An evolutionary recent IFN/IL-6/CEBP axis is linked to monocyte expansion and tuberculosis severity in humans
Abstract
Monocyte counts are increased during human tuberculosis (TB) but it has not been determined whether Mycobacterium tuberculosis (Mtb) directly regulates myeloid commitment. We demonstrated that exposure to Mtb directs primary human CD34+ cells to differentiate into monocytes/macrophages. In vitro myeloid conversion did not require type I or type II IFN signaling. In contrast, Mtb enhanced IL-6 responses by CD34+ cell cultures and IL-6R neutralization inhibited myeloid differentiation and decreased mycobacterial growth in vitro. Integrated systems biology analysis of transcriptomic, proteomic and genomic data of large data sets of healthy controls and TB patients established the existence of a myeloid IL-6/IL6R/CEBP gene module associated with disease severity. Furthermore, genetic and functional analysis revealed the IL6/IL6R/CEBP gene module has undergone recent evolutionary selection, including Neanderthal introgression and human pathogen adaptation, connected to systemic monocyte counts. These results suggest Mtb co-opts an evolutionary recent IFN-IL6-CEBP feed-forward loop, increasing myeloid differentiation linked to severe TB in humans.
https://doi.org/10.7554/eLife.47013.001Introduction
Hematopoiesis, the development of different blood cell lineages from hematopoietic stem cells (HSCs), is a fundamental physiological process in vertebrates. HSCs give rise to lineage-restricted progenitors that gradually differentiate into mature cells. Following cellular differentiation, single-lineage elements including erythrocytes, megakaryocytes, lymphocytes as well as myeloid cells such as monocytes and granulocytes circulate throughout the body performing diverse functions. While HSC development towards cellular lineages during homeostasis has been extensively studied (Hoggatt et al., 2016), the mechanisms by which how progenitors give rise to mature cells during stress responses are less comprehended. For instance, certain pathogens regulate production of blood cells by the bone marrow and it has been shown that fine-tuned regulation of cytokine-induced signals is required for differentiation of HSC into mature cell types (Kleppe et al., 2017; Mirantes et al., 2014; Zhang and Lodish, 2008). For example, the protozoan parasite that causes kalazar, Leishmania donovani, inhabits the bone marrow of humans (Kumar and Nylén, 2012), targets bone marrow stromal macrophages (Cotterell et al., 2000) and induces differentiation of myeloid cells at the expense of lymphoid progenitors (Abidin et al., 2017; Cotterell et al., 2000). In the same line of evidence, after experimental exposure to Gram-negative bacteria, mice display increased amounts of bone marrow-derived neutrophils, through a G-CSF–C/EBPα dependent mechanism (Boettcher et al., 2014). Moreover, infection by intracellular bacteria has been shown to modulate production of circulating leukocytes involving IFN-γ-mediated pathways (Baldridge et al., 2010; MacNamara et al., 2011; Murray et al., 1998). Altogether, these studies indicate vertebrate hosts respond to infection by ‘remodeling’ cell lineage production, which are highly dependent upon the interplay of cytokine-induced hematopoiesis triggered during infection. Interestingly, recent reports have demonstrated hematopoietic stem/progenitor cells (HSPCs) may be infected by different classes of infectious agents such as viruses and bacteria, albeit at low efficiency (Carter et al., 2011; Kolb-Mäurer et al., 2002). Therefore, since many pathogens may reach the bone marrow and provide microbial-HSC interactions, it is possible that, in addition to cytokines, pathogen recognition by progenitor cells directly regulate cell lineage commitment providing an anti-microbial defense system. In contrast, the Red Queen hypothesis (Van Valen, 1973) predicts such pathogens would benefit from cell lineage commitment to establish themselves into the host.
The human pathogen Mycobacterium tuberculosis (Mtb) has been recently detected in circulating HSCs (Lin-CD34+) from latent TB individuals (Tornack et al., 2017). Since Mtb can also gain access to the bone marrow during extra-pulmonary (Mert et al., 2001) as well as active pulmonary TB (Das et al., 2013), it has been suggested that the human bone marrow is a niche/reservoir for this bacterium during natural pathogen infection. However, whether interactions between Mtb and human CD34+ cells drive cellular differentiation has not been formally demonstrated. Interestingly, earlier (Rogers, 1928; Schmitt et al., 1977) and recent (Berry et al., 2010; Zak et al., 2016) studies have reported major changes in the peripheral myeloid cells such as increased blood counts and dysregulated ‘interferon transcriptional signature’ during active TB. More specifically, several ‘interferon-stimulated genes’ (ISGs) are modulated in circulating mature neutrophils and monocytes in active TB patients, which calls forth a possible role of such genes in TB pathogenesis (Berry et al., 2010; Dos Santos et al., 2018; Zak et al., 2016). In contrast, lymphocyte compartments were recently demonstrated to be contracted during progression from latent to active TB in humans (Scriba et al., 2017). Therefore, the observed changes in blood leukocytes could be a consequence of the interactions between Mtb and the bone marrow cellular environment. Thus, we hypothesized that Mtb regulates cellular differentiation of human HSPCs. By employing in vitro functional assays and integrated systems biology analysis of published available cohorts of healthy controls and TB patients, our study suggests that Mtb co-opts an evolutionarily recent IFN/IL-6/CEBP axis linking monocyte differentiation and disease severity.
Results
Mtb H37Rv replicates in primary human CD34+ cell cultures
To investigate the dynamics of Mtb infection by HSPCs, we have exposed peripheral blood mononuclear cells (PBMCs) from healthy donors to H37Rv Mtb (multiplicity of infection, MOI3) and measured bacterial infectivity by CD34+ cells. First, by using a fluorescent dye (syto-24) which does not influence bacteria infectivity (data not shown), flow cytometry experiments demonstrated that Mtb were associated with CD34+ cells following 4 hr exposure to mycobacteria (Figure 1a,b and Figure 1—figure supplement 1a). At that time point, we observed ~69% of CD34+ and ~79% of CD14+ associated with Mtb (Figure 1c). When compared to CD14+ cells, which are highly phagocytic cells, the MFI measurements within the CD34+ cell population were found to be ~4 x lower (Figure 1d). These data suggest that PBMC CD34+ cells may be permissive to Mtb infection in vitro. Similarly, purified cord blood derived CD34+ cells display comparable % and MFI as those seen in PBMC CD34+ cells (Figure 1—figure supplement 1b). Confocal microscopy analysis confirmed the presence of sparse intracellular mycobacteria in purified cord blood derived CD34+ cells at 4 hr post-infection (pi) (Figure 1—figure supplement 1c). These findings raise the possibility that although human primary Lin-CD34+ cells can be infected by Mtb in vitro and in vivo (Tornack et al., 2017), this cell population may display intrinsic resistance to Mtb infection as it has been reported for other bacterial species (Kolb-Mäurer et al., 2002). Next, we employed a purified cell culture system to investigate whether H37RV Mtb replicates in CD34+ cells in different time points. When sorted purified cord-blood CD34+ cells were exposed to Mtb H37Rv (MOI3) and cultivated in StemSpan SFEM II (Bodine et al., 1991; Keller et al., 1995), bacilli numbers exhibited a ~ 1.5 log growth at 5 days post infection (dpi) (Figure 1e). As a control, purified CD14+ cells displayed higher bacterial proliferation than purified CD34+ cells over time (Figure 1-Figure 1—figure supplement 1d). Together, these findings demonstrate that Mtb infects and replicates in primary human CD34+ cell cultures in vitro. While at one dpi bacilli were more associated with the surface of round cells (Figure 1f), at five dpi intracellular bacteria were associated with cells with abundant cytoplasm (Figure 1f), suggesting that Mtb-exposed cultures displayed increased frequencies of cells exhibiting morphological alterations over time (Figure 1f, right panel). Indeed, Giemsa staining (Figure 1g, arrows) presented higher frequency of cytoplasm- richer cells in bacteria-exposed vs uninfected cell cultures (Figure 1g, right panel), thus suggesting that Mtb infection enhances cellular differentiation by CD34+ cells in vitro.
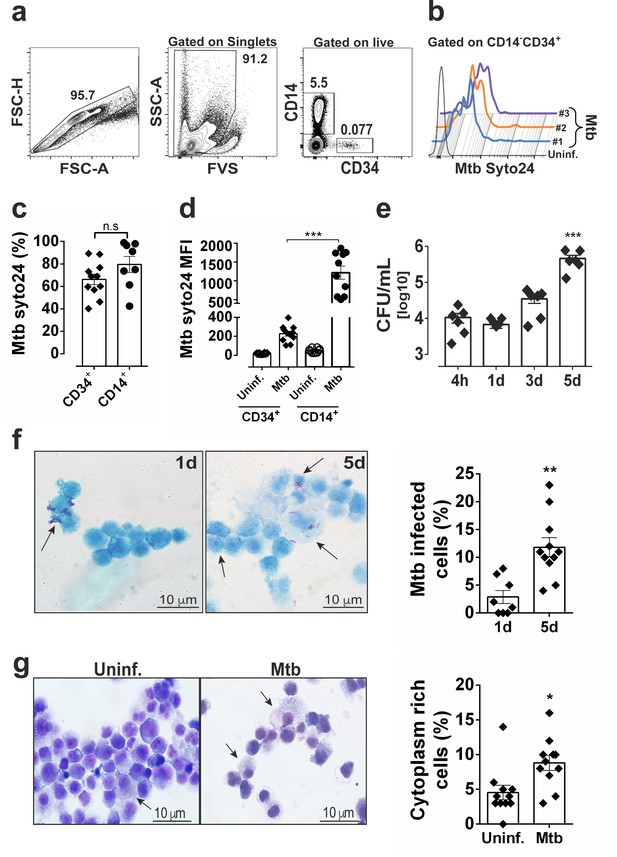
Mtb H37Rv infects human CD34+ cells and proliferates in cell cultures in vitro.
PBMC from healthy donors were exposed to syto24-labeled Mtb H37Rv (MOI3, Figure 1—figure supplement 1a) for 4 hr. (a) Representative flow cytometry contour plots of gating strategy to analyze Mtb syto24 association in FVS-negative (live) CD34+ events and CD14+ events. (b) Live CD34+Lin- events gated in a were analyzed for Mtb-Syto24 MFI. Black line: Uninfected control. Blue, orange and purple lines represent samples from three different donors. (c) Frequencies and (d) MFI of Mtb syto24+ events in CD34+ or CD14+ events gates from uninfected or Mtb syto24-exposed bulk PBMCs. Results are means ± SEM of data pooled from three independent experiments, n = 10 healthy donors. ***p≤0.001 between Mtb syto24 CD34+ vs CD14+ groups. (e,) Purified cord blood-derived CD34+ cells were exposed to Mtb H37Rv (MOI3) for different time points and CFUs from cell culture lysates were enumerated in 7H10 media. Results are means ± SEM of data pooled from five independent experiments, ***p≤0.001 between 5d vs 4 hr groups. (f) Kinyoun staining of CD34+ cells after 1d and 5d of infection and quantification, as described in the methodology section, shown in the right panel. Arrows indicate cells associated with bacilli. Experiments shown are representative of two performed. **p≤0.01 between 5d vs 1d groups. (g) Representative Giemsa staining of CD34+ cells of 5d-cultures and quantification, as described in the methodology section, shown in the right panel. Arrow indicates cytoplasm-rich cells in Mtb-infected cultures and uninfected cultures. Experiments shown are representative of two performed. *p≤0.05 between Mtb vs uninfected groups.
-
Figure 1—source data 1
Raw data from Figure 1.
