1
DGRP behavior phenotypes.
- https://doi.org/10.7554/eLife.29593.009
sin increases the binding affinity for the Klumpfuss transcription factor
sin is a single base pair insertion within a previously uncharacterized non-coding region of the ss locus located ~7 kb upstream of the transcriptional start (Figure 1B and Figure 3A). To identify trans factors whose binding might be affected by sin, we searched for binding motifs affected by sin in SELEX-seq (Nitta et al., 2015) and bacterial one-hybrid datasets (B1H) (Zhu et al., 2011; Enuameh et al., 2013). sin lies in a predicted binding site for the zinc finger transcription factor Klumpfuss (Klu), the fly homolog of Wilms’ Tumor Suppressor Protein 1 (WT1) (Figure 3B, Figure 3—figure supplement 1A) (Klein and Campos-Ortega, 1997; Yang et al., 1997). This region is perfectly conserved across 21 Drosophila species covering 50 million years of evolution, consistent with a critical regulatory role (Figure 3C, Figure 3—figure supplement 1B–C).
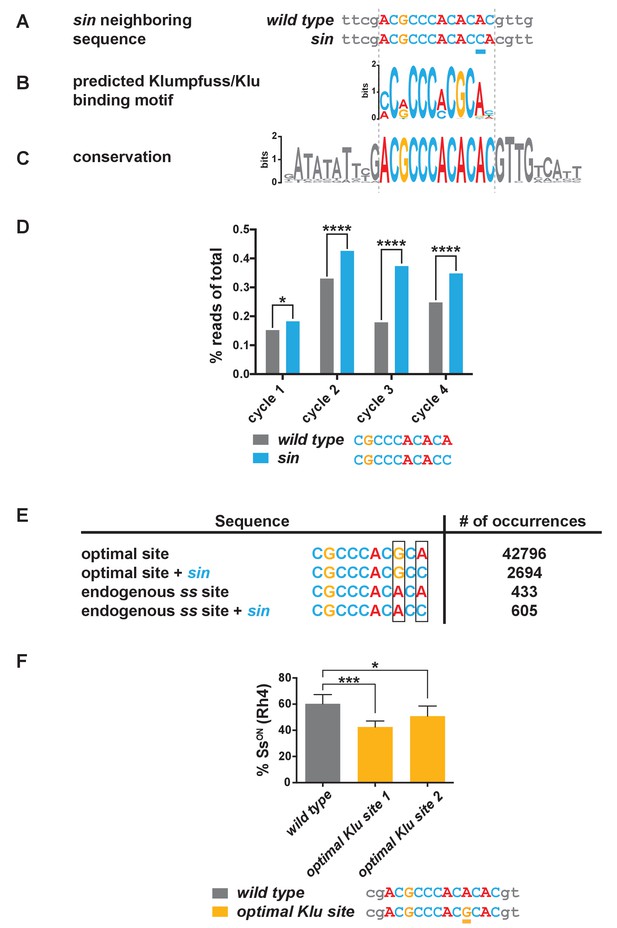
sin increases the binding affinity for the transcription factor Klumpfuss.
(A–C) Colored bases indicate the predicted Klu binding site. (A) sin is a single base pair insertion of a C at Chr. 3R: 16,410,775 (release 6). Underline indicates sin. (B) Position weight matrix (PWM) for Klu binding predicted from SELEX-seq. See also Figure 3—figure supplement 1A. (C) The Klu site is perfectly conserved across 21 species of Drosophila covering 50 million years of evolution. Conservation logo of the Klu site and neighboring sequence in the ss locus. Height of bases indicates degree of conservation. See also Figure 3—figure supplement 1B. (D) sin increased Klu binding affinity in vitro. Quantification of the number of reads for the Klu site with and without sin in four cycles of SELEX-seq. * indicates p<0.05; **** indicates p<0.0001. (E) Comparing the number of read-occurrences in the SELEX-seq dataset, sin increases binding of Klu to the endogenous ss site but decreases binding of Klu to the optimal site, suggesting a binding site affinity dependence between bases. Boxes highlight positions 8 and 10 in the 10-mer core sequences. (F) Flies hemizygous for an optimal Klu site displayed a lower proportion of SsON R7s compared to wild type flies. optimal Klu site 1 and optimal Klu site 2 are independent lines derived from CRISPR-mediated mutagenesis. * indicates p<0.05; *** indicates p<0.001. Error bars indicate standard deviation (SD).
To evaluate the effect of sin on Klu binding, we analyzed available SELEX-seq binding data (Nitta et al., 2015), focusing on the core 10-mer. The number of reads containing the Klu binding site with sin (CGCCCACACC) was significantly higher than without sin (CGCCCACACA) (Figure 3D), and thus, Klu binds the endogenous ss sequence with sin better than without it. Considering the frequency of 10-mers as a measure of site preference, we found that 506 10-mers (0.10%) have frequencies greater than the Klu site without sin, whereas only 366 10-mers (0.07%) have frequencies greater than the Klu site with sin. Together, sin increases the binding affinity of the Klu site in vitro.