- https://doi.org/10.7554/eLife.47013.005
Live Mtb induces CD34+ cells towards myeloid differentiation and monocyte output
Next, to investigate whether Mtb triggered cellular differentiation by human CD34+ cells, we evaluated differential expression of 180 transcription factors (TFs) associated with differentiation of distinct hematopoietic cells (Novershtern et al., 2011) in RNA-seq samples of Mtb-exposed purified cells (Figure 1—figure supplement 1e – Figure 2—source data 1). Interestingly, Mtb infection increased the expression of lineage-specific regulators of myeloid (GRAN/MONO) (SPI1, CEBPB, CEBPA, EGR2 and STAT2), but not lymphoid (B and T CELL) (GABPA, SOX5, TCF3, GATA3, LEF1, RORA and LMO7) or megakaryoid/erythroid (EARLY/LATE ERY) (GATA1, FOXO3, NFE2, TAL1) differentiation (Figure 2a – Figure 2—source data 1). Similarly, CellRouter (Lummertz da Rocha et al., 2018) signature score of the GRAN/MONO gene set was found to be increased in Mtb vs uninfected samples in all time points studied (Figure 2b - Figure 2—source data 1). Next, we applied a network biology-built computational platform (Cahan et al., 2014) which, based on classification scores, can assess the extent to which a given population resembles mature cell types. Figure 2c (Figure 2—source data 1) shows that mRNA samples from Mtb-exposed CD34+ cells presented enrichment of monocyte/macrophage profiles, but not other mature cell populations such as lymphocytes or dendritic cells. Additionally, at five dpi, CD34+ cell cultures displayed increased frequencies of cells positive for CD11b (Figure 2d), a surface molecule expressed during myeloid differentiation (Hickstein et al., 1989; Rosmarin et al., 1989). Together, these data suggest that Mtb drives human primary CD34+ cells towards myeloid differentiation. We next employed flow cytometry to measure cell surface molecules previously associated with myeloid differentiation of human CD34+ cells (Cimato et al., 2016; Gorczyca et al., 2011; Kawamura et al., 2017; Manz et al., 2002; Olweus et al., 1995). More specifically, we gated on CD34+ events and quantified % CD64+CD4+ cells. Corroborating our hypothesis, Mtb enhanced the frequency of CD64+CD4+CD34+ cells (Figure 2e–h) at five dpi, but not CD10+CD34+ (lymphoid) or CD41a+CD34+ (megakaryoid) progenitors (Figure 2—figure supplement 1a). Similarly, Lin-CD34+ cells from PBMC (Figure 2—figure supplement 1b) samples from healthy donors exposed to Mtb displayed increased frequency of CD4+CD64+CD34+ cells and augmented levels of CD38 and HLA-DR (Figure 2—figure supplement 1b,c), two molecules associated with advanced stage of cellular differentiation (Cimato et al., 2016; De Bruyn et al., 1995; Terstappen et al., 1991). When compared to their base line levels, we also observed an increased % CD4+CD64+CD34+ cells in Mtb-exposed bulk bone marrow samples from two healthy individuals (Figure 2—figure supplement 1c, p=0.08). In addition, frequencies of CD4+CD64+CD34+ cells were higher in cultures infected to live H37Rv Mtb than those exposed to heat-killed (HK) bacteria (Figure 2f,g). These results suggest that the observed cellular phenotype was mostly due to the activities of live pathogen and only partially to mycobacterial PAMPs such as TLR2 (Ara-LAM) or TLR9 (Mtb gDNA) agonists (Bafica et al., 2005; Underhill et al., 1999), which induced CD38 and HLA-DR, but not CD4 and CD64 expression in CD34+ cells (Figure 2—figure supplement 1d). Importantly, increased frequency of CD4+CD64+CD34+ cells was also observed when cell cultures were exposed to a clinical isolate of Mtb (Figure 2h), ruling out a possible genetic factor associated with the laboratory strain H37Rv (Brites and Gagneux, 2015). Furthermore, CD34+ cell death was not enhanced by Mtb infection as demonstrated by the use of a live-and-dead probe and lactate dehydrogenase (LDH) quantification in cell culture supernatants (Figure 2—figure supplement 1e,f). Together, these data indicate live Mtb directs primary human CD34+ cells towards myeloid differentiation in vitro.
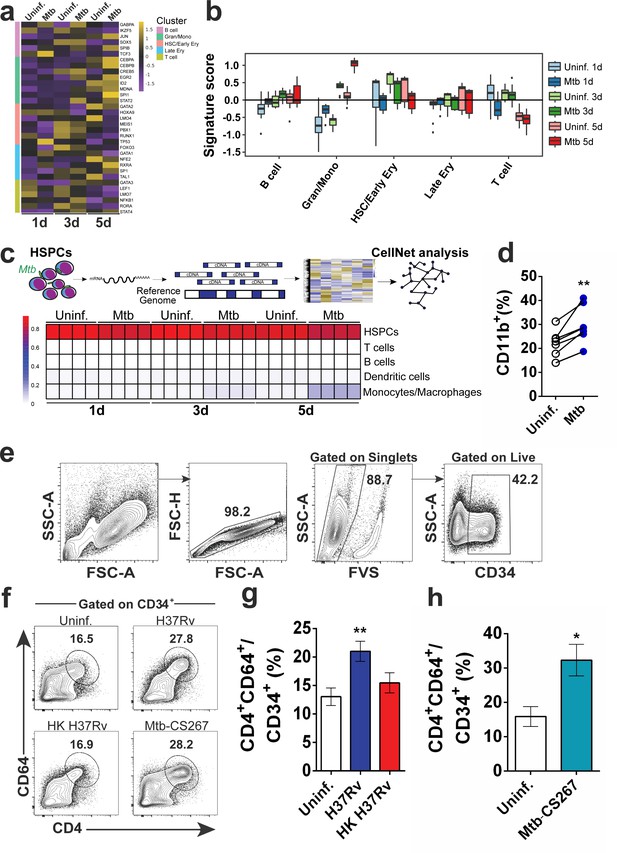
Live Mtb induces human CD34+ cells towards myeloid differentiation in vitro.
Purified CD34+ cells from healthy donors (n = 3) were exposed to Mtb H37Rv (MOI3) for different time points and mRNA-seq was performed as described in methodology section. (a) Heatmap of the mRNA expression (z-score) of transcription factors involved in cell lineage commitment (Novershtern et al., 2011). (b) Signature score of data from a) by employing CellRouter analysis. (c) Heatmap from mRNA data of uninfected vs Mtb infected cultures analyzed by CellNet. (d) Purified CD34+ cells were exposed to Mtb H37Rv (MOI3) for 5 days and flow cytometry was performed. Graph represents frequencies of CD11b+ events in uninfected (open circles) vs Mtb-infected cultures (blue circles) from four independent experiments. **p≤0.01 between Mtb and uninfected groups. (e) Purified CD34+ cells were exposed to Mtb H37Rv, Heat-killed (HK) Mtb H37Rv or Mtb clinical isolate 267 (Mtb-CS267) (MOI3) for 5 days and flow cytometry with the gating strategy was performed. (f) Representative contour plots show frequencies of CD4+CD64+ events in CD34+ events. CD34+CD4+CD64+ events of polled data from f) were plotted to generate bar graphs (g) and (h). Results are means ± SEM of data pooled from four independent experiments (g) and two independent experiments (h). (g) ** indicates p≤0.01 between H37Rv vs uninfected or HK H37RV groups. (h) * indicates p≤0.05 between Mtb-CS267 vs uninfected groups.
-
Figure 2—source data 1
Raw data from Figure 2.
- https://doi.org/10.7554/eLife.47013.009
-
Figure 2—source data 2
Counts matrix of RNAseq data of Mtb-exposed and control CD34+ cell transcriptomes.
- https://doi.org/10.7554/eLife.47013.010
Next, to investigate whether Mtb enhanced HSPC differentiation into mature myeloid populations (Kawamura et al., 2017; Lee et al., 2015; Manz et al., 2002), purified CD34+ cells were exposed to Mtb and 10d later, surface molecules were measured by flow cytometry. Live H37Rv (Figure 3a,e) or clinical isolate Mtb, (Figure 3—figure supplement 1a) but not Leishmania infantum promastigotes (data not shown), enhanced expression of the monocyte surface molecule CD14, confirming the observed monocyte/macrophage output enrichment by CellNet analysis (Figure 2c). Interestingly, in this in vitro culture system, CD14+ cells started to emerge at low levels at day three in both uninfected and Mtb-exposed cell cultures, while enhancement in monocyte frequency was observed in Mtb-stimulated cells at later time points, that is at 7 and 10 dpi. (Figure 3—figure supplement 1b). Compared to control cell cultures, CD14+ cells induced by Mtb displayed similar MFI expression of CD11b, HLA-DR, CD64 and CD16 surface molecules (Figure 3i) and most, but not all experiments presented increased frequency of CD14+CD16+ monocytes (Figure 3j), which were previously associated with severe pulmonary TB (Balboa et al., 2011). Moreover, albeit not statistically significant, Mtb enhanced the frequency of CD16+CD66b+ neutrophils in the majority but not all samples tested (Figure 3b,f). In contrast, HK Mtb did not stimulate monocyte or neutrophil output (Figure 3e,f). As expected (Figure 2—figure supplement 1a), megakaryoid/platelet- (Figure 3c,g), dendritic cell- (Figure 3d,h) or erythroid- (Figure 3—figure supplement 1c) associated markers were unchanged after exposure to live or HK Mtb. Altogether, these results suggest Mtb selectively favors the generation of monocytes and, to a lesser extent, neutrophils, by human CD34+ cells in vitro.
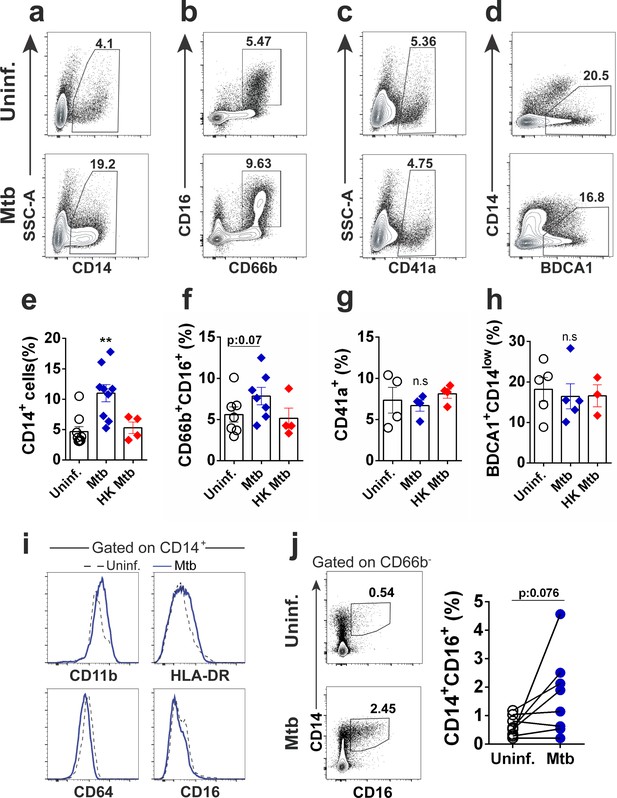
Mtb infection increases monocyte output from CD34+ cells in vitro.
Purified CD34+ cells were exposed to live Mtb H37Rv or HK Mtb H37Rv (MOI3) for 10 days and flow cytometry was employed to determine the mature cell frequencies in the cell cultures. Representative dot plots of (a) monocytes (CD14+), (b) neutrophils (CD16+CD66b+), (c) megakaryocytes/platelets (CD41a+) and (d) classical myeloid dendritic cells (BDCA1+CD14low) in uninfected and Mtb-infected CD34+ cell cultures. Graphs show frequencies of (e) CD14+, (f) CD16+CD66b+, (g) CD41a+ and (h) BDCA1+CD14low events in uninfected (open circles), live Mtb-infected (blue diamonds) or HK Mtb-exposed (red diamonds) cell cultures at day 10. Each symbol represents one individual experiment. Results are means ± SEM of data pooled from 3 to 9 independent experiments. **p≤0.01 between Mtb vs uninfected or HK Mtb groups. (i,) Histograms show the expression of CD11b, HLA-DR, CD64 and CD16 in CD14+ events from a). Black dashed lines: Uninfected control. Blue solid lines: Mtb-infected group. Data representative of 5 independent experiments. (j,) Frequency of CD14+CD16+ events in Mtb-exposed cell cultures after 10d. Contour dot plot of CD14+CD16+ frequencies from one representative donor. Open circles: Uninfected control. Blue circles: Mtb-infected group. Each symbol represents an individual experiment. Pooled data of eight independent experiments, n = 5 different donors. p=0.076 between Mtb vs uninfected groups.
-
Figure 3—source data 1
Raw data from Figure 3.
- https://doi.org/10.7554/eLife.47013.014
In vitro Mtb-enhanced myeloid differentiation is mediated by IL-6R, but not type I or type II IFN signaling
Cytokines are important triggers of Lin-CD34+ differentiation in vivo and in vitro (Endele et al., 2014; Hoggatt et al., 2016; Zhang and Lodish, 2008) and Reactome pathway analysis of genes differentially expressed between Mtb-infected versus uninfected conditions displayed enrichment of ‘cytokine signaling in immune system’ (Figure 3—figure supplement 1d; n = 3 donors, two independent experiments - Figure 2—source data 1). Among several genes, we observed a significant enrichment of IL6 (Supplementary file 1 - Figure 2—source data 1), a key HSPC-derived regulator of myeloid differentiation in mouse and human models (Jansen et al., 1992; Zhao et al., 2014) which was confirmed in our system by the addition of exogenous IL-6 to CD34+ cells (Figure 4—figure supplement 1a). Moreover, cytokine receptors, including IL6R, as well as their cytokine partners, containing IL6, were enriched in Mtb-exposed CD34+ cell cultures (Figure 4a,b and Figure 4—figure supplement 1b - Figure 2—source data 1). Similarly, increased IL6 expression was confirmed by qPCR (Figure 4—figure supplement 1c). In addition, ‘interferon signaling’ and ‘interferon alpha/beta’ pathways were significantly enriched in Mtb-exposed CD34+ cells (Figure 3—figure supplement 1d and Supplementary file 1 - Figure 2—source data 1). This was confirmed in five donors, which displayed increased levels of IFNA2, IFNB and IFNG transcripts, albeit at a lower level relative to IL6 mRNA (Figure 4—figure supplement 1c). Importantly, interferon-stimulated genes (ISGs) such as MX1, ISG15 and IFI16 as well as IL-6R-stimulated genes such as IL1RA, GRB2 and CXCL8 were enhanced in Mtb-stimulated CD34+ cells from five different donors (Figure 4—figure supplement 1d), suggesting IL-6 and IFN signaling are active in these cells. Corroborating previous findings showing that HSPCs produce IL-6 following microbial stimuli (Allakhverdi and Delespesse, 2012), live Mtb also induced intracellular IL-6 production in Lin-CD34+ cells from bacteria-exposed PBMC 1d cultures (Figure 4c). This was confirmed in 1d culture supernatants of purified CD34+ cells exposed to live Mtb which presented augmented levels of IL-6, but not IFN-γ, IL-1β or TNF (Figure 4—figure supplement 1e). Interestingly, while HK Mtb also stimulated production of IL-6 (Figure 4c), dead bacteria did not induce CD38, CD4 and CD64 expression in PBMC Lin-CD34+ cells as seen in cell cultures exposed to live Mtb (Figure 4—figure supplement 1f). When compared to the live pathogen, qPCR experiments with HK Mtb-exposed purified CD34+ cells did not show induction of ISG STAT1 (Figure 4—figure supplement 1g), suggesting the existence of cross talking regulatory pathways between live Mtb, IL-6 and IFN signaling to boost myeloid differentiation in vitro. Since these data pointed that IL-6 and IFN signaling are potential pathways involved in Mtb-enhanced myeloid differentiation by CD34+ cells, we employed neutralizing monoclonal antibodies as a tool to investigate this possibility. While type I IFN signaling was necessary for Mtb-stimulated ISGs such as STAT1 and MX1 transcription (Figure 4—figure supplement 1h), neither type I nor type II IFN signaling pathways were required for Mtb-enhanced monocyte/granulocyte conversion (Figure 4d,e and Figure 4—figure supplement 1i). In contrast, neutralizing anti-IL-6Ra antibody (α-IL-6R) inhibited background levels of CD14+ monocytes and CD66b+ granulocytes, as well as Mtb-enhanced myeloid differentiation by CD34+ cell cultures (Figure 4f–h) but not transcription of STAT1 and MX1 (Figure 4—figure supplement 1h). In addition, megakaryoid, erythroid- or dendritic cell-associated surface molecules were unaltered in α-IL-6R-treated cell cultures (Figure 4—figure supplement 1j–l). Interestingly, Mtb-exposed CD34+ cell cultures treated with α-IL-6R (Figure 4i) presented significantly lower CFU counts when compared with infected untreated control cell cultures, while α-IFNAR2 (Figure 4j) or α-IFN-γ (Figure 4k) did not affect CFU counts. Together, these results suggest live Mtb enhances IL-6R-mediated myeloid differentiation by human CD34+ cells in vitro.
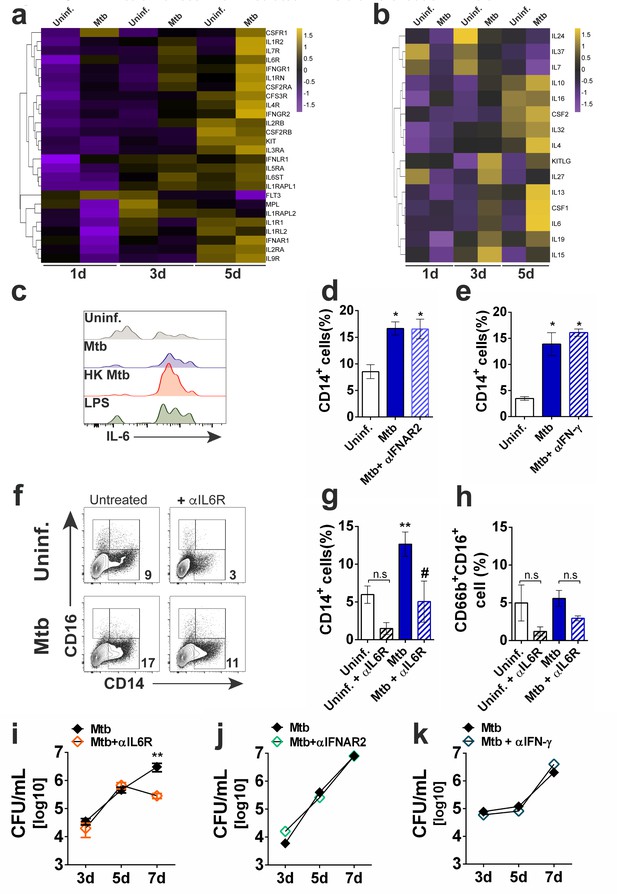
Mtb enhances IL-6R-mediated myeloid differentiation in vitro.
Purified CD34+ cells were exposed to Mtb H37Rv (MOI3) for different time points and mRNA-seq was performed as described in the methodology section. (a) Heatmap (z-score) of differentially expressed cytokine receptor genes. (b) Heatmap (z-score) of differentially expressed cytokine genes. Shown is the average mRNA expression of three different donors from two independent experiments. (c) PBMC from healthy donors were exposed to Mtb H37Rv, HK Mtb or LPS (100 ng/mL) for 24 hr and intracellular IL-6 was detected by flow cytometry. Live CD34+Lin- events gated as in Figure 1a were analyzed for IL-6 MFI. Representative histogram from two independent experiments. Purified CD34+ cells were treated with (d) α-IFNAR2 (1 µg/ml) or (e) α-IFN-γ (10 µg/ml) and then exposed to Mtb H37Rv (MOI3) during 10d for determination of CD14+ monocyte frequencies. Results are means ± SEM of data pooled from two independent experiments. *p≤0.05 between Mtb, α-IFNAR2 or α-IFN-γ vs uninfected groups. (f) Representative contour plots of CD14+ monocytes in CD34+ cell cultures exposed to Mtb, in the presence or absence of α-IL6R (Tocilizumab, 1 µg/ml) for 10d. (g) Results shown are means ± SEM of data pooled from three independent experiments from (f) **p≤0.01 between Mtb vs uninfected groups and #p≤0.05 between Mtb and Mtb+α-IL6R-treated groups. (h) Results shown are means ± SEM of data pooled from three independent experiments showing frequency of CD66+CD16+ neutrophils in Mtb-infected cell cultures in the presence or absence of α-IL6R. Purified CD34+ cell cultures were treated as in (d–f) with (i) α-IL6R, (j) α -IFNAR2 and (k) α-IFN-γ and then exposed to Mtb (MOI3) for different time points and CFU enumerated as described in the methodology section. Results are means ± SEM of data pooled from four independent experiments. **p≤0.01 between Mtb and Mtb+ α-IL6R at 7d.
-
Figure 4—source data 1
Raw data from Figure 4.
- https://doi.org/10.7554/eLife.47013.018
An IL6/IL6R/CEBPB gene module is enriched in the active TB transcriptome and proteome
To investigate whether IL-6R signaling correlates with monocyte expansion and TB-associated pathology in vivo, we performed a comprehensive systems biology analysis integrating several large transcriptomic and proteomic data sets from published cohorts of healthy controls and patients with latent, active and disseminated TB (Berry et al., 2010; Hecker et al., 2013; Naranbhai et al., 2015; Novikov et al., 2011; Scriba et al., 2017) (Supplementary file 2). First, we used Ingenuity Pathway analysis (IPA) to determine IL-6/IL-6R upstream regulators in transcriptomes from publicly available CD14+ monocytes of active TB patients (Berry et al., 2010).. As shown in Figure 5a (top panel), IL6, IL6ST, IL6R and STAT3 were significantly enriched in transcriptomes of active TB monocytes, when compared to cells from healthy controls. As reported previously (Berry et al., 2010; Mayer-Barber et al., 2011; Novikov et al., 2011), STAT1 and IL1B were also confirmed as upstream regulators in active TB monocytes (Figure 5a, top panel). We next examined potential genes share between IL6/IL6R and type I IFN signaling pathways in active TB monocytes. Strikingly, the two top upstream regulators in TB monocytes, IRF1 and STAT1 (Figure 5a, top panel), were the only genes in common between the TB monocyte gene signature (Berry et al., 2010), the ‘IL6/STAT3 pathway’ and the ‘in vivo IFN-β” signature (Figure 5a, Venn diagram, bottom panel), suggesting these genes might be regulated by both IL-6 and type I IFN during active TB in vivo. Since type I IFN and IL-6 share the ability to induce phosphorylation of both STAT1 and STAT3 (Ho and Ivashkiv, 2006), we ran gene set enrichment analysis (GSEA) (Subramanian et al., 2005) to identify potential overlapping downstream target genes in the whole blood ‘Berry TB’ disease signature (Berry et al., 2010). In addition to the previously demonstrated type I IFN/STAT1 signature (Berry et al., 2010), the ‘IL6/STAT3’ pathway was significantly enriched in this data set (FDR-corrected p<10−4, Supplementary file 2). Next, we defined protein signatures by overlapping the ‘Berry TB’, the ‘IL6/STAT3’ pathway with a published plasma proteome defining disease progression from latent to active TB (Scriba et al., 2017) (‘Scriba Plasma TB’, Figure 5b, Venn Diagram top panel). STRING network analysis of protein-protein interactions confirmed two clusters (Figure 5b, bottom panel), which comprised three signatures: ‘CD34/myeloid’, ‘IL6/STAT3’ and ‘IFN/IL6-shared’ pathways. Reanalysis of the published ‘Scriba Plasma TB’ proteome set (Scriba et al., 2017) confirmed increased IL-6/STAT3 protein levels and changes in CD34/CD38 homeostasis, which were found to be early events in TB pathogenesis (Figure 5c, top panel). ‘IL6/STAT3’ pathway-associated proteins such as PLA2G2A, CRP, STAT3, IL-6 and CFB increased around 12 months before TB diagnosis (Figure 5c,d, top panels - orange circles and bars), which was concomitant with significant changes in the ‘IFN/IL6-shared’ plasma markers CXCL10, IFNAR1 and MMP9 (Figure 5c,d top panels - blue circles and bars). The ‘IL6/STAT3’ and ‘IFN/IL6-shared”pathways were also significantly enriched in a gene set recently linked to monocyte expansion in vivo, measured as monocyte:lymphocyte (ML) ratio (Naranbhai et al., 2015) (Supplementary file 2), and positively correlated with mycobacterial growth in vitro, thus connecting monocyte expansion and increased Mtb survival. Interestingly, the changes found in both the ‘IL6/STAT3’ and ‘IFN/IL6-shared’ pathways during development of TB disease preceded enrichment of the ‘ML ratio’ gene set (Naranbhai et al., 2015) (6 months before diagnosis, p<0.05, Figure 5c, bottom panel and Figure 5d, top panel, gray bar) and reduction of the CD34/CD38 gene markers, in agreement with our in vitro model of Mtb-enhanced CD34+ differentiation (CD34+ → CD34+CD38+ → CD14+). Moreover, fold changes were higher for ‘IL6/STAT3’ pathway genes than for ‘IFN/IL6-shared’ genes, and significantly higher than ‘ML ratio’ genes (p<0.05) or ‘CD34/myeloid’ differentiation genes (p<0.01) (Figure 5d bottom panel). Together, these data suggest sequential activation of IL-6/IL-6R and IFN signaling pathways before monocyte expansion during TB disease progression in vivo, raising a possible link between these two events in disease pathogenesis. In support of this idea, CD34+ cells exposed to Mtb in vitro displayed increased levels of pSTAT1 as well as C/EBPβ (and a slight enhancement of C/EBPα), which are key TF regulators of ISGs and myeloid differentiation genes, respectively (Figure 5—figure supplement 1a). Interestingly, qPCR experiments from HK Mtb-exposed CD34+ cells did not show induction of myeloid differentiation TFs CEBPA and CEBPB (Figure 4—figure supplement 1g). Furthermore, we observed that CEBPB, CEBPD and STAT3 as well as IRF1, STAT1 and ICSBP/IRF8 TFs were significantly enriched in Mtb-infected CD34+ transcriptomes (Figure 5e and Figure 5—figure supplement 1b - Figure 2—source data 1), which were associated with increased mycobacterial replication in vitro (Figure 1e). These results suggest that Mtb infection activates a gene module shared by both type I IFN and IL-6, linking downstream ISGs and CEBPs.
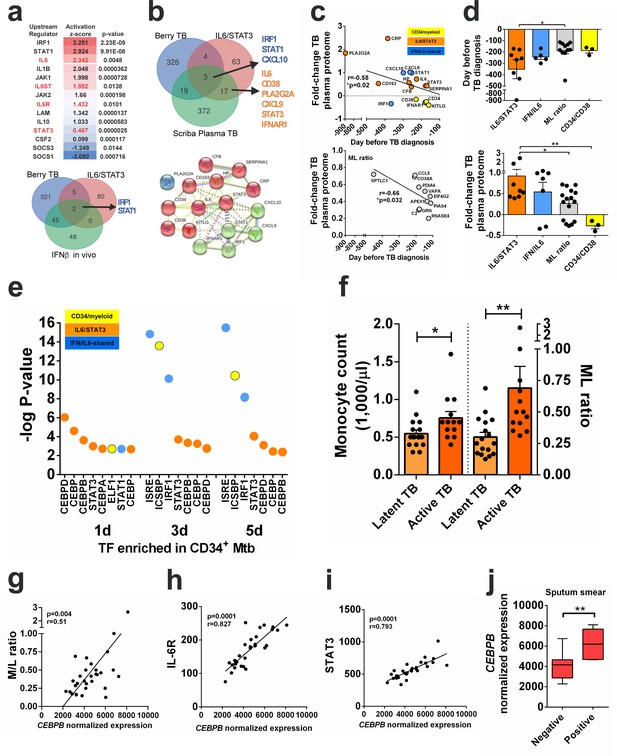
IL6/IL6R/CEBPB gene module is enriched in active TB transcriptome and proteome and correlates with monocyte expansion.