We further analyzed SELEX-seq data to understand the differences between Klu binding affinities for the predicted optimal site and endogenous site in ss. The endogenous 10-mer core sequence in ss (CGCCCACACA) deviates from the optimal site (CGCCCACGCA) at position 8, causing a dramatic reduction in the affinity for the endogenous site (Figure 3E). Interestingly, sin in position 10 significantly decreases binding affinity for the optimal site (compare CGCCCACGCA to CGCCCACGCC), whereas it increases affinity for the endogenous Klu site in ss (compare CGCCCACACA to CGCCCACACC) (Figure 3E). A PWM is a good representation of the sequence preferences of a DNA-binding protein, but it assumes independent contributions of individual bases. In this case, we observe dependence between positions within the motif that the PWM disregards. Our analysis indicates that Klu binding affinity is dependent on the relationship between the bases in position 8 and 10. This dependence reveals that Klu preferentially interacts with the site with sin (C in position 10) over the site without sin (A in position 10) in the endogenous spineless locus (A in position 8), in contrast to the general predictions of the PWM (preferred G in position eight and A in position 10). Dependence between positions suggests that binding of transcription factors like Klu is determined not only by sequence but also by DNA shape, as has been described previously (Abe et al., 2015; Zhou et al., 2015; Chiu et al., 2017). These data suggest that the Klu site in the endogenous locus is a low-affinity site and that sin increases its affinity.
Since sin is predicted to increase the binding affinity for Klu and sin caused a reduction in the on/off ratio of Ss expression, we hypothesized that mutating the Klu site to an optimized high-affinity site would also cause a decrease in the proportion of SsON R7s. We used CRISPR to mutate the endogenous low-affinity Klu site (ACGCCCACACAC) to the predicted optimized high-affinity site (ACGCCCACGCAC) and observed a decrease in the proportion of SsON R7s similar to flies with sin (Figure 3F). The observation that an optimized high-affinity Klu site causes a similar phenotype as sin is consistent with the conclusion that sin increases the binding affinity for Klu.
Klu lowers the SsON/SsOFF ratio in R7s
Klu/WT1 has been shown to be a transcriptional repressor in other systems (Drummond et al., 1992; McDonald et al., 2003; Kaspar et al., 2008). As sin decreases Ss expression frequency and is predicted to increase Klu binding affinity, we hypothesized that Klu also represses stochastic ss expression in R7s. We found that Klu was expressed in R7s in larval eye imaginal discs in a Gaussian distribution (Figure 4A–B; Figure 4—figure supplement 1) (Wildonger et al., 2005). We predicted that increasing Klu levels would cause a decrease in the proportion of SsON R7s, whereas decreasing or completely ablating Klu would cause an increase in the proportion of SsON R7s. Indeed, increasing the levels of Klu in Klu-expressing cells (klu > klu), all photoreceptors (eye > klu), or specifically in all R7s (R7 > klu) caused a decrease in the proportion of SsON (Rh4) R7s (Figure 4C–D; Figure 1—figure supplement 2C and E). This decrease in the SsON/SsOFF ratio upon increasing Klu levels mimicked the effect of sin, consistent with sin increasing the binding affinity for the Klu repressor.
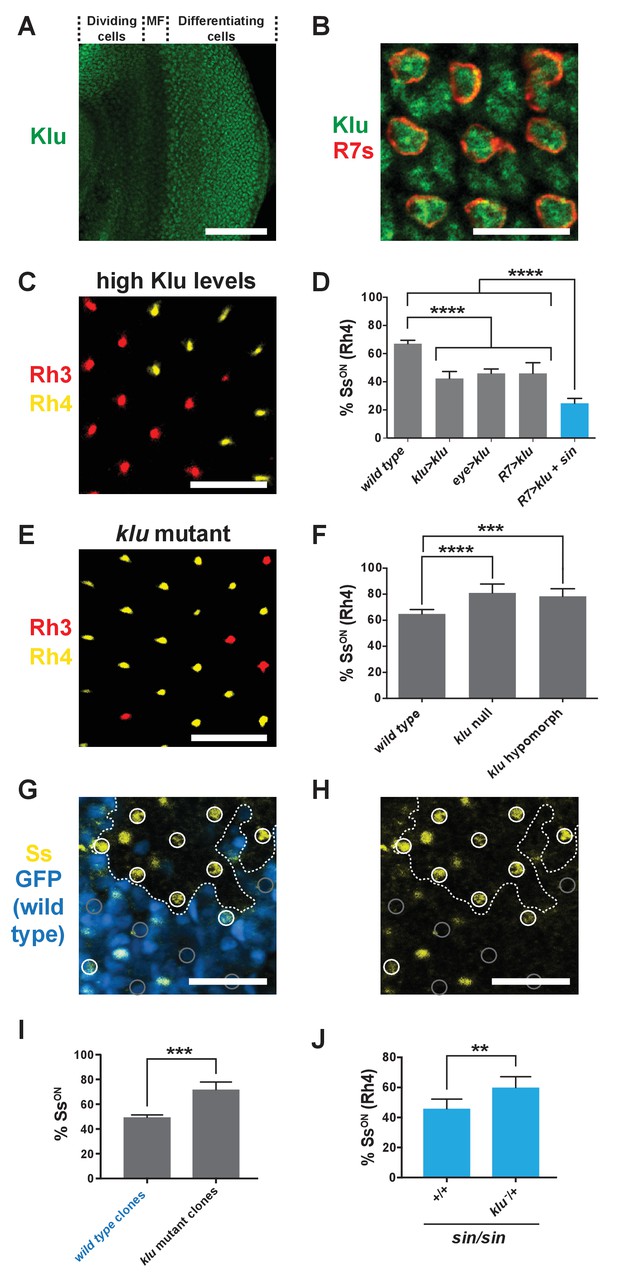
Levels of Klu determine the ratio of SsON/SsOFF R7s.