(a) Top panel: upstream regulators significantly enriched by causal Ingenuity Pathway Analysis (IPA) in monocyte transcriptomes from patients with active TB (GSE19443), ranked by activation z-score, p-values are corrected for genome-wide testing (FDR). Bottom panel: IRF1 and STAT1 are the top upstream regulators shared between the ‘Berry TB’ disease signature (Berry et al., 2010) (GSE19435, GSE19439, GSE19444), the ‘IL6/STAT3’ pathway (Hallmark GSEA) and the human ‘in vivo IFN-β” signature (GSEA HECKER_IFNB1_TARGETS). (b) Top panel: overlap between the ‘Berry TB’ disease signature, the ‘IL6/STAT3’ pathway and the ‘Scriba plasma TB’ proteomic signature (Scriba et al., 2017) identified ‘IFN/IL6-shared’ and ‘IL6/STAT3-specific’ signatures. Bottom panel: significant STRING protein-protein interaction network (p<10−16) for ‘IFN/IL6-shared’ genes (green marbles) and ‘IL6/STAT3’ genes (red marbles), clustering separately by k-means. (c) Top panel: significant linear increase over time before active TB diagnosis in plasma proteome (Scriba et al., 2017) for ‘CD34/myeloid’ (yellow), ‘IL6/STAT3’ (orange) and ‘IFN/IL6-shared’ (blue) clusters found in (b). Bottom panel: monocyte/lymphocyte (ML) ratio gene set members defined by Naranbhai et al. (2015) over time before active TB diagnosis in plasma proteome (Scriba et al., 2017).(d) Top panel: increased ‘IL6/STAT3’ cluster protein expression precedes monocyte expansion markers (ML ratio gene set) in the TB plasma proteome. Bottom panel: data as in d) shows significant higher fold-changes for ‘IL6/STAT3’ vs. ‘ML ratio’ or ‘CD34/myeloid’ cluster members. *p-value<0.05, ** p-value<0.01. (e) Transcription factor enrichment analysis (GSEA) of differentially expressed genes determined by RNA-seq in Mtb-exposed CD34+ cells in vitro (n = 3 donors). (f), monocyte count and ML ratio in samples from latent vs active TB patients from Berry et al. (2010) reanalysis. *p-value<0.05, ** p-value<0.01 between active TB vs latent TB groups. Transcriptional data of whole blood reanalysis from Berry et al. (2010) shows a significant correlation of CEBPB transcripts with g) M/L ratio; h) IL6R; i) STAT3 transcript levels, and j) mycobacterial positivity in sputum smears in patients with active TB. ** p-value<0.01 between positive vs negative groups.
-
Figure 5—source data 1
Raw data from Figure 5.
- https://doi.org/10.7554/eLife.47013.021
An IL6/IL6R/CEBP gene module correlates with monocyte expansion and TB severity
TB pathogenesis is a convoluted process which interconnects mycobacterial dissemination, host inflammatory responses and systemic tissue pathology. To further investigate a potential link between this gene module (IL6/IL6R/CEBP) with disease severity and monocyte expansion in vivo, we first examined large transcriptomic data sets of ‘disseminated TB’, which includes extrapulmonary and lymph node TB (GSE63548). The ‘IL6/STAT3’ pathway was found to be significantly enriched among differentially expressed genes in both extrapulmonary (FDR p=10−3) and lymph node TB (FDR p=10−4, Supplementary file 2). Furthermore, downstream targets of STAT3, CEBPB, CEBPD, SPI1/PU1, ICSBP/IRF8, which are TF regulators of myeloid differentiation, were enriched in ‘disseminated TB’ and in the ‘ML ratio’ gene sets (Supplementary file 2), suggesting these TFs are activated during severe disease and associated to monocyte expansion in vivo. In contrast, ISRE (STAT1/STAT2) and IRF1 motifs, the major upstream regulators observed in TB monocyte transcriptome (Figure 5a top panel) and shared between IL-6 and IFN signaling (Figure 5b), were not enriched in the ‘ML ratio’ gene set (Supplementary file 2). Of note, only CEBPB targets were significantly enriched in the ‘ML ratio’ gene set in healthy subjects (Supplementary file 2), supporting its link with myeloid differentiation during homeostasis. Since the IL6/IL6R/CEBP gene module was correlated with both systemic disease dissemination and monocyte expansion, two processes associated with TB disease (Rogers, 1928; Schmitt et al., 1977), we next examined whether these genes might be connected to disease severity in a published cohort with detailed clinical parameters and transcriptome data (Berry et al., 2010). When compared to latent TB subjects, we observed that both the monocyte counts and ML ratio were significantly increased in active TB patients (Figure 5f). CEBPB transcripts positively correlated with ML ratio levels (Figure 5g), IL6R transcripts (Figure 5h), STAT3 (Figure 5i) as well as inflammatory biomarkers such as C-reactive protein (CRP) and erythrocyte sedimentation rate (ESR) (Figure 5—figure supplement 1c). In addition, CEBPB mRNA levels were significantly higher in Mtb-positive vs. Mtb-negative sputum smears (Figure 5j), and positively correlated to tissue damage, total symptom counts as well as ISG15 levels (Figure 5—figure supplement 1c), in agreement with our previous findings (Dos Santos et al., 2018). Taken together, these results indicate that the IL6/IL6R/CEBP gene module is a hub correlated with monocyte expansion during Mtb infection in vivo and is amplified in severe pulmonary and systemic disease.
Recent mammalian/primate genetic changes link an IFN/IL-6/IL-6R/CEBP axis to monocyte expansion and TB pathogenesis in humans
The ‘type I IFN’ signature found in active TB (Berry et al., 2010), shared with the IL-6/IL-6R-regulated gene set (Figure 5), comprises a number of well-characterized ISGs with cross-species antiviral activity such as IRF1 and OAS. It has been reported that these ISGs have been undergoing strong purifying selection during primate evolution (Manry et al., 2011; Shaw et al., 2017), including recent Neanderthal introgression (Enard and Petrov, 2018; Quach et al., 2016). We thus undertook an evolutionary approach to investigate whether the IL6/IL6R/CEBP gene module and its partial overlap with type I IFN signaling is linked to monocyte expansion and TB severity. To do so, we performed a stepwise analysis, starting from early mammalian emergence (>100 million years ago (mya), over primate (>50 mya) and hominid evolution (>15 mya). We have also examined the recent human evolution including Neanderthal introgression (<100,000 years ago) and human pathogen adaptation (15,000–1,500 years ago), up to extant human genetic variation through analysis of large genome wide association studies (GWAS).
First, STRING network measurements of amino acid conservation and gene co-occurrence across mammalian and primate evolution revealed that IL-6, IL-6R and C/EBP family members C/EBPα, C/EBPβ and C/EBPδ differ substantially throughout primate evolution and even among closely related hominins (Pan troglodytes and Gorilla gorilla) (Figure 6a, heat map). In contrast, matched control molecules in the same STRING network (KLF5/NFKB1/MAPK1//STAT1/STAT3) remained largely conserved in most mammals, and even in birds and reptiles (Figure 6a, heat map and Figure 5—figure supplement 1d). Next, to investigate the biological consequence of the evolutionary differences in overlapping IL-6/IFN signaling, we reanalyzed cross-species type I IFN regulation from the ‘mammalian interferome’ database (Shaw et al., 2017). As expected, the conserved ‘IFN/IL6-shared’ genes CXCL10/CXCL9/STAT1/STAT2 displayed higher fold-changes upon type I IFN treatment across all 10 species (from chicken to human, Figure 6b, top panel). Interestingly, the ‘IL-6/STAT3’ pathway genes IL6, STAT3 and SOCS3 were also significantly upregulated while IL6R was significantly down-regulated (Figure 6b, top panel) in the same experimental setting. Among CD34/myeloid differentiation genes, ICSBP/IRF8 and CD38 were strongly upregulated, but only in 4/10 and 2/10 species, respectively, while ELF1 was homogeneously and significantly upregulated in 9/10 species (Figure 6b, top panel). These results suggest that type I IFN consistently regulates expression of IL-6 signaling and myeloid-associated genes in different species. However, among the entire IL6/IL6R/CEBP myeloid gene set, CEBPB was the topmost variable ISG across mammalian evolution (CV >1000%, Figure 6b, bottom panel). Remarkably, type I IFN-induced upregulation of both CEBPB and CEBPD, previously identified as NF-IL6 and NF-IL6β, respectively (Ramji and Foka, 2002) was present only in humans and lacking in all other mammals investigated (Figure 6b, bottom panel, inset). Mechanistically, ChipSeq analysis of IFN-treated human CD14+ monocytes corresponding to regions with active chromatin (DNase Hypersensitivity Sites, DHS and H3K27 acetylation, not shown) confirmed the existence of functional STAT1 peaks in CEBPB and CEBPD (Figure 6c, top and middle panels, denoted by vertical blue lines). These peaks correlated with increased downstream transcription in CD14+ cells, as compared to purified CD34+ cells (Figure 6c, RNA-seq). In agreement with our findings (represented in Figure 6b, bottom panel, inset), only 3 out of 11 (27%) STAT1 binding peaks in CEBPB and CEBPD were found in conserved regions (Conservation Birds-Mammals line, Figure 6c), while 6 out of 7 (86%) STAT1 peaks were conserved in CXCL9 and CXCL10 genes (Figure 6c, bottom panel). Interestingly, transcriptional regulation of CEBPB and CEBPD in humans and macaques, but not mouse cells stimulated with double-stranded RNA, which mimics a viral infection (Figure 6—figure supplement 1a), were also observed in an independent data set (Hagai et al., 2018). As expected, CXCL9 and CXCL10 responses are conserved in dsRNA-stimulated cells from humans, macaques and mouse (Figure 6—figure supplement 1a). Thus, transcriptional induction of CEBPB and CEBPD controlled by IL-6- and type I IFN-signaling appears as a relatively recent event in mammalian and primate evolution.
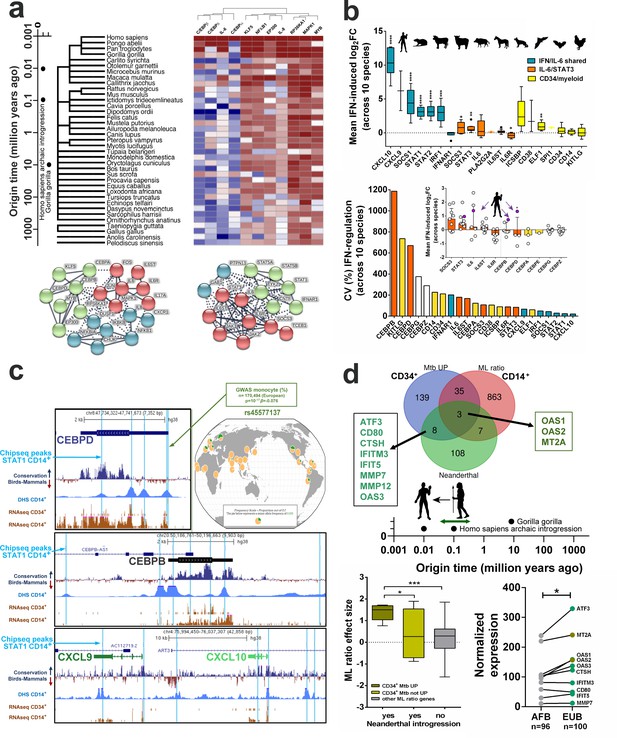
Evolutionary recent and human-specific genetic adaptation link IL6/IL6R/CEBP gene module with monocyte expansion and TB pathogenesis.
(a) Heat map showing CEBPB network generated by STRING co-occurrence protein conservation scores across primates, mammals, birds and reptiles. Note only CEBPB and CEBPA differ strongly among hominids, while CEBPA/CEBPB/CEBPD vary significantly throughout primate and mammalian evolution, as compared to highly conserved STAT1/STAT3 (Figure 5—figure supplement 1d). (b) Top panel: Highly conserved type I IFN upregulation of ‘IFN/IL6-shared’ genes from humans to birds (derived from http://isg.data.cvr.ac.uk/) (Shaw et al., 2017), as compared to ‘IL6/STAT3’ and ‘CD34/myeloid differentiation’ genes. Bottom panel: CEBPB and CEBPD displays highest variation, and CXCL10 the lowest variation in type I IFN transcriptional regulation across human-mammalian-bird evolution. Inset, CEBPB and CEBPD selectively acquired type I IFN upregulation in humans (filled circles); ** p-value<0.01 and * p-value<0.05 represent CEBPB and CEBPD values, respectively for humans versus the other species. (c) ChipSeq analysis of STAT1-binding peaks in CEBPD (top panel), CEBPB (middle panel), CXCL9 and CXCL10 (bottom panel) in IFN-stimulated human monocytes, corresponding to regions with active chromatin (DNase Hypersensitivity Sites, DHS) and correlating with increased downstream transcription in CD14+ monocytes, as compared to purified CD34+ cells. Conservation analysis among >40 vertebrates (phyloP [Pollard et al., 2010], from chicken to human, analogous to Figure 5b) indicates STAT1 peaks are mostly conserved in CXCL9/CXCL10 (6/7) but not in CEBPD/CEBPB (3/11). (d) Top panel: overlap between human genes with significant Neanderthal introgression (Enard and Petrov, 2018; Quach et al., 2016), genes differentially expressed in Mtb-exposed CD34+ cells (CD34+ Mtb UP) and the ‘ML ratio’ gene set. Bottom left panel: OAS1, OAS2 and MT2A transcripts presented significantly higher effect sizes upon ML ratios, corresponding to monocyte expansion, as compared to other introgressed genes (p<0.05) and to all other genes shown to regulate ML ratio in vivo (p<0.001). Bottom right panel: normalized expression of introgressed genes found in CD34+Mtb UP (Venn diagram) in TLR1/2 agonist-treated monocytes from a cohort of matched Belgian individuals of European (EUB) vs. African (AFB) descendance, with documented presence or absence of Neanderthal introgression (Quach et al., 2016), respectively. p-value<0.05, ** p-value<0.01, *** p-value<0.001, **** p-value<0.0001.
-
Figure 6—source data 1
Raw data from Figure 6.
- https://doi.org/10.7554/eLife.47013.025
Genome-wide association studies (GWAS) connect the IL6/IL6R/CEBP gene module with monocyte expansion in TB disease
Since genetic susceptibility and transcriptional responses to intracellular pathogens have shown significant links to Neanderthal introgression in populations of European and Asian descent (Dannemann et al., 2017; Quach et al., 2016), we next explored enrichment for introgression in the IL6/IL6R/CEBPB and ‘ML ratio’ gene sets. As shown in Figure 6d (Figure 2—source data 1), eleven genes with Neanderthal introgression were significantly upregulated in our Mtb-exposed CD34+ cells transcriptome (enrichment p<0.0001). Of those, OAS1, OAS2 and MT2A transcripts had significantly higher effect sizes upon ML ratios, as compared to other introgressed genes (p<0.05) and to all other genes shown to regulate ML ratio in vivo (p<0.001, Figure 6d). This finding was confirmed in a recently published data set (n = 198) of purified microbial-exposed CD14+ monocytes from a Belgian cohort of European (EUB) and African (AFB) descendance, with documented presence or absence of Neanderthal introgression, respectively (Quach et al., 2016). Strikingly, 9 out of 11 introgressed genes enriched during Mtb-triggered monocyte differentiation (Figure 6d, Venn diagram) were significantly upregulated in TLR1/TLR2-stimulated (Figure 6d, right panel), but not unstimulated monocytes (not shown). These findings suggest pathogen exposure may enhance gene pathways recently selected during hominid evolution linked to monocyte expansion.
We next interrogated whether the IL6/IL6R/CEBP gene module was linked with monocyte expansion in several large published data sets of standing human variation. Two large GWAS studies (Astle et al., 2016; Kanai et al., 2018) containing >230,000 individuals have identified single-nucleotide polymorphisms (SNPs) in or adjacent to IL6R, CEBPA-CEBPD-CEBPE and ICSBP/IRF8 genes as significantly associated to blood monocyte counts (Supplementary file 2 and ranked in Figure 7a as monocyte count GWAS). Moreover, gene-specific z-scores for human polygenic adaptation to pathogens in 51 different populations worldwide (Daub et al., 2013) were positive, representing higher levels of population differentiation, for all genes in our proposed IL6/IL6R/CEBP myeloid differentiation module (except CXCL10, Figure 7a and Supplementary file 3). Lastly, we examined whether myeloid differentiation genes identified in this study are found in GWAS TB susceptibility genes. A significant enrichment (p<0.0001) for differentially expressed genes from our Mtb-exposed CD34+ transcriptome and TB susceptibility GWAS/candidate genes (18 out of 172 genes, including IL6, STAT1 and CD14) was also observed (Figure 6—figure supplement 1b). Similarly, a significant (p<0.0001) overlap was found for the IL6/IL6R/CEPB module and TB genetic susceptibility (six shared genes CD14, CXCL10, IL6, IL6R, IRF1 and STAT1, Figure 6—figure supplement 1b).
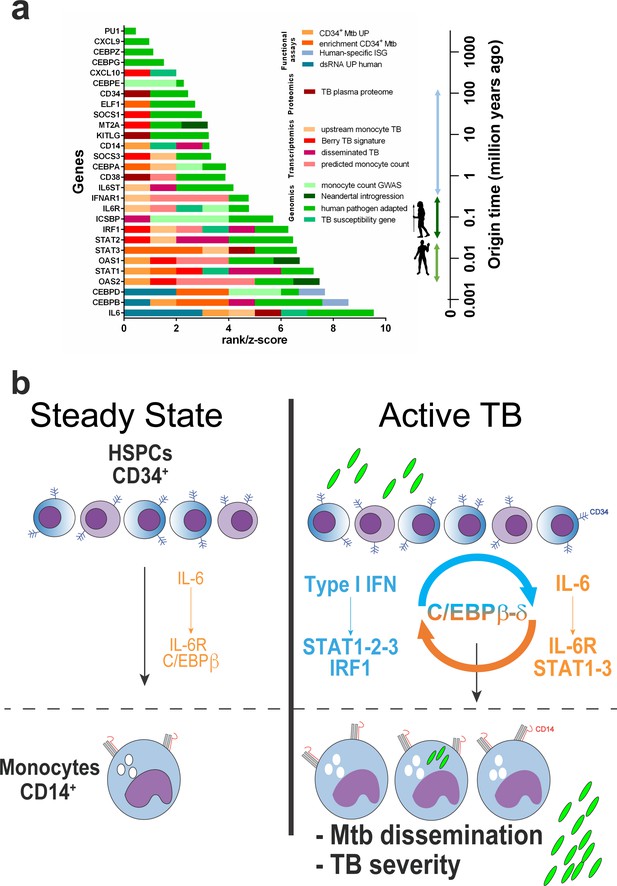
Compiled multi-level evidence for an IL6/IL6R/CEBP gene module linking CD34+ myeloid differentiation to TB pathogenesis and disease severity.
(a) Ranks and scores were determined as 0–1 (presence-absence in data set) or 0-1-2-3, according to enrichment analysis or differential gene expression (quartiles); z-scores were obtained from Daub et al.55 (b) Proposed model for C/EBPβ and C/EBPδ acting as a bridge in the type I IFN and IL-6 feed-forward loop exploited by Mtb to induce monocyte differentiation and TB disease severity (details in the text).
-
Figure 7—source data 1
Raw data from Figure 7.
- https://doi.org/10.7554/eLife.47013.027
As ranked in Figure 7a, 24 out of 28 members of this gene module display a genome-wide, transcriptomic, proteomic or functional association to human TB, being strongest for IL6 and its downstream signaling TFs CEBPB and CEBPD, demonstrated in 5–6 independent data sets each. Collectively, our multi-level-based evidence suggests Mtb exploits an evolutionary recent IFN/IL-6/IL-6R/CEBP axis linked to monocyte expansion and human TB disease.
Discussion
Emerging evidence has suggested that Mtb establishes an infectious niche in the human bone marrow during active TB, which is associated with altered numbers of leukocytes in the periphery (Das et al., 2013; Mert et al., 2001; Naranbhai et al., 2015; Rogers, 1928; Schmitt et al., 1977; Tornack et al., 2017; Wang et al., 2015). In the present study, we observed that Mtb consistently stimulated myeloid differentiation molecules in CD34+ cell cultures from three different human tissues, namely: bone marrow, peripheral blood or cord blood samples. Employing a purified cord-blood-derived CD34+ culture cell system, we observed that Mtb enhances IL-6R-mediated myeloid differentiation by human primary CD34+ cells in vitro. Importantly, IL-6/IL-6R downstream molecules such as C/EBPβ, C/EBPδ, STAT3 and their targets were significantly enriched in cell transcriptomes from active TB patients as well as were positively correlated with disease severity. Therefore, our data expands previous studies and raise a scenario in which Mtb skews myeloid development, mediated by IL-6/IL-6R signaling, as a key step in human TB pathogenesis.
While Mtb enhanced IL6 expression in purified CD34+ cell cultures from all donors, IFNA and IFNB mRNA were detected in some but not all donors. However, ISGs were highly enriched in the bacteria-exposed samples suggesting that although low/undetectable amounts of type I IFN were produced in infected cell cultures (Rodero et al., 2017), these cytokines were present in the cell culture (Figure 4—figure supplement 1h). Furthermore, our results show that live Mtb is a potent stimulus to induce ISGs (Figure 6—figure supplement 1c - Figure 2—source data 1) and myeloid differentiation in primary human CD34+ cells. However, while heat killed mycobacteria induced IL-6 production by CD34+ cells, it poorly stimulated STAT1, CEBPB and differentiation cell surface molecules by progenitor cells as well as CD14+ monocyte levels. Interestingly, although IL-6R signaling was involved in both myeloid differentiation and Mtb growth by CD34+ cell cultures, type I or type II IFN signaling were not. These results suggest that monocyte maturation is connected to Mtb proliferation in vitro and could explain why the effects of anti-IL6R antibodies on cellular differentiation inhibited bacteria growth (Figure 4f–i). Although the ISG gene set was enriched in Mtb-exposed CD34+ cells, our data suggest type I or type II IFN signaling appear not to mediate Mtb-enhanced monocyte development in vitro. Collectively, this evidence suggests that unknown activities of live pathogen infection regulate myeloid differentiation involving an IL-6R-mediated process and implies cross-talking of regulatory pathways between live Mtb, IL-6 and IFN signaling to boost myeloid differentiation of CD34+ cells. At the molecular level, it has been reported that IFN-induced C/EBPβ triggers gamma-activated transcriptional elements (GATE) sequences independent of STAT1 (Li et al., 2007), suggesting the existence of cooperative and/or redundant roles of IL-6 and IFN signaling in different molecular settings. The mechanisms by which endogenous IL-6, IFN-α/β and live Mtb interplay to enhance C/EBP-mediated myeloid differentiation of HSPCs require further investigation. Of note, in our previously characterized cohort of multiple sclerosis patients (Menezes et al., 2014; Van Weyenbergh et al., 2001), IFN-β therapy in vivo did not significantly change monocyte or lymphocyte counts, nor did it increase the ML ratio after three months of treatment in patients with documented clinical response (data not shown), supporting the idea that type I IFN by itself is not sufficient to cause monocyte expansion in vivo.
It has not been determined how mature myeloid cell populations from active TB patients acquire the ‘ISG’ signature. While monocytes and other cells may encounter Mtb-associated inflammatory stimuli in infected tissues (e.g. lungs and liver), our data suggest the possibility that Mtb may activate these cells during their development in the bone marrow. We have not directly addressed whether circulating monocytes/granulocytes acquire their phenotype in the bone marrow, during development of myeloid progenitors in vivo. Nevertheless, a recent study by Norris and Ernst (2018) demonstrated increased monocyte egress from the bone marrow in a murine model of Mtb infection. Considering mycobacteria (Arts et al., 2018; Das et al., 2013; Joosten et al., 2018; Mert et al., 2001; Mitroulis et al., 2018) can access the bone marrow and stimulate IL-6, it is possible that individuals draining higher amounts of Mtb into the bone marrow display increased inflammatory alterations including IL-6R-mediated myelopoiesis, upregulation of IFN-stimulated responses and amplified disease severity. Likewise, we found that enrichment of the IL6/IL6R/CEBP axis positively correlated with systemic disease such as lymph node and extrapulmonary TB (Figure 5f,g).
Several independent studies have also indicated a detrimental role of ‘IFN and IFN-induced genes’ during Mtb infection in human TB (Berry et al., 2010; Bustamante et al., 2014; Dos Santos et al., 2018; Novikov et al., 2011; Scriba et al., 2017; Zhang et al., 2018) and murine models (Antonelli et al., 2010; Manca et al., 2001). Our results expand these previous studies, revealing a novel IL6/IL6R/CEBP gene module and its link to monocyte development, mycobacterial dissemination and TB disease severity. Furthermore, as evidenced by Scriba et al. (2017) and re-analyzed in the present study (Figure 5c,d), both IFN and IL6 pathways are early events in TB pathogenesis, detectable in the plasma proteome >6 months before diagnosis. Nevertheless, a pivotal role for IL6/IL6R signaling has not been evident from previous ‘omics’ approaches. While IL-6 signaling partially overlaps with type I IFN responses (Figure 5a,b), possibly due to their shared ability to activate STAT1 and STAT3 (Ho and Ivashkiv, 2006), whole blood transcriptomic analyses in TB are predominated by an ‘IFN-inducible neutrophil signature’ (Berry et al., 2010). Therefore, the high numbers of neutrophils in the blood possibly mask differential expression of other gene sets in less frequent populations, such as monocytes, monocyte subsets and, in particular, Lin-CD34+ cells.