(A) Klu was expressed in the developing larval eye disc. MF indicates morphogenetic furrow. Scale bar indicates 100 µm. (B) Klu was expressed in all R7s in the developing larval eye disc. Red indicates R7s marked by pm181 >Gal4, UAS > mCD8 GFP. Scale bar indicates 10 µm. See also Figure 4—figure supplement 1. (C–D) Increasing Klu levels decreased the proportion of SsON R7s. Increasing Klu levels in flies with sin caused an additional reduction in the proportion of SsON R7s. In C, representative image of a retina from an eye > klu fly. R7s express either Rh3 (SsOFF) or Rh4 (SsON). Scale bar indicates 20 µm. In D, **** indicates p<0.0001. Error bars indicate standard deviation (SD). (E–F) klu loss-of-function mutants displayed increases in the proportion of SsON R7s. In E, representative image of a retina from a klu hypomorph. R7s express either Rh3 (SsOFF) or Rh4 (SsON). Scale bar indicates 20 µm. In F, **** indicates p<0.0001; *** indicates p<0.001. Error bars indicate standard deviation (SD). (G–H) The proportion of SsON R7s was higher in klu null mutant clones compared to wild type clones in mid-pupal retinas. GFP+ indicates wild type clone; GFP- indicates klu mutant clone. The dotted line marks the clone boundary. White circles indicate SsON R7s; gray circles indicate SsOFF R7s. In addition to expression in circled R7s, Ss was expressed in bristle cells (unmarked). Scale bar indicates 20 µm. (I) Quantification of % SsON R7s in klu null mutant and wild type clones. *** indicates p<0.001. Error bars indicate standard deviation (SD). (J) Decreasing klu gene dosage in klu null mutant heterozygotes suppressed the sin phenotype. ** indicates p<0.01. Error bars indicate standard deviation (SD).
Conversely, klu loss-of-function mutants displayed increases in the proportion of SsON (Rh4) R7s (Figure 4E–F). We examined Ss expression directly and found that the proportion of SsON R7s increased in klu null mutants (Figure 1—figure supplement 2D–E). Moreover, we found that the proportion of SsON R7s increased in klu mutant clones compared to wild type clones (Figure 4G–I). As the proportion of SsON R7s increases specifically in klu mutant clones and decreases upon ectopic expression of Klu in R7s, we conclude that Klu is endogenously expressed at intermediate levels and acts cell-autonomously to determine Ss expression state.
Our data suggest that the ratio of Ss on/off gene expression is controlled by both the level of Klu protein and the binding affinity of the Klu site. To test this idea, we altered Klu levels in flies with the higher affinity Klu site (i.e. with sin). Because the proportion of SsON R7s is reduced in flies with increased Klu levels (high repressor levels) or in flies with the sin variant (high binding affinity), we predicted a further reduction in flies with both high Klu and sin (high repressor levels, high binding affinity). We generated flies with increased levels of Klu in a sin genetic background and observed a significant additional reduction in the proportion of SsON R7s (Figure 4D).
To further test the relationship between Klu levels and binding site affinity, we reduced klu gene dosage in flies with sin and found that the sin phenotype was suppressed in klu mutant heterozygotes (Figure 4J). We conclude that sin increases Klu binding affinity and that the binding affinity of the Klu site and levels of Klu protein determine the proportion of SsON R7s.
Discussion
Our studies of wild-derived flies revealed significant variation in stochastic Ss expression. We identified sin, a single base pair insertion in the ~60 kb ss locus that dramatically lowers the SsON/SsOFF ratio by increasing the binding affinity for the transcriptional repressor Klu. This decrease in Ss expression frequency changes the proportion of color-detecting photoreceptors and alters innate color preference in flies.
sin appears to be a relatively new mutation in D. melanogaster populations. sin is absent among diverse drosophilid species spanning millions of years of divergence (Figure 3—figure supplement 1B–C) and is segregating at an extremely low frequency among non-admixed African D. melanogaster lineages (Figure 1—figure supplement 3A–F). sin likely rose to intermediate frequencies following D. melanogaster’s colonization of Europe about 10–15 thousand years ago (Li and Stephan, 2006). sin continues to segregate at intermediate frequencies amongst North American populations (Figure 1—figure supplement 3A–F), which were established within the last 150 years from mixtures of European and African populations (Bergland et al., 2016). The recent rise in the frequency of sin suggests that it could be the target of natural selection, perhaps via modulation of innate color preference. We tested this model by assessing patterns of allele frequency differentiation among populations sampled worldwide and by examining haplotype homozygosity surrounding sin. We compared these statistics at sin to the distribution of statistics calculated from several thousand randomly selected 1–2 bp indel polymorphisms that segregate at ~25% in the DGRP. Curiously, sin did not deviate from genome-wide patterns (Figure 1—figure supplement 3G–J) suggesting that it might be selectively neutral in contemporary D. melanogaster populations.
It is interesting that Rhodopsin expression varies so significantly in the wild, given the nearly invariant hexagonal lattice of ommatidia in the fly eye. Rhodopsins are G-protein coupled receptors (GPCRs), a class of proteins identified as a source of natural behavioral variation in worms, mice, and voles (Young et al., 1999; Yalcin et al., 2004; Bendesky et al., 2011). Dramatic differences in Rhodopsin expression patterns across insect species (Hilbrant et al., 2014; Wernet et al., 2015) suggest that variation in the expression of GPCRs, rather than retinal morphology, may allow rapid evolution in response to environmental changes.
sin increases the binding affinity of a conserved Klu site, suggesting that the site is suboptimal or low-affinity for Klu binding. Low-affinity sites ensure the timing and specificity of gene expression (Jiang and Levine, 1993; Gaudet and Mango, 2002; Scardigli et al., 2003; Rowan et al., 2010; Ramos and Barolo, 2013; Crocker et al., 2015; Farley et al., 2015; Crocker et al., 2016). Our studies reveal a critical role for a low-affinity binding site in the regulation of a stochastically expressed gene. The suboptimal Klu site, bound by endogenous levels of Klu, yields the normal 65:35 SsON/SsOFF ratio. Changing the affinity of the site or the level of Klu alters the ratio of SsON/SsOFF cells. We conclude that stochastic on/off gene expression is controlled by threshold levels of trans factors binding to low-affinity sites.