Most ISGs present in the whole blood Berry TB signature (Berry et al., 2010), the Scriba TB plasma proteome (Scriba et al., 2017) and the Naranbhai et al. ML ratio gene set (Naranbhai et al., 2015) displayed cross-species type I IFN-induction throughout mammalian evolution (Figure 6b). Despite a shared STAT1/STAT3 activation by type I IFN and IL-6, homeostatic activation of C/EBPβ is mostly IL-6-specific, as evidenced by data mining and STRING analysis (Figure 6a), in keeping with its original description as NF-IL6 (Akira et al., 1990). Across species, CEBPB and CEBPD are among the highly variable ISG of the entire IFN/IL-6/CD34/myeloid gene set (Figure 6b). Likewise, although type I IFN-induced IL6 and STAT3 transcription are conserved in all mammalian species studied, IFN-inducibility of CEBPB and CEBPD mRNA appeared to be recently acquired in primate evolution. In line with our observations, IL6, CEBPB and SPI1/PU1 genetic polymorphisms have been previously associated with TB susceptibility (Zhang et al., 2014; Zhang et al., 2012). In addition, a recently identified trans e-QTL (rs5743618) (Quach et al., 2016) in TLR1, a gene with peak Neanderthal introgression (Dannemann et al., 2017; Enard and Petrov, 2018; Hagai et al., 2018; Quach et al., 2016) has been associated to TB susceptibility in several populations worldwide (Barletta-Naveca et al., 2018; Naderi et al., 2016; Qi et al., 2015). Biological pathway analysis of genes significantly regulated in trans of rs5743618 revealed a significant enrichment of IL-6/STAT3 signaling and IL6 as the most connected gene (data not shown). In agreement, Mtb-induced macrophage IL-6 production, among other cytokines, can be predicted on the basis of strong genetic components as recently reported by Bakker et al. (2018) in a large GWAS/immunophenotyping cohort study. By employing a data-driven multi-level analysis from large cohorts, we expand these previous observations and revealed significant genetic links shared between IL-6/IL-6R/CEBP signaling, CD34+ myeloid differentiation, monocyte homeostasis and TB susceptibility (compiled in Figure 7a). Together, these findings favor the hypothesis that such genetic changes have undergone stepwise mammalian, primate and recent human selection, including Neanderthal introgression and worldwide population-specific pathogen adaptation.
In summary, our observations suggest that Mtb boosts myeloid differentiation by exploiting a feed-forward loop between IL-6 and type I IFN molecular networks, bridged by C/EBPβ (and C/EBPδ) (Figure 7b). Yet, further experiments will define the precise mechanisms of crosstalk between IFN, IL-6 and CEBP family members, specifically CEBPβ and CEBPδ, during natural Mtb infection. While this question merits direct investigation, nonetheless, the use of IL-6R blockade as an adjunct therapy to treat multi-drug resistant severe TB has been proposed (Okada et al., 2011; Zumla et al., 2016). Thus, the present study provides evidence of a novel host-directed target for therapeutic intervention in a major human disease.
Materials and methods
Reagent type (species) or resources | Designation | Source or reference | Identifiers | Additional information |
---|---|---|---|---|
Strain (Mycobacterium tuberculosis) | H37Rv | ATCC | ||
Strain (Mycobacterium tuberculosis) | Mtb-CS267 | Clinical Isolate | This study | |
Cell Line (Homo sapiens) | Human Cord Blood (CB) purified CD34+ cells | STEMCELL Technologies | Catalog#70008.5 | Cell line maintained in StemSpan II Expansion Media - STEMCELL Technologies- Catalog#09605 |
Cell (Homo sapiens) | Peripheral blood mononuclear cell (PBMC) | Cells maintened in RPMI 1640 complete, Sigma-Aldrich – Catalog#R8758 | ||
Chemical Compound, drug | Middlebrook 7H10 agar | BD Biosciences | Catalog# 262710 | Supplemented with 10% Oleic Acid,Albumin, Dextrose, Catalase (Sigma-Aldrich – Catalog# M0678-1VL) |
Chemical Compound, drug | L-Glutamine (200 mM) | Sigma-Aldrich | Catalog# 25030081 | |
Chemical Compound, drug | Sodium Pyruvate (100 mM) | Life Technologies. | Catalog# 11360070 | |
Biological Sample | Genomic DNA, Mycobacterium tuberculosis, Strain H37Rv | This study | Dosage: 10 μg/ml | |
Chemical Compound, drug | Mycobacterium tuberculosis, Strain H37Rv, Purified Lipoarabinomannan (LAM) | BEI Resources | Catalog# NR-14848 | Dosage: 5 µg/mL |
Peptide, Recombinant protein | Recombinant Human Interleukin 6 (rh IL-) | ImmunoTools | Catalog# 11340064 | |
Antibody | FITC Anti-Lineage 1, human: CD3 clone SK7, CD16 clone 3G8, CD19 clone SJ25C1, CD20 clone L27, CD14 clone MφP9, CD56 clone NCAM. | BD Biosciences | Catalog# 340546 | (1:30) |
Antibody | PE Anti-human CD34, clone 581. | BD Biosciences | Catalog# 555822 | (1:20) |
Antibody | FITC Anti-human CD34, clone 8G12. | BD Biosciences | Catalog# 345801 | (1:50) |
Antibody | PerCP Anti-human CD34, clone 581. | Biolegend | Catalog# 343519 | (1:20) |
Antibody | PECy7 Anti-human HLA-DR, clone L243 | Biolegend | Catalog# 307615 | (1:200) |
Antibody | Bv510 Mouse Anti-Human HLA-DR, clone G46-6 | BD Horizon | Catalog# 563083 | (1:100) |
Antibody | APC Anti-human CD38, clone HIT2 | Biolegend | Catalog# 303510 | (1:100) |
Antibody | Bv421 Anti-Human CD64, clone 10.1. | BD Biosciences | Catalog# 562872 | (1:100) |
Antibody | FITC Anti-human CD10, clone HI10A | BD Biosciences | Catalog# 340925 | (1:50) |
Antibody | Alexa Fluor 488 Anti-human CD14, clone M5E2. | Biolegend | Catalog# 301817 | (1:50) |
Antibody | APCCy7 Anti-mouse/human CD11b, clone M1/70 | Biolegend | Catalog# 101226 | (1:100) |
Antibody | V450 Anti-human CD14, clone MφP9 | BD Biosciences | Catalog# 560350 | (1:100) |
Antibody | PE Anti-human CD66b, clone G10F5. | Biolegend | Catalog#305106 | (1:100) |
Antibody | APCCy7 Anti-human BDCA1, clone L161. | Biolegend | Catalog# 331520 | (1:200) |
Antibody | FITC Anti-human CD41a, clone 6C9. | ImmunoTools | Catalog# 21330413 | (1:50) |
Antibody | APC Anti-human BDCA2, clone 201A | Biolegend | Catalog# 354205 | (1:50) |
Antibody | Bv510 Anti-human BDCA3, clone 1A4. | BD Biosciences | Catalog# 563298 | (1:100) |
Antibody | PE Anti-human CD123, clone 7G3. | BD Biosciences | Catalog# 554529 | (1:20) |
Antibody | APC Anti-human CD16, clone 3G8. | BD BiosciencesCatalog# | 561248 | (1:50) |
Antibody | V450 Anti-human CD64, clone 10.1. | BD Biosciences | Catalog# 561202 | (1:20) |
Antibody | FITC Anti-human CD3, clone UCHT1. | Biolegend | Catalog# 300440 | (1:100) |
Antibody | FITC Anti-human CD19, clone 4G7. | BD Biosciences | Catalog# 347543 | (1:50) |
Antibody | Alexa Fluor 488 Anti-human CD14, clone M5E2. | BD Biosciences | Catalog# 561706 | (1:50) |
Antibody | PerCP-Cy5.5 Anti-human CD34, clone 8G12. | BD Biosciences | Catalog# 347203 | (1:25) |
Antibody | PE Anti-human IL-6, clone 8C9. | ImmunoTools | Catalog# 21670064 | (1:10) |
Antibody | FITC Anti-human CD56, clone NCAM16.2. | BD Biosciences | Catalog# 345811 | (1:100) |
Antibody | FITC Anti-human CD16, clone HI16a. | ImmunoTools | Catalog# 21810163 | (1:100) |
Antibody | Monoclonal Anti-STAT1 (phospo Y701), clone M135. | Abcam | Catalog# ab29045 | (1:1000) |
Antibody | Monoclonal Anti-STAT1, clone SM1. | Abcam | Catalog# ab3987 | (1:1000) |
Antibody | Polyclonal Anti-C/EBPβ | Santa Cruz Biotechnology | Catalog# sc-150 | (1:250) |
Antibody | Neutralizing Anti-human IFNAR2, clone MMHAR-2 | PBL Assay Science | Catalog# 21370–1 | Dosage: 1 µg/mL |
Antibody | Monoclonal, Anti-IFN-γ, clone B27. | ImmunoTools | Catalog# 21853531 | Dosage: 10 µg/mL |
Antibody | Anti-IL6, Tocilizumab. | Roche | Dosage: 1 µg/mL | |
Antibody | Monoclonal Anti-beta actin | Abcam | Catalog# mAbcam 8226 | (1:5000) |
Chemical Compound, drug | Flexible Viability Stain 450 | BD Horizon | Catalog#562247 | (1:1000) |
Chemical Compound, drug | Carbol Fuchsin | Sigma-Aldrich | Catalog# C4165 | |
Chemical Compound, drug | Methylene Blue | Sigma-Aldrich | Catalog# 03978 | |
Chemical Compound, drug | Hoechst 33342 | Immunochemistry technologies | Catalog# 639 | |
Commercial assay, kit | M-PER Mammalian Protein Extraction Reagent | Thermo Fisher Scientific | Catalog# 78501 | |
Commercial assay, kit | cOmplete ULTRA Tablets, Mini, EASYpack Protease Inhibitor Cocktail | Roche | Catalog# 05 892970001 | |
Commercial assay, kit | High-Capacity cDNA Reverse Transcription Kit | Applied Biosystems | Catalog# 4368814 | |
Chemical Compound, drug | TRIzol LS Reagent | Invitrogen | Catalog# 10296010 | |
Commercial assay, kit | NuGEN - Trio low input RNA-seq | NuGEN | Catalog#0507–08 | |
Software, algorithm | FlowJo software v. 10.1 | TreeStar | FlowJo, RRID:SCR_008520_ | https://www.flowjo.com/ |
Software, algorithm | GraphPad Prism 6 Software | GraphPad | GraphPad Prism, RRID:SCR_002798 | https://www.graphpad.com/ |
Reagents
Mtb Ara-LAM was obtained from BEI Resources and used at 5 µg/mL. Mtb H37Rv genomic DNA was obtained from 28 days colonies growing in Löwenstein–Jensen medium by CTAB method as previously described (Yamashiro et al., 2016). Recombinant human (rh) IL-6 was purchased from Immunotools. Anti-IFNAR2A (clone MMHAR-2, PBL) and anti-IFN-γ (clone B27, Immunotools) neutralizing antibodies were used at 1 and 10 µg/mL, respectively and anti-IL-6R (Tocilizumab, Roche) was used at 1 µg/mL. Fluorescent dye Syto24 was obtained from Thermo Fisher Scientific.
Mycobacteria cultures
Request a detailed protocolThe virulent laboratory H37Rv Mtb strain and the clinical Mtb isolate (Mtb-CS267) were maintained in safety containment facilities at LACEN and UFSC as described elsewhere (Yamashiro et al., 2016). Briefly, Mtb was cultured in Löwenstein-Jensen medium (Laborclin) and incubated for 4 weeks at 37°C. Prior to use, bacterial suspensions were prepared by disruption in saline solution using sterile glass beads. Bacterial concentration was determined by a number 1 McFarland scale, corresponding to 3 × 108 bacteria/mL.