The level of Klu (analog input) determines the binary on/off ratio of Ss expression (digital output). In contrast, gene regulation is best understood in cases where levels of transcription factors (analog input) regulate the levels of target gene expression (analog output). Interestingly, sin or genetic perturbation of klu affected the frequency of Ss expression (Figures 1F, I–K and 4C–J, Figure 1—figure supplement 2) but not levels (Figure 1—figure supplement 4).
The on/off nature of Ss expression suggests a cooperative mechanism whereby Klu acts with other factors to regulate ss. Conservation of additional base pairs surrounding the Klu site (Figure 3C) is consistent with cooperative binding of Klu and others factors, possibly through dimerization or multimerization. These additional conserved base pairs could also enable binding of activating transcription factors to sites that overlap with the Klu site. These activating transcription factors may compete with the repressor Klu for binding to determine the stochastic on/off expression state of ss.
The expression state of ss could be determined by the intrinsic variation in Klu levels (Figure 4—figure supplement 1). In this model, if Klu levels exceed a threshold, ss is off, and if Klu levels are below the threshold, ss is on. Alternatively, Klu levels could set the threshold for a different gene regulatory mechanism, such as DNA looping or heterochromatin spreading. The regions encompassing and neighboring the Klu binding site drive gene expression in the eye (Figure 1—figure supplement 1), suggesting that complex interactions between this regulatory DNA element, the R7/R8 enhancer, and the two silencers (Figure 1B) ultimately control the ss on/off decision.
Cell fate specification is commonly thought of as a reproducible process whereby cell types uniformly express specific batteries of genes. This reproducibility is often the result of high levels of transcription factors binding to high-affinity sites, far exceeding a regulatory threshold, yielding expression of target genes in all cells of a given type. In contrast, the stochastic on/off expression of Ss requires finely tuned levels of regulators binding to low-affinity sites. We predict that fine tuning of binding site affinities and transcription factor levels will emerge as a common mechanistic feature that determines the ratio of alternative fates in stochastic systems.
Materials and methods
Drosophila genotypes and stocks
Request a detailed protocolFlies were raised on standard cornmeal-molasses-agar medium and grown at 25°C.
Short genotype | Complete genotype | Figures | Source |
---|---|---|---|
lab stock or wild type | yw; sp/CyO;+ or + ; +; + | 1C, 4D, 4F, Figure 1—figure supplements 2A, E and 4D–E | |
Low | w1118/Y; DGRP-397 / +; DGRP-397/Df(3R)Exel6269 | 1E | DGRP (Mackay et al., 2012) (Parks et al., 2004) |
High | w1118/Y; DGRP-229 / +; DGRP-229/Df(3R)Exel6269 | 1E | DGRP (Mackay et al., 2012) |
Wild-derived lines | w1118/Y; DGRP / +; DGRP/Df(3R)Exel6269 | 1F, Figure 1—source data 1 | DGRP (Mackay et al., 2012) |
African Line No sin (AL-1) | KN34; KN34; KN34 | 1I | (Lack et al., 2015) |
African Line No sin (AL-2) | KR42; KR42; KR42 | 1I | (Lack et al., 2015) |
African Line sin (AL-3) | KN133N; KN133N; KN133N | 1I | (Lack et al., 2015) |
African Line sin (AL-4) | KR7; KR7; KR7 | 1I | (Lack et al., 2015) |
Lab Line No sin (LL-1) | yw; +; +/Df(3R)Exel6269 | 1J | |
Lab Line sin (LL-2) or sin/ss df | yw; +; TM6B (with sin)/Df(3R)Exel6269 | 1J, Figure 1—figure supplement 4E | |
CRISPR No sin(CL-1) | yw; +; CRISPR negative/Df(3R)Exel6269 | 1K | |
CRISPR sin (CL-2) | yw; +; CRISPR positive 1/Df(3R)Exel6269 | 1K | |
CRISPR sin (CL-3) | yw; +; CRISPR positive 2/Df(3R)Exel6269 | 1K | |
CRISPR sin (CL-4) | yw; +; CRISPR positive 3/Df(3R)Exel6269 | 1K | |
Innate color preference | DGRP; DGRP; DGRP | 2, Figure 2—source data 1 | DGRP (Mackay et al., 2012) |
Wild type (control for optimal Klu site) | yw; +; +/Df(3R)Exel6269 | 3E | |
CRISPR Optimal Klu site 1 | yw; +; CRISPR positive 1/Df(3R)Exel6269 | 3E | |
CRISPR Optimal Klu site 2 | yw; +; CRISPR positive 2/Df(3R)Exel6269 | 3E | |
Klu expression in larval eye imaginal discs | yw; pm181 > Gal4, UAS > mCD8 GFP/CyO; + | 4A, 4B | |
klu > klu | yw; +; klu > Gal4/UAS > klu | 4D | (Klein and Campos-Ortega, 1997) |
eye > klu | yw; +; lGMR > Gal4/UAS > klu | 4C, 4D, Figure 1—figure supplement 2C and E, 4E | (Wernet et al., 2003) |
R7 > klu | yw; panR7 > Gal4 / +; UAS > klu/+ | 4D | (Chou et al., 1999b1999) |
Wild type (control for klu mutants) | yw; ey > Gal4, UAS > flp/+; GMR > hid, cL FRT2A/ubi > GFP FRT2A | 4F | |
klu null | yw; ey > Gal4, UAS > flp/+; GMR > hid,cL FRT2A/kluRR51 FRT2A | 4F, Figure 1—figure supplement 2D–E, Figure 1—figure supplement 4E | (Klein and Campos-Ortega, 1997; Stowers and Schwarz, 1999) |
klu hypomorph | yw; ey > Gal4, UAS > flp/+; GMR > hid, cL FRT2A/kluPBacPBac LL02701FRT2A FRT82b P[Car20y] | 4E, 4F | (Stowers and Schwarz, 1999; Schuldiner et al., 2008) |
klu null mutant clones | ey > flp; +/CyO; kluR51 FRT2A/ubi > GFP FRT2A | 4G-I | |
+/+, sin/sin | +; +; sin | 4J, Figure 1—figure supplement 2B and E | |
klu-/+, sin/sin | +; +; kluR51 FRT2A, sin / +, sin | 4J | |
R7 reporter | yw; pm181 > Gal4, UAS > mCD8 GFP/CyO; + | Figure 4—figure supplement 1 | |
14 F02 | w1118; P{GMR14F02-GAL4}attP2 / +; UAS > H2B:YFP / + | Figure 1—figure supplement 1A–B | (Jenett et al., 2012) |
15 F08 | w1118; P{GMR15F08-GAL4}attP2 / +; UAS > H2B:YFP / + | Figure 1—figure supplement 1A and C | (Jenett et al., 2012) |
15 F02 | w1118; P{GMR15F02-GAL4}attP2 / +; UAS > H2B:YFP / + | Figure 1—figure supplement 1A and D | (Jenett et al., 2012) |
Antibodies
Antibodies were used at the following dilutions: mouse anti-Rh3 (1:100) (gift from S. Britt, University of Colorado), rabbit anti-Rh4 (1:100) (gift from C. Zuker, Columbia University), rat anti-Klu (1:200) (gift from C. Desplan, New York University), guinea pig anti-Ss (1:500) (gift from Y.N. Jan, University of California, San Francisco), sheep anti-GFP (1:500) (BioRad, Hercules, CA, USA), rat anti-ELAV (1:50) (DSHB, Iowa City, Iowa, USA), mouse anti-Pros (1:10) (DSHB), and Alexa 488 Phalloidin (1:80) (Invitrogen, Thermo Fisher Scientific, Waltham, MA, USA). All secondary antibodies were Alexa Fluor-conjugated (1:400) and made in donkey (Molecular Probes).
Antibody staining
Request a detailed protocolAdult, mid-pupal, and larval retinas were dissected as described (Hsiao et al., 2012) and fixed for 15 min with 4% formaldehyde at room temperature. Retinas were rinsed three times in PBS plus 0.3% Triton X-100 (PBX) and washed in PBX for >2 hr. Retinas were incubated with primary antibodies diluted in PBX overnight at room temperature and then rinsed three times in PBX and washed in PBX for >4 hr. Retinas were incubated with secondary antibodies diluted in PBX overnight at room temperature and then rinsed three times in PBX and washed in PBX for >2 hr. Retinas were mounted in SlowFade Gold Antifade Reagent (Invitrogen). Images were acquired using a Zeiss LSM 700 confocal microscope.
Quantification of expression
Request a detailed protocolFrequency of Rh3 (SsOFF) and Rh4 (SsON) expression in R7s was scored in adults. Six or more retinas were scored for each genotype (N). 100 or more R7s were scored for each retina (n). Frequency was assessed using custom semi-automated software (see below) or manually.
Frequency of Ss expression in R7s was assessed with a Ss antibody in mid-pupal animals. Four or more retinas were scored for each genotype (N). 70 or more R7s were scored for each retina (n). Frequency was assessed manually.
Levels of Ss expression in SsON R7s were assessed with a Ss antibody in mid-pupal animals. Three retinas were scored (N). 40 or more SsON R7s were scored for each retina (n). We used ImageJ software to quantify Ss levels in SsON R7s (Figure 1—figure supplement 4A–C). A circular ‘‘region of interest’’ was manually placed at the center of each SsON R7 (identified by expression of Ss and the R7 marker Prospero) to avoid signal from neighboring photoreceptors. ImageJ software assessed the mean pixel intensity for each region of interest for each SsON R7.
Levels of Klu expression in R7s were assessed with a Klu antibody in third instar larval animals. Five retinas were scored (N). 65 or more R7s were scored for each retina (n). We used ImageJ software to quantify Klu levels in all R7s (Figure 4—figure supplement 1). A circular ‘‘region of interest’’ was manually placed at the center of each R7 (identified by pm181 >GAL4; UAS > GFP reporter expression) to avoid signal from neighboring photoreceptors. ImageJ software assessed the mean pixel intensity for each region of interest for each R7.
To determine the number of rows from the equator to the dorsal third region of the adult retina, we first used phalloidin to stain actin (marking rhabdomeres of ommatidia) to locate the equator of each retina. We then counted the number of rows from the equator to the first R7 cell with co-expression of Rh3 and Rh4.
Image processing
Request a detailed protocolWe employed a custom algorithm to identify the positions of individual R7 photoreceptors within an image of the fly retina. First, individual fluorescence images from each wavelength channel were denoised using a homomorphic filter (Oppenheim et al., 1968) and Gaussian blur. Next, R7 boundaries were located using the Canny edge detection method (Canny, 1986). Cells were then roughly segmented using the convex hull algorithm (Barber et al., 1996). Active contouring (Chan and Vese, 2001) was used to refine the segments to fit the R7s more closely. Finally, a watershed transform was applied to the image, dividing it into regions that each contain a single R7. Regions were excluded by size or distance from the center to prevent artifacts due to the curvature of the fly retina. For the remaining regions, normalized intensities from the Rh3 and Rh4 channels were compared in order to assign each region a label, indicating that its R7# is stained with Rh3 or Rh4. A MATLAB (The MathWorks, Inc.) script that implements our algorithm is available at https://app.assembla.com/spaces/roberts-lab-public/wiki/Fly_Retina_Analysis.