Subjects samples, cells and Mtb infections
Request a detailed protocolThis study was approved by the institutional review boards of Universidade Federal de Santa Catarina and The University Hospital Prof. Polydoro Ernani de São Thiago (IRB# 89894417.8.0000.0121). Informed consent was obtained from all subjects. Peripheral blood and bone marrow mononuclear cells were obtained using Ficoll-Paque (GE) in accordance with the manufacturer’s instructions. Briefly, blood collected in lithium-heparin containing tubes was further diluted in saline solution 1:1 and added over one volume of Ficoll-Paque reagent. The gradient was centrifuged for 40 min at 400 x g, 20°C. The top serum fraction was carefully removed, the mononuclear fraction was harvested and washed once in a final volume of 50 mL of saline solution for 10 min at 400 x g, 20°C. Subsequently, cell pellet was suspended and washed twice with 20 mL of saline solution for 10 min at 200 x g, 20°C, to remove platelets. Cells were then suspended to the desired concentration in RPMI 1640 (Life Technologies) supplemented with 1% fresh complement inactivated (30 min at 56°C) autologous serum, 2 mM L-glutamine (Life Technologies), 1 mM sodium pyruvate (Life Technologies) and 25 mM HEPES (Life Technologies). Human Cord Blood (CB) purified CD34+ cells from five different donors were obtained from STEMCELL Technologies and resuspended in StemSpan Expansion Media – SFEM II (STEMCELL Technologies) according to manufacturer’s instruction. Optimal cell density for replication was 5 × 104 CD34+ cell/mL. In a set of experiments, CD34+ cells were further enriched using a cell sorter (FACSMelody, BD). Following 4 days of expansion, cells were washed and diluted in SFEM II media without cytokine cocktail to the desired concentration. Culture purity was assessed by FACS and showed more than 90% of CD34+ events after expansion. For in vitro infection experiments, 1 McFarland scale was diluted in media to fit the desired multiplicity of infection (MOI). For each experiment, bacteria solution was plated in Middlebrook 7H10 agar (BD Biosciences) supplemented with 10% Oleic Acid Albumin Dextrose Complex (OADC) and incubated at 37°C to confirm initial bacteria input. In a set of experiments, 1 McFarland scale was incubated with 500 nM of Syto24 dye as described previously (Yamashiro et al., 2016). In some experiments, H37Rv Mtb was heat killed (HK) at 100°C for 30 min. Leishmania infantum promastigotes were kindly provide by Ms. Karime Mansur/UFSC and Dr. Patrícia Stoco/UFSC and used at MOI = 3. In cytokine/cytokine neutralizing experiments, cells were pretreated with anti-IFNAR2 (1 µg/mL), anti-IFN-γ (10 µg/mL) or anti-IL-6R (1 µg/mL) for 1 hr and exposed to Mtb (MOI3). Following different time points post-infection, cells were harvested and centrifuged at 400 x g for 10 min, 20°C. Supernatants were then stored at −20°C, cells washed once in sterile saline solution and lysed by using 200 µL of 0.05% Tween 80 solution (Vetec) in sterile saline. Cell lysates were diluted in several concentrations (10−1 to 10−5), plated onto Middlebrook 7H10 agar (BD Biosciences) supplemented with OADC 10% and incubated at 37°C. After 28 days, colony-forming units (CFU) were counted and the results were expressed graphically as CFU/mL.
Microscopy experiments
Request a detailed protocolAfter different time points post-infection, cells were washed and fixed with PFA 2% overnight at 4°C. Subsequently, cells were washed with sterile water solution and adhered into coverslips by cytospin centrifugation. Samples were then fixed with methanol for 5 min, washed with sterile water and stained with carbol-fuchsin (Sigma) for 2 min. Samples were washed once with sterile water and counterstaining was done with methylene blue dye (Sigma) for 30 s. Coverslips were fixed in slides with Permount mounting medium (Sigma) and examined using Olympus BX40 microscope and digital camera Olympus DP72. Quantification was performed by enumeration of number of infected cells or “cytoplasm-rich cells, defined as cells bigger than 10 um and with approximately 2:1 cytoplasm/nucleus ratio. Cells were counted in at least 10 fields from two different experiments and plotted as % of events. Syto24-stained Mtb was visualized in CD34+ cells by using confocal fluorescence-equipped inverted phase contrast microscope and photographed with a digital imaging system camera. Briefly, 1 × 105 CD34+ cells were seeded in 24-well plate and infected with Mtb syto24, MOI3, for 4 hr. Further, cells were washed, fixed with PFA 2% and adhered into coverslip by cytospin centrifugation. For nucleus visualization, cells were stained with Hoechst 33342 (Immunochemistry technologies) for 2 hr. Cells were after washed and mounted for analysis in Leica DMI6000 B confocal microscope.
Immunoblotting
Request a detailed protocolCD34+ cells were seeded at 3 × 105 cells in 24-well plate and infected with Mtb (MOI3). After 5 days of infection, cells were centrifuged at 4°C, pellet was lysed using M-PER lysis buffer (Thermo Fisher Scientific) containing protease inhibitors (Complete, Mini Protease Inhibitor Tablets, Roche) and protein extracts were prepared according to manufacturer’s instructions. For Western blot, 15 µg of total protein were separated and transferred to nitrocellulose difluoride 0.22 µm blotting membranes. Membranes were blocked for 1 hr with TBST containing 5% w/v BSA and subsequently washed three times with TBST for 5 min each wash. Further, membranes were then probed with anti-pSTAT1 Y701 1:1000 (M135 – Abcam), anti-STAT1 1:1000 (SM1 – Abcam), anti-C/EBPβ 1:250 (sc-150 – Santa Cruz) or anti-β-actin 1:5000 (8226 – Abcam) primary antibodies diluted in 5% w/v BSA, 0.1% tween 20 in TBS, at 4°C with gentle shaking overnight. Membranes were washed with TBST, incubated in secondary HRP-linked Ab for 2 hr at room temperature, washed and chemiluminescence developed using ECL substrate (Pierce). Relative expression was normalized with β-actin control and pixel area was calculated using ImageJ software.
Flow cytometry
Request a detailed protocolPBMC and bone marrow mononuclear cells were seeded at 5 × 105 cells per well in a final volume of 200 µL. After 4 hr of resting at 37°C with 5% CO2, cells were infected with Mtb (MOI3) for 72 hr, unless indicated otherwise. Cells were detached from the plate by vigorous pipetting, centrifuged at 450 x g for 10 min and washed twice in saline solution and stained with fixable viability stain FVS V450 (BD Biosciences) at the concentration 1:1000 for 15 min at room temperature. Cells were then washed with FACS buffer (PBS supplemented with 1% BSA and 0.1% sodium azide) and incubated with 10% pooled AB human serum at 4°C for 15 min. The following antibodies were used in different combinations for staining:
Staining of human CD34+ in PBMC: anti-Lin1(CD3, CD14, CD16, CD19, CD20,CD56) (FITC, clones MφP9, NCAM 16, 3G8, SK7, L27, SJ25-C1), anti-CD34 (PE, PE, clone 581), anti-CD34 (FITC, 8G12), anti-CD34 (PerCP, clone 581), anti-HLA-DR (PE-Cy7, clone L243), anti-HLA-DR (Bv510, clone G46-6), anti-CD38 (APC, clone HIT2), anti-CD4 (APC-Cy7,GK1.5), anti-CD64 (Bv421, clone 10.1), anti-CD10 (FITC, clone HI10A), anti-CD14 (V450, clone MoP9), anti-CD14 (Alexa488, clone M5E2) were added at titrated determined concentration and incubated for 40 min at 4°C.
Staining of CB CD34+ cells: anti-CD34 (PE, clone 581), anti-CD11b (APCCy7, clone M1/70), anti-CD4 (APC-Cy7, clone GK1.5), anti-CD64, (Bv421, MoP9), anti-CD14 (V450, clone MoP9) anti-CD14 (Alexa488, clone M5E2), anti-CD66b (PE, clone G10F5), anti-BDCA1 (APC-Cy7, clone L161), anti-CD41a (FITC, clone 6C9), anti-BDCA2 (APC, clone 201A), anti-BDCA3 (Bv510, clone 1A4), anti-Clec9A (A700, clone FAB6049P), anti-CD123 (PE, clone 7G3), anti-CD16 (APC, clone 3G8) were added at titrated determined concentrations and incubated for 40 min at 4°C. In a set of experiments, PBMCs were exposed to live Mtb, HK Mtb or LPS (100 ng/mL) for 24 hr and the Golgi Plug protein transport inhibitor (BD Biosciences) was added for the last 6 hr according to manufacturer’s instructions. Then, cells were surface stained with FITC-Lin (FITC-anti-CD3, Alexa Fluor 488-anti-CD14, FITC-anti-CD16, FITC-anti-CD19, FITC-anti-CD56) and PerCP/Cy5.5-anti-CD34, followed by permeabilization and PE-anti-IL-6 (clone 8C9) staining. All cells were subsequently washed with FACS buffer and resuspended in 2% PFA. Cells were acquired on BD FACS Verse with FACSuite software. Analysis were performed using FlowJo software v. 10.1 (TreeStar).
Real-time quantitative PCR
Request a detailed protocolTotal RNA was extracted from CD34+ cells exposed or not with Mtb. RNA was extracted after 1, 3 and 5 days of infection using TRIzol reagent (Thermo) according to manufacturer’s instruction. Using 1 µg of RNA, cDNA was produced with a High-Capacity cDNA Reverse Transcription Kit (Applied Biosystems) and 2 µL of 1:8 diluted product was used to the quantitative PCR reaction in a final volume of 10 µL. qPCR reactions were performed using the primers for: IFNA2A F: 5’-TTGACCTTTGCTTTACTGGT-3’, R: 5’-CACAAGGGCTGTATTTCT TC-3’. IL6 F: 5’- CCACACAGACAGCCACTCAC-3’, R: 5’-AGGTTGTTTTCTGCCAGTGC-3’. IFNB F: 5’- AAACTCATGAGCAGTCTGCA-3’, R: 5’-AGGAGATCTTCAGTTTCGGAGG-3’. IFNG F: 5’- TCAGCTCTGCATCGTTTTGG-3’, R: 5’-GTTTCCATTATCCGCTACATCTGAA-3’. IFI16 F: 5’-ACTGAGTACAACAAAGCCATTTGA-3’, R: 5’-TTGTGACATTGTCCTGTCCCCAC-3’. MX1 F: 5’-ATCCTGGGATTTTGGGGCTT-3’, R: 5’-CCGCTTGTCGCTGGTGTCG-3’. ISG15 F: 5’-TCCTGGTGAGGAATAACAAGGG-3’, R: 5’-CTCAGCCAGAACAGGTCGTC-3’. CXCL8 F: 5’-GAGGTGATTGAGGTGGACCAC-3’, R: 5’-CACACCTCTGCACCCAGTTT-3’. IL1RA F: 5’-ATGGAGGGAAGATGTGCCTGTC-3’, R: 5’-GTCCTGCTTTCTGTTCTCGCTC-3’. GRB2 F: 5’-GAAATGCTTAGCAAACAGCGGCA-3’, R: 5’-TCCACTTCGGAGCACCTTGAAG-3’. STAT1 F: 5’-ATGGCAGTCTGGCGGCTGAATT-3’, R: 5’-CCAAACCAGGCTGGCACAATTG-3’. CEBPA F: 5’-TGGACAAGAACAGCAACGAGTA-3’, R: 5’-ATTGTCACTGGTCAGCTCCAG-3’. CEBPB F: 5’-TGGGACCCAGCATGTCTC-3’, R: 5’-TCCGCCTCGTAGTAGAAGTTG-3’.
RNA isolation and sequencing
Request a detailed protocolTotal RNA from purified CB CD34+ cells exposed to Mtb in vitro was isolated using TRIzol LS (Invitrogen; 10296010). RNA-seq libraries were prepared using the Nugen Ovation Trio low input RNA Library Systems V2 (Nugen; 0507–08) according to the manufacturer’s instructions by the Nucleomics Platform (VIB, Leuven, Belgium). Pooled libraries were sequenced as 150 bp, paired-end reads on an Illumina HiSeq 2500 using v4 chemistry.
RNA-seq data quality assessment and differential expression analyses
Request a detailed protocolIllumina sequencing adapters and reads with Phred quality scores lower than 20 were removed with Trimmomatic (0.36). Trimmed reads were aligned to H. sapiens reference genome (hg38) by STAR (2.6.0 c). Aligned reads were mapped to genes using feature Counts from the Subread package (1.6.1). Genes with reads of less than three were removed. Library based normalization was used to transform raw counts to RPKM and further normalized using the edgeR TMM normalization (3.10.0). Data were then transformed using the limma voom function (3.36.2), prior to batch correction using ComBat (sva 3.28.0). Negative binomial and linear model-based methods were used for differential expression analysis, using packages edgeR and limma packages. Differentially expressed genes (DEGs) were calculated with t-statistics, moderated F-statistic, and log-odds of differential expression by empirical Bayes moderation of the standard errors (Supplementary file 4).
CellNet and CellRouter analysis
Request a detailed protocolWe applied CellNet to classify RNA-seq samples as previously described (Cahan et al., 2014). Raw RNA sequencing data files were used for CellNet analysis. We used R version 3.4.1, CellNet version 0.0.0.9000, Salmon (Patro et al., 2017) version 0.8.2 and the corresponding index downloaded from the CellNet website. We used CellRouter (Lummertz da Rocha et al., 2018) to calculate signature scores for each sample based on cell-type specific transcriptional factors collected from literature. Specifically, for this analysis, we normalized raw counts by library size as implemented in the R package DESeq2 (Love et al., 2014). We then plotted the distributions of signature scores across experimental conditions. Moreover, we used CellRouter to identify genes preferentially expressed in each experimental condition and used those genes for Reactome pathways enrichment analysis using the Enrichr package version 1.0.
Systems biology analysis
Request a detailed protocolIngenuity Pathway Analysis (IPA) software was used to perform the initial pathway/function level analysis on genes determined to be differentially expressed in transcriptomic analysis (Ingenuity Systems, Red Wood City, CA). Uncorrected p-values and absolute fold-changes were used with cut-offs of p<0.05 (monocyte transcriptomes from active TB patients) or p<0.01 (differentially expressed genes in Mtb-exposed CD34+ cells and all publicly available datasets from GEO). Differentially expressed genes were sorted into gene networks and canonical pathways, and significantly overrepresented pathways and upstream regulators were identified. Additional pathway, GO (Gene Ontology) and transcription factor target enrichment analysis was performed using GSEA (Gene Set Enrichment Analysis, Broad Institute Molecular Signatures Database (MSigDB)) and WebGestalt (WEB-based GEne SeT AnaLysis Toolkit). Gene sets from GO, Hallmark, KEGG pathways, WikiPathways and Pathway Commons databases, as well as transcription factor motifs, were considered overrepresented if their FDR-corrected p-value was <0.05. To validate our compiled IL6/IL6R/CEBP and CD34+ myeloid differentiation gene modules, we used STRING (version 10.5) protein-protein interaction enrichment analysis (www.string-db.org), using the whole human genome as background. Principal component analysis, correlation matrices, unsupervised hierarchical (Eucledian distance) clustering were performed using XLSTAT and visualized using MORPHEUS (https://software.broadinstitute.org/morpheus/). Chipseq, active chromatin and transcriptional (RNAseq) data of CD14 and CD34+ cells were downloaded from ENCODE (https://genome.ucsc.edu/ENCODE/) and visualized using the UCSC browser (Haeussler et al., 2019).