Genome-Wide association studies
Request a detailed protocolGenotype data from the DGRP freeze two lifted to the dm6/BDGP6 release of the D. melanogaster genome was obtained from (ftp://ftp.hgsc.bcm.edu/DGRP/). Phenotypes were calculated for the progeny of crosses of DGRP lines and Df(3R)Exel6269 flies. To estimate genotypes of these flies from the DGRP data, we simulated each cross. For each SNP or indel variant in the DGRP genotype data, we assigned a new genotype: (1) homozygous reference remains homozygous reference, (2) homozygous alternate maps to homozygous alternate if in deficiency region, otherwise heterozygous, and (3) all other genotypes mapped to missing or unknown and not included in subsequent analyses. We performed quantitative trait association analysis using plink2 --linear (version 1.90 beta 25 Mar 2016; PMID:25722852). To reduce the impact of population structure, we included the first 20 principal components of the standardized genetic relationship matrix as covariates (calculated using plink2 --pca). To empirically correct p-values for each site, we performed a max(T) permutation test with 10,000 permutations (mperm option to plink2).
CRISPR-mediated mutagenesis
Request a detailed protocolsin was inserted into a lab stock line using CRISPR (Gratz et al., 2013; Port et al., 2014). Sense and antisense DNA oligos for the forward and reverse strands of the gRNA were designed to generate BbsI restriction site overhangs. The oligos were annealed and cloned into the pCFD3 cloning vector (Addgene, Cambridge, MA). A single stranded DNA homology bridge was generated with 60 bp homologous regions flanking each side of the predicted cleavage site. The gRNA construct (500 ng/ul) and homology bridge oligo (100 ng/ul) were injected into Drosophila embryos (BestGene, Inc.). Single males were crossed with a balancer stock (yw; +; TM2/TM6B), and F1 female progeny were screened for the insertion via PCR and sequencing. Single F1 males whose siblings were sin-positive were crossed to the balancer stock (yw; +; TM2/TM6B) and the F2 progeny were screened for the insertion via PCR and sequencing. sin-negative flies from a single founder were used to establish a stable stock (CL-1) and sin-positive flies from three founders were used to establish independent stable stocks (CL-2, CL-3, CL-4).
Oligo name | Sequence |
---|---|
Homologous bridge | TCTCTCTCTCTCTCTGTGTGTGTGTCACTCACAAATGACAACGTGGTGTGGGCGTCGAATATATGAGTTACTTCGCACCCAGCCAGCCAAGCCAGAGCAAATTGAGCCAAACCAAAGCAAA |
gRNA F | GTCGTGTGTGGGCGTCGAATATAT |
gRNA R | AAACATATATTCGACGCCCACACA |
Genotype F | GCCACCCTTCGACCATTTTGG |
Genotype R | GTCAGCCACTACATGGTTTCG |
The Klu optimal site was generated in a lab stock line using CRISPR (Gratz et al., 2013; Port et al., 2014). CRISPR was performed with the same gRNA and genotyping primers as described above, but with a new homologous bridge donor.
Oligo name | Sequence |
---|---|
Homologous bridge | TCTCTCTCTCTCTCTGTGTGTGTGTCACTCACAAATGACAACGTGCGTGGGCGTCGAATATATGAGTTACTTCGCACCCAGCCAGCCAAGCCAGAGCAAATTGAGCCAAACCAAAGCAAA |
gRNA F | GTCGTGTGTGGGCGTCGAATATAT |
gRNA R | AAACATATATTCGACGCCCACACA |
Genotype F | GCCACCCTTCGACCATTTTGG |
Genotype R | GTCAGCCACTACATGGTTTCG |
T-maze behavioral assays
Request a detailed protocolAdult flies were raised on standard medium on a 14 hr/10 hr light and dark cycle at 25°C. The behavioral assay room was illuminated by a 630 nm red LED bulb (superbrightleds.com; PAR30IP-x8-90) whose emitted light lies outside of the sensitivity spectrum for fly photodetection. For each trial, 100 female flies were starved for 8 hr and then inserted into the elevator of the T-maze (Robert Eifert, Bayshore, NY). The elevator was lowered to a junction that, on each side, held an unused plastic tube (Falcon 352017). The T-maze was covered in black chalkboard tape and the plastic tubes were painted black. The T-maze and lights were kept a constant distance apart by a custom 3D-printed holder. A blue LED light (450 nm) and a green LED light (525 nm) on opposite sides were simultaneously turned on. Blue and green LED lights were obtained from superbrightleds.com (E12-B5, E12G5). The blue light was covered with three layers of 3x neutral density (ND) filters, while the green light was covered with one layer. After 20s, the lights were turned off and the tubes were removed and capped. Flies from each tube were counted and the preference index (PI) was calculated using the formula PI = (NG – Nb) / (NG + Nb), where NG equals the number of flies in the tube illuminated with green light and Nb equals the number of flies in the tube illuminated with blue light. PI ranged from −1 to 1, with negative values indicating a blue preference and positive values indicating a green preference. Five or more trials were conducted for each genotype (N). 100 or more flies were scored for each trial (n).
Consensus sequence
Request a detailed protocolFor the B1H data sets, WebLogo3 was used to generate position weight matrices (PWMs) (Zhu et al., 2011; Enuameh et al., 2013)(Figure 3—figure supplement 1A). For the SELEX-SEQ data sets, MEME-ChIP version 4.11.2 was used to generate PWMs (Machanick and Bailey, 2011; Nitta et al., 2015) (ENA: ERX606541-ERX606544). Motif discovery and enrichment mode was set to normal, and the 1st order model of sequences was used as the background model. Expected motif site distribution was set at zero or one occurrence per sequence. Minimum width of motifs to be found by MEME was set to 12, and the max was set to 20.