Data processing and statistical analyses
Request a detailed protocolData derived from in vitro experiments was processed using GraphPad Prism six software and analyzed using unpaired t test, one-way ANOVA or two-way ANOVA according to the experimental settings. Data from experiments performed in triplicate are expressed as mean ± SEM. Non-parametric tests (Mann-Whitney, Spearman correlation) were used for clinical data (sputum bacillar load, modal X-ray grade, symptom count) and molecular data that were not normally distributed, Pearson correlation was used for molecular data with a normal distribution. A list of the statistics analysis methods used in each figure is available a supplementary file (Supplementary file 4). Statistical significance was expressed as follows: *p≤0.05, **p≤0.01 and ***p≤0.001.
Data availability
Sequencing data have been deposited in GEO under accession code GSE129270.
-
NCBI Gene Expression OmnibusID GSE63548. Expression profiling of lymph nodes in tuberculosis patients reveal inflammatory milieu at site of infection.
-
NCBI Gene Expression OmnibusID GSE19443. Blood Transcriptional Profiles of Active TB (UK Test Set Separated).
-
NCBI Gene Expression OmnibusID GSE19435. Transcriptional profiles in Blood of patients with Tuberculosis - Longitudinal Study.
-
NCBI Gene Expression OmnibusID GSE19439. Blood Transcriptional Profiles in Active and Latent Tuberculosis UK (Training Set).
-
NCBI Gene Expression OmnibusID GSE19444. Blood Transcriptional Profiles of Active and Latent TB (UK Test Set).
References
-
Intranasal Poly-IC treatment exacerbates tuberculosis in mice through the pulmonary recruitment of a pathogen-permissive monocyte/macrophage populationJournal of Clinical Investigation 120:1674–1682.https://doi.org/10.1172/JCI40817
-
TLR9 regulates Th1 responses and cooperates with TLR2 in mediating optimal resistance to Mycobacterium tuberculosisThe Journal of Experimental Medicine 202:1715–1724.https://doi.org/10.1084/jem.20051782
-
Co-evolution of Mycobacterium tuberculosis and Homo sapiensImmunological Reviews 264:6–24.https://doi.org/10.1111/imr.12264
-
Simultaneous measurement of human hematopoietic stem and progenitor cells in blood using multicolor flow cytometryCytometry Part B: Clinical Cytometry 90:415–423.https://doi.org/10.1002/cyto.b.21354
-
CD271+ bone marrow mesenchymal stem cells may provide a niche for dormant Mycobacterium tuberculosisScience Translational Medicine 5:170ra13.https://doi.org/10.1126/scitranslmed.3004912
-
Evidence for polygenic adaptation to pathogens in the human genomeMolecular Biology and Evolution 30:1544–1558.https://doi.org/10.1093/molbev/mst080
-
ISG15-Induced IL-10 is a novel Anti-Inflammatory myeloid Axis disrupted during active tuberculosisThe Journal of Immunology 200:1434–1442.https://doi.org/10.4049/jimmunol.1701120
-
Instruction of hematopoietic lineage choice by cytokine signalingExperimental Cell Research 329:207–213.https://doi.org/10.1016/j.yexcr.2014.07.011
-
Immunophenotypic pattern of myeloid populations by flow cytometry analysisMethods in Cell Biology 103:221–266.https://doi.org/10.1016/B978-0-12-385493-3.00010-3
-
The UCSC genome browser database: 2019 updateNucleic Acids Research 47:D853–D858.https://doi.org/10.1093/nar/gky1095
-
Regulation of expression of the CD11b and CD18 subunits of the neutrophil adherence receptor during human myeloid differentiationThe Journal of Biological Chemistry 264:21812–21817.
-
Role of STAT3 in type I interferon responses. negative regulation of STAT1-dependent inflammatory gene activationThe Journal of Biological Chemistry 281:14111–14118.https://doi.org/10.1074/jbc.M511797200
-
Hematopoietic stem cell niche in health and diseaseAnnual Review of Pathology: Mechanisms of Disease 11:555–581.https://doi.org/10.1146/annurev-pathol-012615-044414
-
Interleukin 6 is a permissive factor for monocytic colony formation by human hematopoietic progenitor cellsJournal of Experimental Medicine 175:1151–1154.https://doi.org/10.1084/jem.175.4.1151
-
Mycobacterial growth inhibition is associated with trained innate immunityJournal of Clinical Investigation 128:1837–1851.https://doi.org/10.1172/JCI97508
-
Immunobiology of visceral leishmaniasisFrontiers in Immunology 3:251.https://doi.org/10.3389/fimmu.2012.00251
-
Restricted dendritic cell and monocyte progenitors in human cord blood and bone marrowThe Journal of Experimental Medicine 212:385–399.https://doi.org/10.1084/jem.20141442
-
The interferon signaling network and transcription factor C/EBP-betaCellular & Molecular Immunology 4:407–418.
-
Infection-induced myelopoiesis during intracellular bacterial infection is critically dependent upon IFN-γ signalingThe Journal of Immunology 186:1032–1043.https://doi.org/10.4049/jimmunol.1001893
-
Evolutionary genetic dissection of human interferonsThe Journal of Experimental Medicine 208:2747–2759.https://doi.org/10.1084/jem.20111680
-
Pro-inflammatory cytokines: emerging players regulating HSC function in normal and diseased hematopoiesisExperimental Cell Research 329:248–254.https://doi.org/10.1016/j.yexcr.2014.08.017
-
Toll-like receptor 1 polymorphisms increased the risk of pulmonary tuberculosis in an iranian population sampleBiomedical and Environmental Sciences : BES 29:825–828.https://doi.org/10.3967/bes2016.110
-
Mycobacterium tuberculosis triggers host type I IFN signaling to regulate IL-1β production in human macrophagesThe Journal of Immunology 187:2540–2547.https://doi.org/10.4049/jimmunol.1100926
-
Anti-IL-6 receptor antibody causes less promotion of tuberculosis infection than Anti-TNF- Antibody in miceClinical and Developmental Immunology 2011:1–9.https://doi.org/10.1155/2011/404929
-
Detection of nonneutral substitution rates on mammalian phylogeniesGenome Research 20:110–121.https://doi.org/10.1101/gr.097857.109
-
CCAAT/enhancer-binding proteins: structure, function and regulationBiochemical Journal 365:561–575.https://doi.org/10.1042/bj20020508
-
Detection of interferon alpha protein reveals differential levels and cellular sources in diseaseThe Journal of Experimental Medicine 214:1547–1555.https://doi.org/10.1084/jem.20161451
-
A study of the blood monocytes in children with tuberculosisNew England Journal of Medicine 198:740–749.https://doi.org/10.1056/NEJM192805241981410
-
Monocyte recruitment in tuberculosis and sarcoidosisBritish Journal of Haematology 35:11–17.https://doi.org/10.1111/j.1365-2141.1977.tb00557.x
-
Treatment of multiple sclerosis patients with interferon-beta primes monocyte-derived macrophages for apoptotic cell deathJournal of Leukocyte Biology 70:745–748.
-
Ratio of monocytes to lymphocytes in peripheral blood in patients diagnosed with active tuberculosisThe Brazilian Journal of Infectious Diseases 19:125–131.https://doi.org/10.1016/j.bjid.2014.10.008
-
A functional single-nucleotide polymorphism in the promoter of the gene encoding interleukin 6 is associated with susceptibility to tuberculosisThe Journal of Infectious Diseases 205:1697–1704.https://doi.org/10.1093/infdis/jis266
-
Cytokines regulating hematopoietic stem cell functionCurrent Opinion in Hematology 15:307–311.https://doi.org/10.1097/MOH.0b013e3283007db5
Article and author information
Author details
Funding
Howard Hughes Medical Institute (Early Career Scientist 55007412)
- André Báfica
Coordenação de Aperfeiçoamento de Pessoal de Nível Superior (23038.010048/2013-27)
- Daniel S Mansur
Fonds Wetenschappelijk Onderzoek (G0D6817N)
- Johan Van Weyenbergh
National Institutes of Health (Global Research Initiative Program TW008276)
- André Báfica
Conselho Nacional de Desenvolvimento Científico e Tecnológico (PQ)
- André Báfica
- Daniel S Mansur
Fonds Wetenschappelijk Onderzoek (VLAIO IWT141614)
- Tim Dierckx
The funders had no role in study design, data collection and interpretation, or the decision to submit the work for publication.
Acknowledgements
We thank Drs. José Henrique M Oliveira/UFSC and João T Marques/UFMG for their critical reading of this manuscript and UFSC microscopy (LCME) and biology (LAMEB) facilities for technical support. This work was funded by Howard Hughes Medical Institute – Early Career Scientist (AB; 55007412), National Institutes of Health Global Research Initiative Program (AB, TW008276), Coordenação de Aperfeiçoamento de Pessoal de Nível Superior (CAPES) Computational Biology (DSM; 23038.010048/2013–27), FWO (JVW; G0D6817N), FWO (TD; VLAIO IWT141614) and CNPQ/PQ Scholars (AB and DSM).
Ethics
Human subjects: This study was approved by the institutional review boards of Universidade Federal de Santa Catarina and The University Hospital Prof. Polydoro Ernani de São Thiago (IRB# 89894417.8.0000.0121). Informed consent was obtained from all subjects.
Copyright
© 2019, Delgobo et al.
This article is distributed under the terms of the Creative Commons Attribution License, which permits unrestricted use and redistribution provided that the original author and source are credited.
Metrics
-
- 4,197
- views
-
- 468
- downloads
-
- 30
- citations
Views, downloads and citations are aggregated across all versions of this paper published by eLife.
Download links
Downloads (link to download the article as PDF)
Open citations (links to open the citations from this article in various online reference manager services)
Cite this article (links to download the citations from this article in formats compatible with various reference manager tools)
Further reading
-
- Computational and Systems Biology
- Genetics and Genomics
Obesity is a major risk factor for type 2 diabetes, dyslipidemia, cardiovascular disease, and hypertension. Intriguingly, there is a subset of metabolically healthy obese (MHO) individuals who are seemingly able to maintain a healthy metabolic profile free of metabolic syndrome. The molecular underpinnings of MHO, however, are not well understood. Here, we report that CTRP10/C1QL2-deficient mice represent a unique female model of MHO. CTRP10 modulates weight gain in a striking and sexually dimorphic manner. Female, but not male, mice lacking CTRP10 develop obesity with age on a low-fat diet while maintaining an otherwise healthy metabolic profile. When fed an obesogenic diet, female Ctrp10 knockout (KO) mice show rapid weight gain. Despite pronounced obesity, Ctrp10 KO female mice do not develop steatosis, dyslipidemia, glucose intolerance, insulin resistance, oxidative stress, or low-grade inflammation. Obesity is largely uncoupled from metabolic dysregulation in female KO mice. Multi-tissue transcriptomic analyses highlighted gene expression changes and pathways associated with insulin-sensitive obesity. Transcriptional correlation of the differentially expressed gene (DEG) orthologs in humans also shows sex differences in gene connectivity within and across metabolic tissues, underscoring the conserved sex-dependent function of CTRP10. Collectively, our findings suggest that CTRP10 negatively regulates body weight in females, and that loss of CTRP10 results in benign obesity with largely preserved insulin sensitivity and metabolic health. This female MHO mouse model is valuable for understanding sex-biased mechanisms that uncouple obesity from metabolic dysfunction.
-
- Computational and Systems Biology
Mass spectrometry imaging (MSI) is a powerful technology used to define the spatial distribution and relative abundance of metabolites across tissue cryosections. While software packages exist for pixel-by-pixel individual metabolite and limited target pairs of ratio imaging, the research community lacks an easy computing and application tool that images any metabolite abundance ratio pairs. Importantly, recognition of correlated metabolite pairs may contribute to the discovery of unanticipated molecules in shared metabolic pathways. Here, we describe the development and implementation of an untargeted R package workflow for pixel-by-pixel ratio imaging of all metabolites detected in an MSI experiment. Considering untargeted MSI studies of murine brain and embryogenesis, we demonstrate that ratio imaging minimizes systematic data variation introduced by sample handling, markedly enhances spatial image contrast, and reveals previously unrecognized metabotype-distinct tissue regions. Furthermore, ratio imaging facilitates identification of novel regional biomarkers and provides anatomical information regarding spatial distribution of metabolite-linked biochemical pathways. The algorithm described herein is applicable to any MSI dataset containing spatial information for metabolites, peptides or proteins, offering a potent hypothesis generation tool to enhance knowledge obtained from current spatial metabolite profiling technologies.