Conservation analysis
Request a detailed protocolThe Klu site and neighboring sequences for 21 Drosophila species were obtained from the UCSC genome browser. TOMTOM version 4.11.2. was used to generate the conservation PWM (Gupta et al., 2007)(Figure 3C, Figure 3—figure supplement 1B).
SELEX-seq analysis
Request a detailed protocolSELEX-seq datasets from (Nitta et al., 2015) were obtained from ENA (ERX606541-ERX606544). For read-level analysis, we counted the number of reads containing the Klu binding site with sin, without sin, and neither site (there were no reads with both sites). We performed McNemar's test to assess significance. We computed the frequency of each 10-mer within each dataset using Jellyfish version 2.2.6 (Marçais and Kingsford, 2011). Using these counts, we determined the number of 10-mers with frequency greater than that of the Klu binding site with and without sin. Frequencies reported are for the combination of all four SELEX datasets.
Jellyfish 2.2.6 was used to canonically count kmers of length 10 with an initial hash of size 100M from fasta files generated from the first and fourth rounds of selection. Kmers were reverse complemented as necessary to minimize the hamming distance from the consensus sequence. Reported counts come from the fourth round.
Population genetic analyses
Request a detailed protocolWe estimated allele frequencies from populations sampled worldwide at sin and at other 1–2 bp indel polymorphisms. Allele frequency estimates based on pooled resequencing of populations sampled in North America and Europe were obtained from (Bergland et al., 2014) and (Kapun et al., 2016). Allele frequencies based on haplotypes (Lack et al., 2016) were also obtained from populations sampled in North America, the Caribbean, Europe, and Africa.
For pooled samples, we mapped raw sequence reads to Release 6 of the Drosophila melanogaster genome, removed PCR duplicates, performed indel-realignment using GATK version, and called allele frequencies using VarScan. For haplotype data, we relied on published indel VCF files obtained from the Drosophila Genome Nexus (DGN; http://www.johnpool.net/genomes.html). Regions of admixture in African genomes were identified based on analyses by Lack et al., 2016. DGN data were mapped to Release 5 of the Drosophila genome and we converted those coordinates to Release six using the lift-over file available from the UCSC Genome browser (http://hgdownload.soe.ucsc.edu/goldenPath/dm3/liftOver/dm3ToDm6.over.chain.gz).
We sought to assess whether the distribution of sin among populations sampled worldwide was significantly different than expected by chance based on other comparable indel polymorphisms. sin was originally identified in the Drosophila Genetic Reference Panel (DGRP), derived from a population in Raleigh, NC, where it segregates at ~25%. We observed that sin segregates at ~10–25% in other North American and European populations but is rare/absent in ancestral African populations (Figure 1—figure supplement 3A–F). Such changes in allele frequency among continents could indicate the action of positive selection. To test this model, we identified ~1500 other 1–2 bp autosomal indel polymorphisms that segregate at 25 ± 5% in the DGRP (hereafter, ‘control set’). We estimated FST among continents as well as within North America at sin and our control set. FST values were rank normalized and converted to a Z-score through an inverse normal CDF with mean zero and standard deviation one. sin did not show elevated levels of FST within or between continents relative to the control set, suggesting that sin does not contribute to local adaptation amongst sampled populations (Figure 1—figure supplement 3G–H).
Next, we tested whether haplotype patterns surrounding sin are indicative of a partial selective sweep. We calculated the extended haplotype homozogosity (EHH) score and integrated EHH (iEHH) score for haplotypes with and without sin (derived and ancestral haplotypes, respectively) in the DGRP data where sin was originally identified. EHH scores were also calculated at the control set, as described above. EHH scores were calculated using the R package rehh (Gautier and Vitalis, 2012). The derived sin allele shows an elevated iEHH score compared to the ancestral allele, suggestive of a partial selective sweep (Figure 1—figure supplement 3I–J). To test this model, we calculated the integrated haplotype statistic as,
for sin as well as the control set. IHS for sin is not significantly different than expected by chance relative to other comparable indel polymorphisms (Figure 1—figure supplement 3I–J).
References
-
Stochastic left-right neuronal asymmetry in Caenorhabditis elegansPhilosophical transactions of the Royal Society of London. Series B, Biological sciences 371:.https://doi.org/10.1098/rstb.2015.0407
-
The quickhull algorithm for convex hullsACM Transactions on Mathematical Software 22:469–483.https://doi.org/10.1145/235815.235821
-
Two types of Drosophila R7 photoreceptor cells are arranged randomly: a model for stochastic cell-fate determinationThe Journal of Comparative Neurology 502:75–85.https://doi.org/10.1002/cne.21298
-
A computational approach to edge detectionIEEE Transactions on Pattern Analysis and Machine Intelligence 8:679–698.https://doi.org/10.1109/TPAMI.1986.4767851
-
Active contours without edgesIEEE transactions on image processing : a publication of the IEEE Signal Processing Society 10:266–277.https://doi.org/10.1109/83.902291
-
Genome-wide prediction of minor-groove electrostatic potential enables biophysical modeling of protein-DNA bindingNucleic Acids Research 45:12565–12576.https://doi.org/10.1093/nar/gkx915
-
Thinking about visual behavior; learning about photoreceptor functionCurrent Topics in Developmental Biology 69:187–213.https://doi.org/10.1016/S0070-2153(05)69007-2
-
Patterning of the R7 and R8 photoreceptor cells of Drosophila: evidence for induced and default cell-fate specificationDevelopment 126:607–616.
-
Patterning of the R7 and R8 photoreceptor cells of Drosophila: evidence for induced and default cell-fate specificationDevelopment 126:607–616.
-
The soft touch: low-affinity transcription factor binding sites in development and evolutionCurrent Topics in Developmental Biology 117:455–469.https://doi.org/10.1016/bs.ctdb.2015.11.018
-
Ectopic expression of ultraviolet-rhodopsins in the blue photoreceptor cells of Drosophila: visual physiology and photochemistry of transgenic animalsThe Journal of Neuroscience : The Official Journal of the Society for Neuroscience 12:3862–3868.
-
Organization of the human trichromatic cone mosaicJournal of Neuroscience 25:9669–9679.https://doi.org/10.1523/JNEUROSCI.2414-05.2005
-
Dissection and immunohistochemistry of larval, pupal and adult drosophila retinasJournal of Visualized Experiments e4347.https://doi.org/10.3791/4347
-
Stochastic mechanisms of cell fate specification that yield random or robust outcomesAnnual Review of Cell and Developmental Biology 26:689–719.https://doi.org/10.1146/annurev-cellbio-100109-104113
-
Lessons about terminal differentiation from the specification of color-detecting photoreceptors in the Drosophila retinaAnnals of the New York Academy of Sciences 1293:33–44.https://doi.org/10.1111/nyas.12178
-
Genomic Evidence for Adaptive Inversion Clines in Drosophila melanogasterMolecular Biology and Evolution 33:1317–1336.https://doi.org/10.1093/molbev/msw016
-
Klumpfuss is involved in the determination of sensory organ precursors in DrosophilaDevelopmental Biology 324:177–191.https://doi.org/10.1016/j.ydbio.2008.08.031
-
klumpfuss, a Drosophila gene encoding a member of the EGR family of transcription factors, is involved in bristle and leg developmentDevelopment 124:3123–3134.
-
A thousand fly genomes: an expanded drosophila genome nexusMolecular Biology and Evolution 33:3308–3313.https://doi.org/10.1093/molbev/msw195
-
MEME-ChIP: motif analysis of large DNA datasetsBioinformatics 27:1696–1697.https://doi.org/10.1093/bioinformatics/btr189
-
Stochastic gene expression in mammals: lessons from olfactionTrends in Cell Biology 23:449–456.https://doi.org/10.1016/j.tcb.2013.04.005
-
The reactions to light and to gravity in Drosophila and its mutantsJournal of Experimental Zoology 25:49–106.https://doi.org/10.1002/jez.1400250103
-
A second opsin gene expressed in the ultraviolet-sensitive R7 photoreceptor cells of Drosophila melanogasterThe Journal of Neuroscience : The Official Journal of the Society for Neuroscience 7:1558–1566.
-
Nonlinear filtering of multiplied and convolved signalsProceedings of the IEEE 56:1264–1291.https://doi.org/10.1109/PROC.1968.6570
-
Low-affinity transcription factor binding sites shape morphogen responses and enhancer evolutionPhilosophical Transactions of the Royal Society B: Biological Sciences 368:20130018.https://doi.org/10.1098/rstb.2013.0018
-
Blue- and green-absorbing visual pigments of Drosophila: ectopic expression and physiological characterization of the R8 photoreceptor cell-specific Rh5 and Rh6 rhodopsinsThe Journal of Neuroscience : The Official Journal of the Society for Neuroscience 19:10716–10726.
-
A genetic method for generating Drosophila eyes composed exclusively of mitotic clones of a single genotypeGenetics 152:1631–1639.
-
Classical conditioning and retention in normal and mutant Drosophila melanogasterJournal of Comparative Physiology A 157:263–277.https://doi.org/10.1007/BF01350033
-
Maintaining a stochastic neuronal cell fate decisionGenes & Development 23:385–390.https://doi.org/10.1101/gad.1780509
-
Mechanisms of photoreceptor patterning in vertebrates and invertebratesTrends in Genetics 32:638–659.https://doi.org/10.1016/j.tig.2016.07.004
-
Lozenge directly activates argos and klumpfuss to regulate programmed cell deathGenes & Development 19:1034–1039.https://doi.org/10.1101/gad.1298105
-
A rhodopsin gene expressed in photoreceptor cell R7 of the Drosophila eye: homologies with other signal-transducing moleculesThe Journal of Neuroscience : The Official Journal of the Society for Neuroscience 7:1550–1557.
Article and author information
Author details
Funding
National Eye Institute (R01EY025598)
- Robert J Johnston Jr
Pew Charitable Trusts (00027373)
- Robert J Johnston Jr
The funders had no role in study design, data collection and interpretation, or the decision to submit the work for publication.
Acknowledgements
We are grateful to Steve Britt, Claude Desplan, Lily Jan, Yuh-Nung Jan, Thomas Klein, Chuck Langley, John Pool, Charles Zuker, and the Bloomington and Kyoto Stock Centers for generously providing published fly stocks and antibodies. We thank Erik Anderson, Greg Bashaw, John Kim, Alex Kolodkin, Rejji Kuruvilla, Chris Potter, Geraldine Seydoux, Mark Van Doren and members of the Johnston lab for helpful comments on the manuscript. RJJ was supported by the Pew Scholar Award 00027373 and NIH R01EY025598.
Copyright
© 2017, Anderson et al.
This article is distributed under the terms of the Creative Commons Attribution License, which permits unrestricted use and redistribution provided that the original author and source are credited.
Metrics
-
- 3,549
- views
-
- 440
- downloads
-
- 42
- citations
Views, downloads and citations are aggregated across all versions of this paper published by eLife.
Citations by DOI
-
- 42
- citations for umbrella DOI https://doi.org/10.7554/eLife.29593